Multi-Objective Reinforcement Learning Based Healthcare Expansion Planning Considering Pandemic Events
IEEE journal of biomedical and health informatics(2023)
摘要
Hospital capacity expansion planning is critical for a healthcare authority, especially in regions with a growing diverse population. Policymaking to this end often requires satisfying two conflicting objectives, minimizing capacity expansion cost and minimizing the number of denial of service (DoS) for patients seeking hospital admission. The uncertainty in hospital demand, especially considering a pandemic event, makes expansion planning even more challenging. This work presents a multi-objective reinforcement learning (MORL) based solution for healthcare expansion planning to optimize expansion cost and DoS simultaneously for pandemic and non-pandemic scenarios. Importantly, our model provides a simple and intuitive way to set the balance between these two objectives by only determining their priority percentages, making it suitable across policymakers with different capabilities, preferences, and needs. Specifically, we propose a multi-objective adaptation of the popular Advantage Actor-Critic (A2C) algorithm to avoid forced conversion of DoS discomfort cost to a monetary cost. Our case study for the state of Florida illustrates the success of our MORL based approach compared to the existing benchmark policies, including a state-of-the-art deep RL policy that converts DoS to economic cost to optimize a single objective.
更多查看译文
关键词
Hospitals,Costs,Forecasting,Planning,Statistics,Sociology,Pandemics,Deep RL,denial of service,healthcare capacity expansion,Markov decision process,multi-objective optimization
AI 理解论文
溯源树
样例
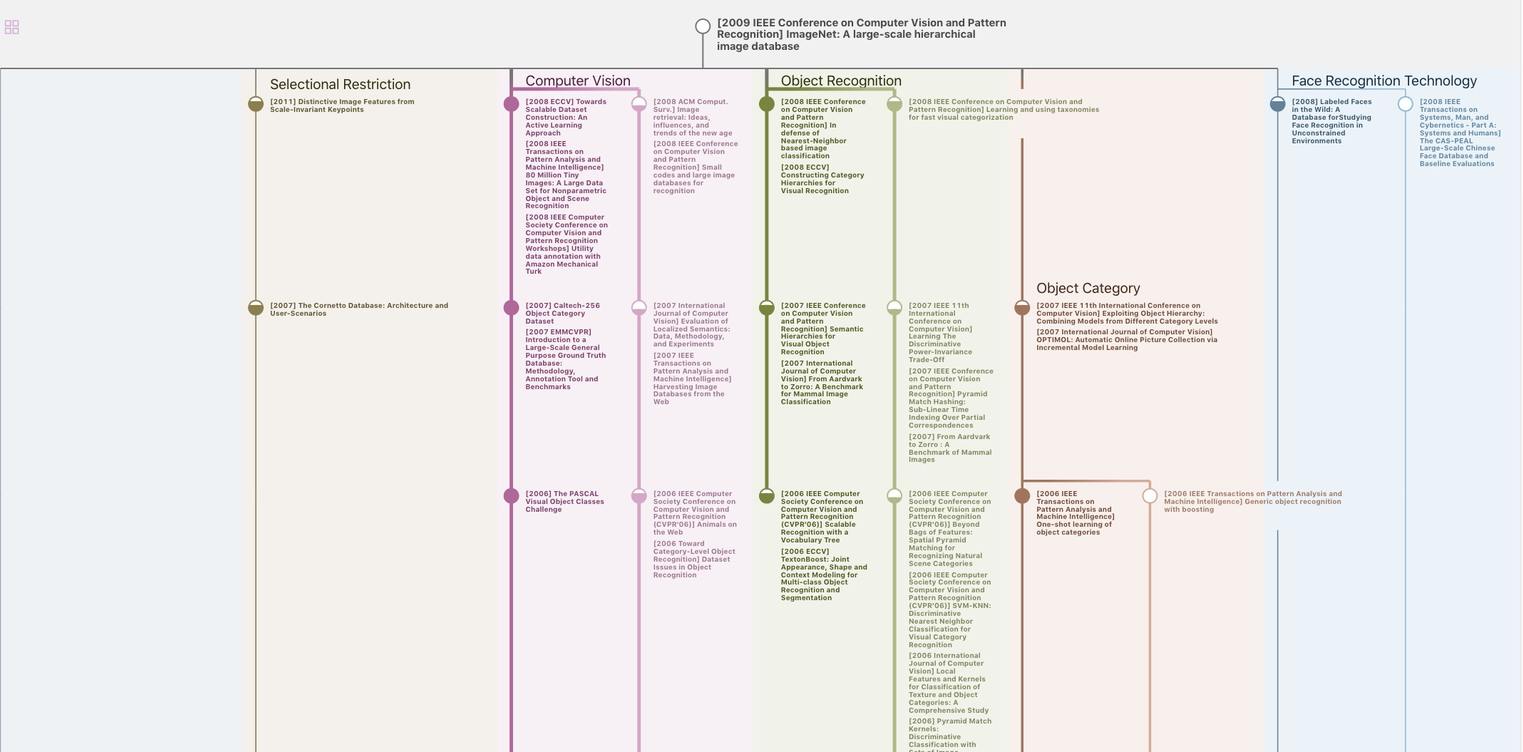
生成溯源树,研究论文发展脉络
Chat Paper
正在生成论文摘要