Targeted learning in observational studies with multi-level treatments: An evaluation of antipsychotic drug treatment safety
arxiv(2022)
摘要
Antipsychotic drugs are widely used to treat serious mental illnesses, but concerns remain about their metabolic risks. Randomized trials have focused on the risk factors for diabetes rather than diabetes itself; observational studies have examined diabetes, but have pooled antipsychotics to compare classes or compared specific drugs to no drug. We estimate the causal effects of six antipsychotics in the absence of randomization within a cohort of nearly 39,000 adults with serious mental illness. Doubly-robust estimators, such as targeted minimum loss-based estimation (TMLE), require correct specification of either the treatment model or outcome model to ensure consistent estimation; however, common TMLE implementations estimate treatment probabilities using multiple binomial regressions rather than multinomial regression. We implement a TMLE estimator that uses multinomial treatment assignment and ensemble machine learning to estimate average treatment effects. Our implementation achieves superior coverage probability relative to the binomial implementation in simulation experiments with varying treatment propensity overlap and event rates. We evaluate the causal effects of the antipsychotics on 3-year diabetes risk or death. We find a safety benefit of moving from a second-generation drug considered among the safest of the second-generation drugs to an infrequently prescribed first-generation drug thought to pose a generally low cardiometabolic risk.
更多查看译文
AI 理解论文
溯源树
样例
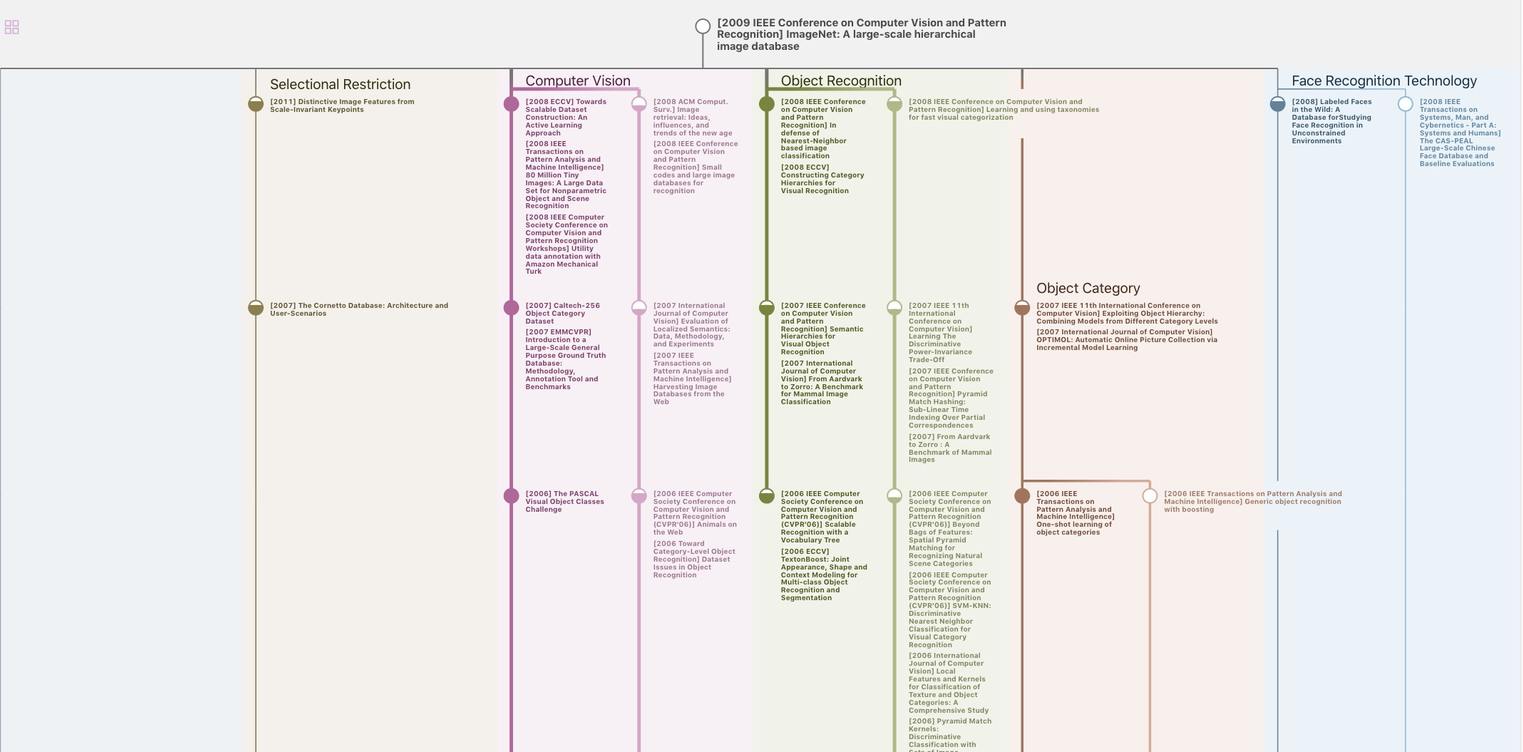
生成溯源树,研究论文发展脉络
Chat Paper
正在生成论文摘要