Imputation under Differential Privacy
arxiv(2022)
摘要
The literature on differential privacy almost invariably assumes that the data to be analyzed are fully observed. In most practical applications this is an unrealistic assumption. A popular strategy to address this problem is imputation, in which missing values are replaced by estimated values given the observed data. In this paper we evaluate various approaches to answering queries on an imputed dataset in a differentially private manner, as well as discuss trade-offs as to where along the pipeline privacy is considered. We show that if imputation is done without consideration to privacy, the sensitivity of certain queries can increase linearly with the number of incomplete records. On the other hand, for a general class of imputation strategies, these worst case scenarios can be greatly reduced by ensuring privacy already during the imputation stage. We use a simulated dataset to demonstrate these results across a number of imputation schemes (both private and non-private) and examine their impact on the utility of a private query on the data.
更多查看译文
关键词
differential privacy
AI 理解论文
溯源树
样例
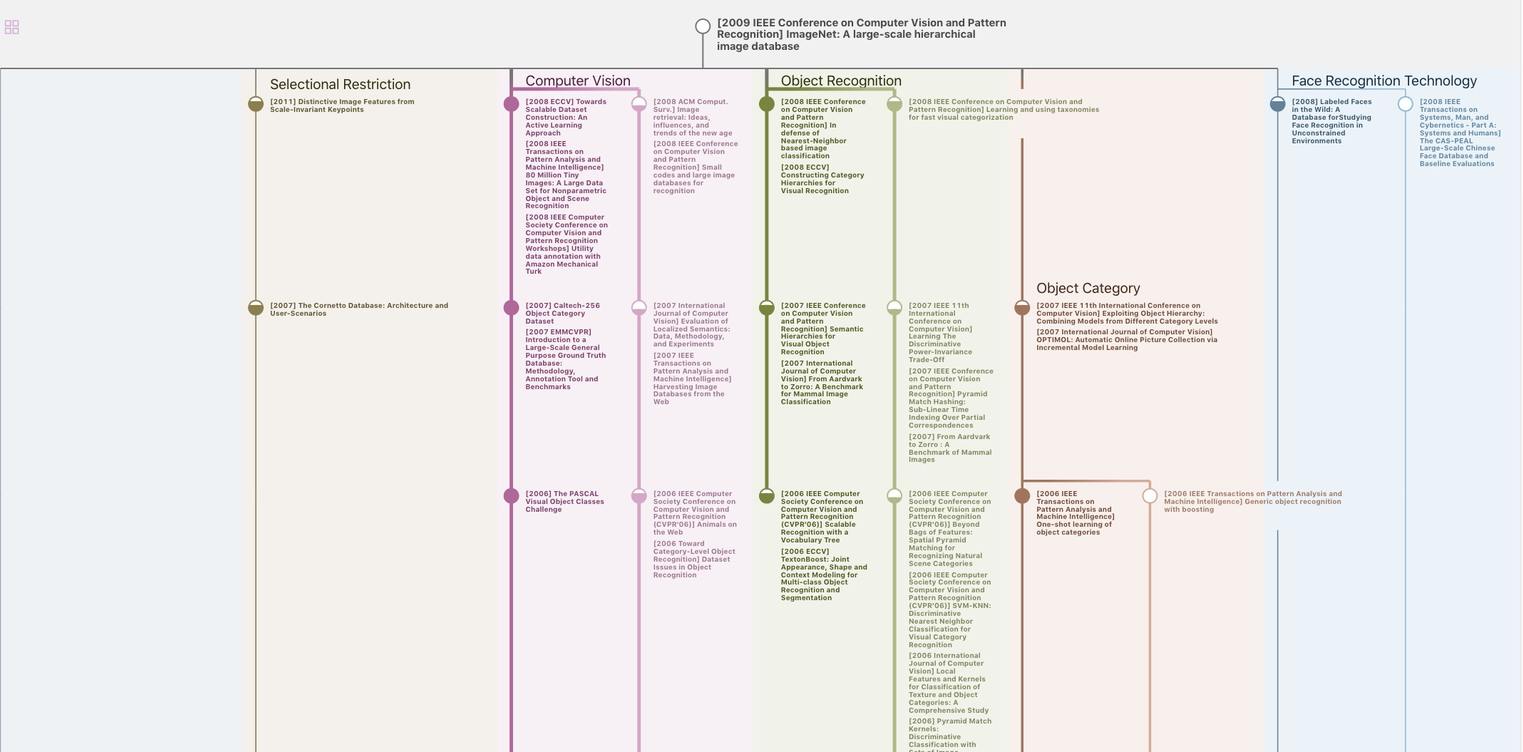
生成溯源树,研究论文发展脉络
Chat Paper
正在生成论文摘要