Feature Extraction and Classification from Planetary Science Datasets enabled by Machine Learning
2023 IEEE AEROSPACE CONFERENCE(2023)
摘要
In this paper we present two examples of recent investigations that we have undertaken, applying Machine Learning (ML) neural networks (NN) to image datasets from outer planet missions to achieve feature recognition. Our first investigation was to recognize ice blocks (also known as rafts, plates, polygons) in the chaos regions of fractured ice on Europa. We used a transfer learning approach, adding and training new layers to an industry-standard Mask R-CNN (Region-based Convolutional Neural Network) to recognize labeled blocks in a training dataset. Subsequently, the updated model was tested against a new dataset, achieving 68% precision. In a different application, we applied the Mask R-CNN to recognize clouds on Titan, again through updated training followed by testing against new data, with a precision of 95% over 369 images. We evaluate the relative successes of our techniques and suggest how training and recognition could be further improved. The new approaches we have used for planetary datasets can further be applied to similar recognition tasks on other planets, including Earth. For imagery of outer planets in particular, the technique holds the possibility of greatly reducing the volume of returned data, via onboard identification of the most interesting image subsets, or by returning only differential data (images where changes have occurred) greatly enhancing the information content of the final data stream.
更多查看译文
关键词
chaos regions,differential data,feature extraction,feature recognition,fractured ice,ice blocks,image datasets,industry-standard Mask R-CNN,interesting image subsets,labeled blocks,Machine Learning,NN,outer planet missions,outer planets,planetary datasets,planetary science datasets,plates,polygons,rafts,Region-based Convolutional Neural Network,relative successes,returned data,similar recognition tasks,training dataset,transfer learning approach,updated model,updated training
AI 理解论文
溯源树
样例
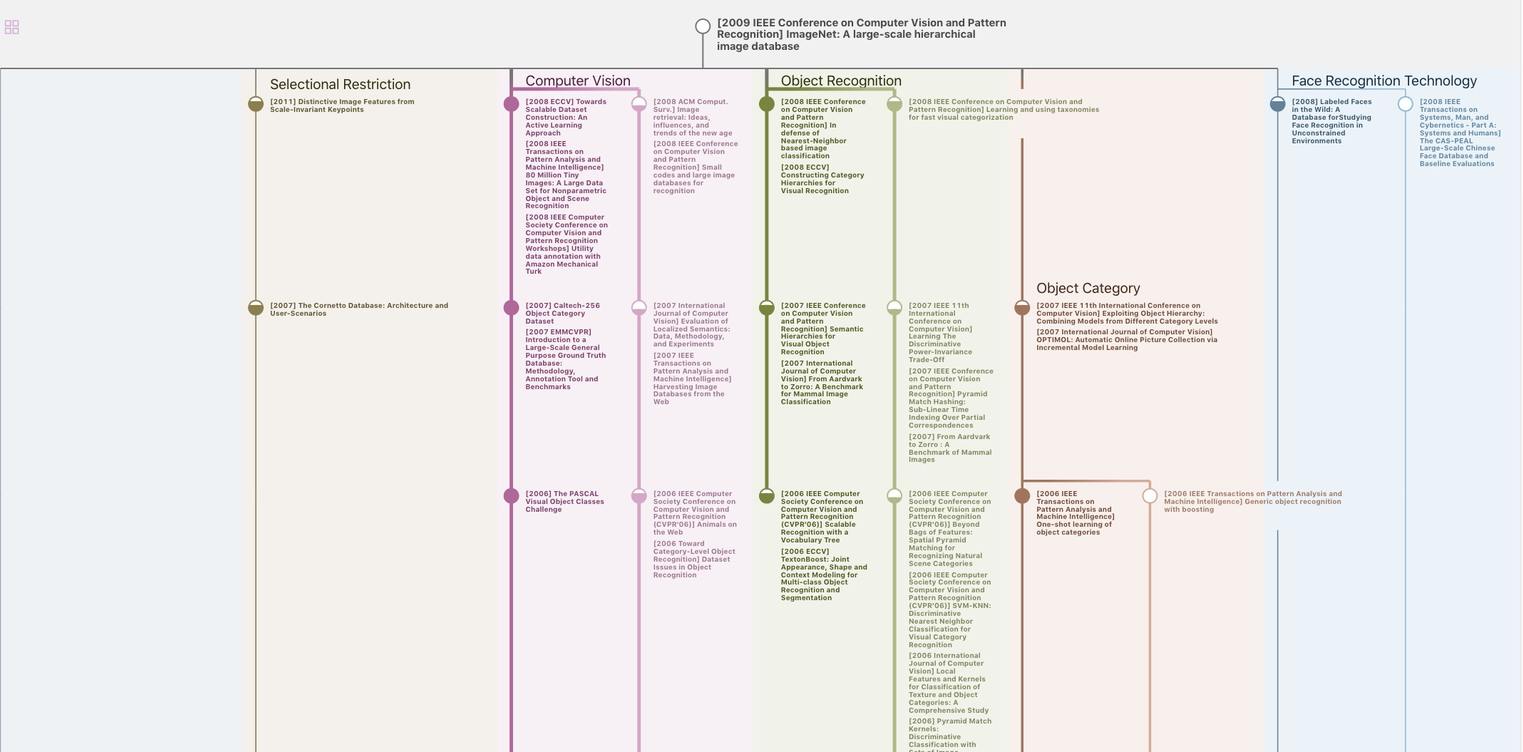
生成溯源树,研究论文发展脉络
Chat Paper
正在生成论文摘要