Blind Image Super-resolution Reconstruction via Iterative and Alternative Optimization
Journal of Electronics & Information Technology(2022)
摘要
Deep convolutional neural network-based image Super-Resolution (SR) methods generally assume that the degradations of Low-Resolution (LR) images are fixed and known (e.g., bicubic downsampling). Thus, they are almost unable to super-resolve images with unknown degradations (e.g., blur kernel and noise level). To address this problem, an iterative and alternative optimization-based blind image SR network is proposed, in which the blur kernel, noise level, and High-Resolution (HR) image are jointly estimated. Specifically, in the proposed method, the image reconstruction network reconstructs an HR image from the given LR image using the estimated blur kernel and noise level as prior knowledge. Correspondingly, the blur kernel and noise level estimators estimate the blur kernel and noise level respectively from the given LR image and the reconstructed HR image. To improve compatibility and promote each other mutually, the blur kernel estimator, noise level estimator, and image reconstruction network are iteratively and alternatively optimized in an end-to-end manner. The proposed network is compared with state-of-the-art methods (i.e., IKC, DASR, MANet, DAN) on commonly used benchmarks (i.e., Set5, Set14, B100, and Urban100) and real-world images. Results show that the proposed method achieves better performance on LR images with unknown degradations. Moreover, the proposed method has advantages in model size or processing efficiency.
更多查看译文
AI 理解论文
溯源树
样例
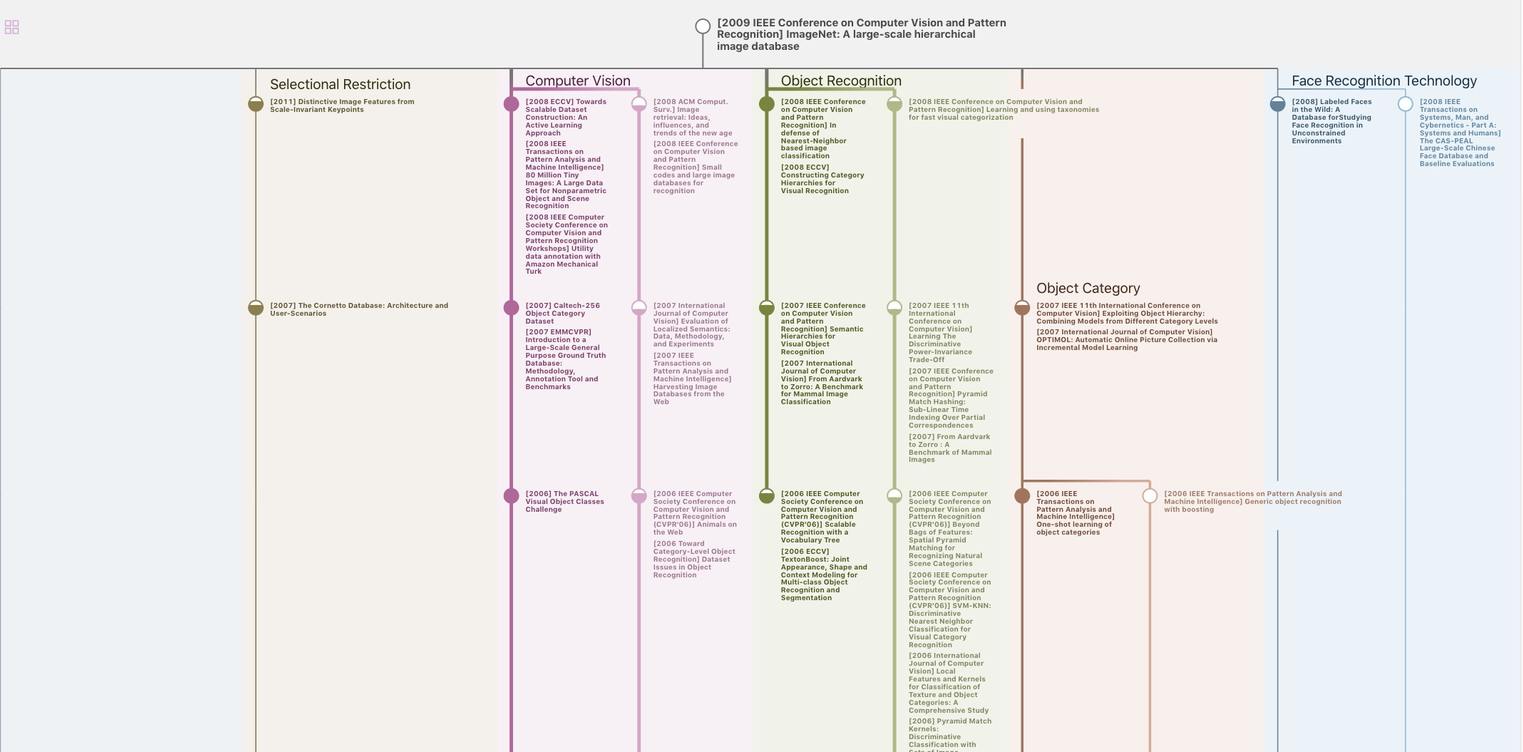
生成溯源树,研究论文发展脉络
Chat Paper
正在生成论文摘要