Determining the Set of Items to Include in Breast Operative Reports, Using Clustering Algorithms on Retrospective Data Extracted from Clinical DataWarehouse.
International Conference on Informatics, Management and Technology in Healthcare (ICIMTH)(2022)
摘要
Medical reports are key elements to guarantee the quality, and continuity of care but their quality remains an issue. Standardization and structuration of reports can increase their quality, but are usually based on expert opinions. Here, we hypothesize that a structured model of medical reports could be learnt using machine learning on retrospective medical reports extracted from clinical data warehouses (CDW). To investigate our hypothesis, we extracted breast cancer operative reports from our CDW. Each document was preprocessed and split into sentences. Clustering was performed using TFIDF, Paraphrase or Universal Sentence Encoder along with K-Means, DBSCAN, or Hierarchical clustering. The best couple was TFIDF/K-Means, providing a sentence coverage of 89 % on our dataset; and allowing to identify 7 main categories of items to include in breast cancer operative reports. These results are encouraging for a document preset creation task and should then be validated and implemented in real life.
更多查看译文
关键词
Breast cancer,Clustering,Machine learning,NLP
AI 理解论文
溯源树
样例
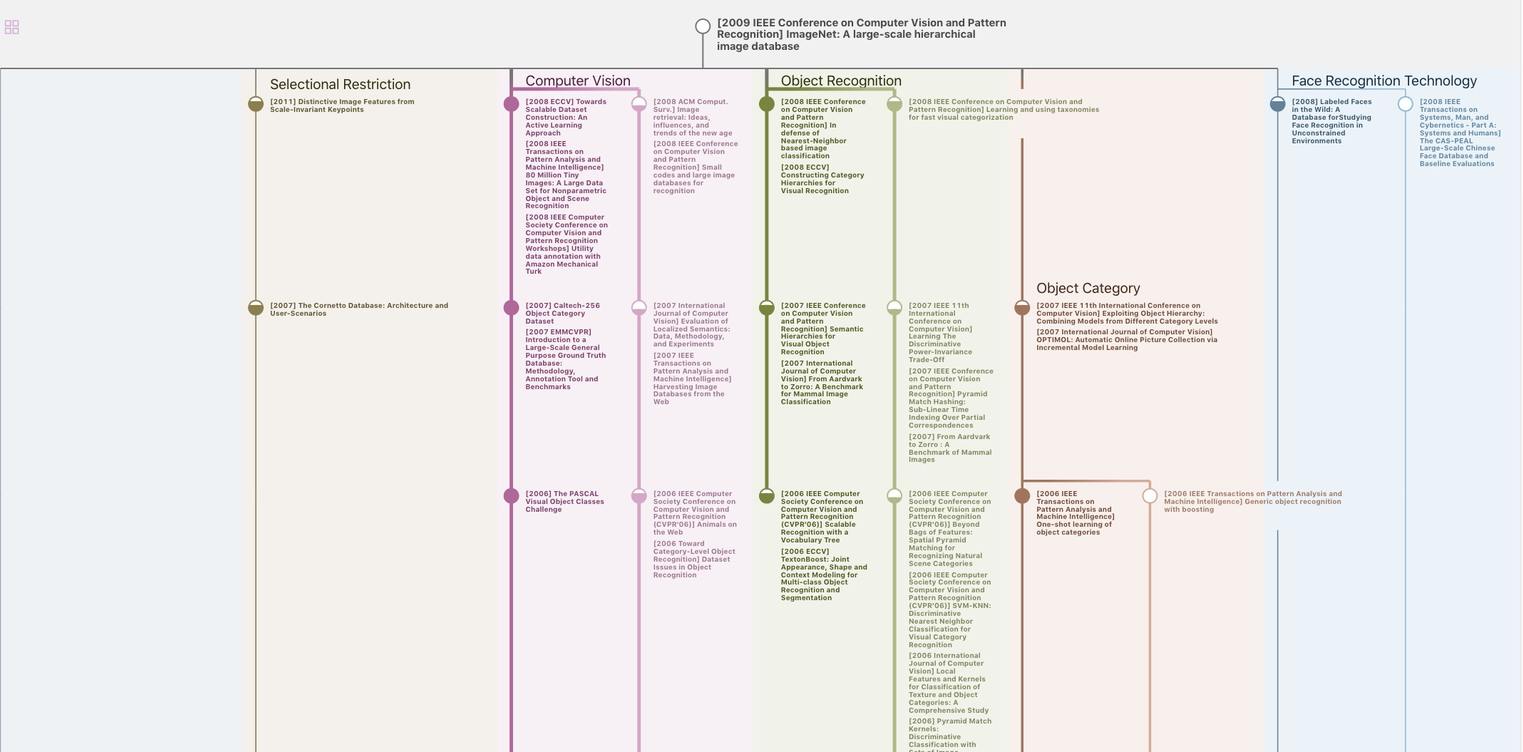
生成溯源树,研究论文发展脉络
Chat Paper
正在生成论文摘要