Multiclass-SGCN: Sparse Graph-based Trajectory Prediction with Agent Class Embedding
arxiv(2022)
摘要
Trajectory prediction of road users in real-world scenarios is challenging because their movement patterns are stochastic and complex. Previous pedestrian-oriented works have been successful in modelling the complex interactions among pedestrians, but fail in predicting trajectories when other types of road users are involved (e.g., cars, cyclists, etc.), because they ignore user types. Although a few recent works construct densely connected graphs with user label information, they suffer from superfluous spatial interactions and temporal dependencies. To address these issues, we propose Multiclass-SGCN, a sparse graph convolution network based approach for multi-class trajectory prediction that takes into consideration velocity and agent label information and uses a novel interaction mask to adaptively decide the spatial and temporal connections of agents based on their interaction scores. The proposed approach significantly outperformed state-of-the-art approaches on the Stanford Drone Dataset, providing more realistic and plausible trajectory predictions.
更多查看译文
关键词
trajectory prediction, multi-class agents, graph convolution networks, self-attention
AI 理解论文
溯源树
样例
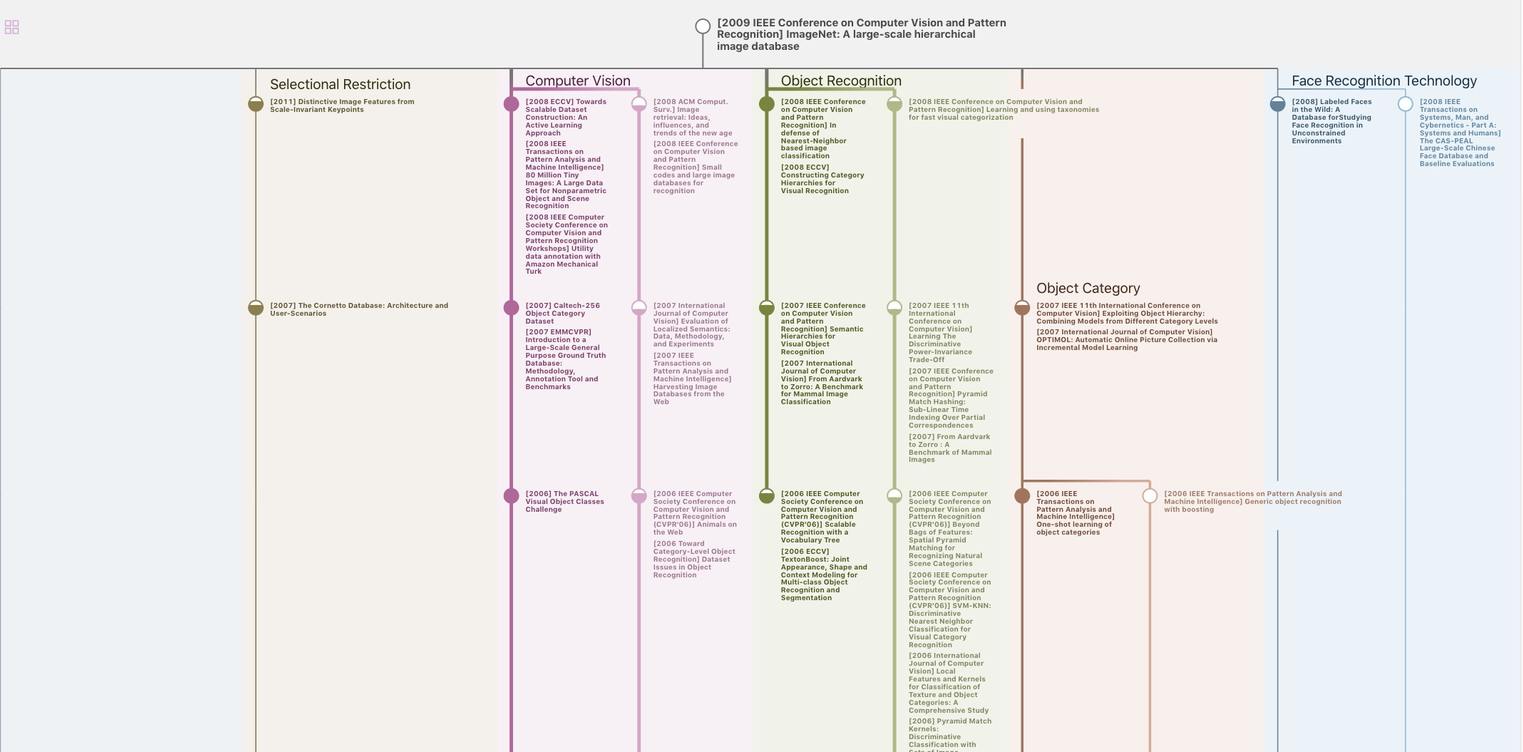
生成溯源树,研究论文发展脉络
Chat Paper
正在生成论文摘要