On the Convergence of Distributed Stochastic Bilevel Optimization Algorithms over a Network
arxiv(2023)
摘要
Bilevel optimization has been applied to a wide variety of machine learning models, and numerous stochastic bilevel optimization algorithms have been developed in recent years. However, most existing algorithms restrict their focus on the single-machine setting so that they are incapable of handling the distributed data. To address this issue, under the setting where all participants compose a network and perform peer-to-peer communication in this network, we developed two novel decentralized stochastic bilevel optimization algorithms based on the gradient tracking communication mechanism and two different gradient estimators. Additionally, we established their convergence rates for nonconvex-strongly-convex problems with novel theoretical analysis strategies. To our knowledge, this is the first work achieving these theoretical results. Finally, we applied our algorithms to practical machine learning models, and the experimental results confirmed the efficacy of our algorithms.
更多查看译文
关键词
stochastic bilevel optimization,algorithms,network
AI 理解论文
溯源树
样例
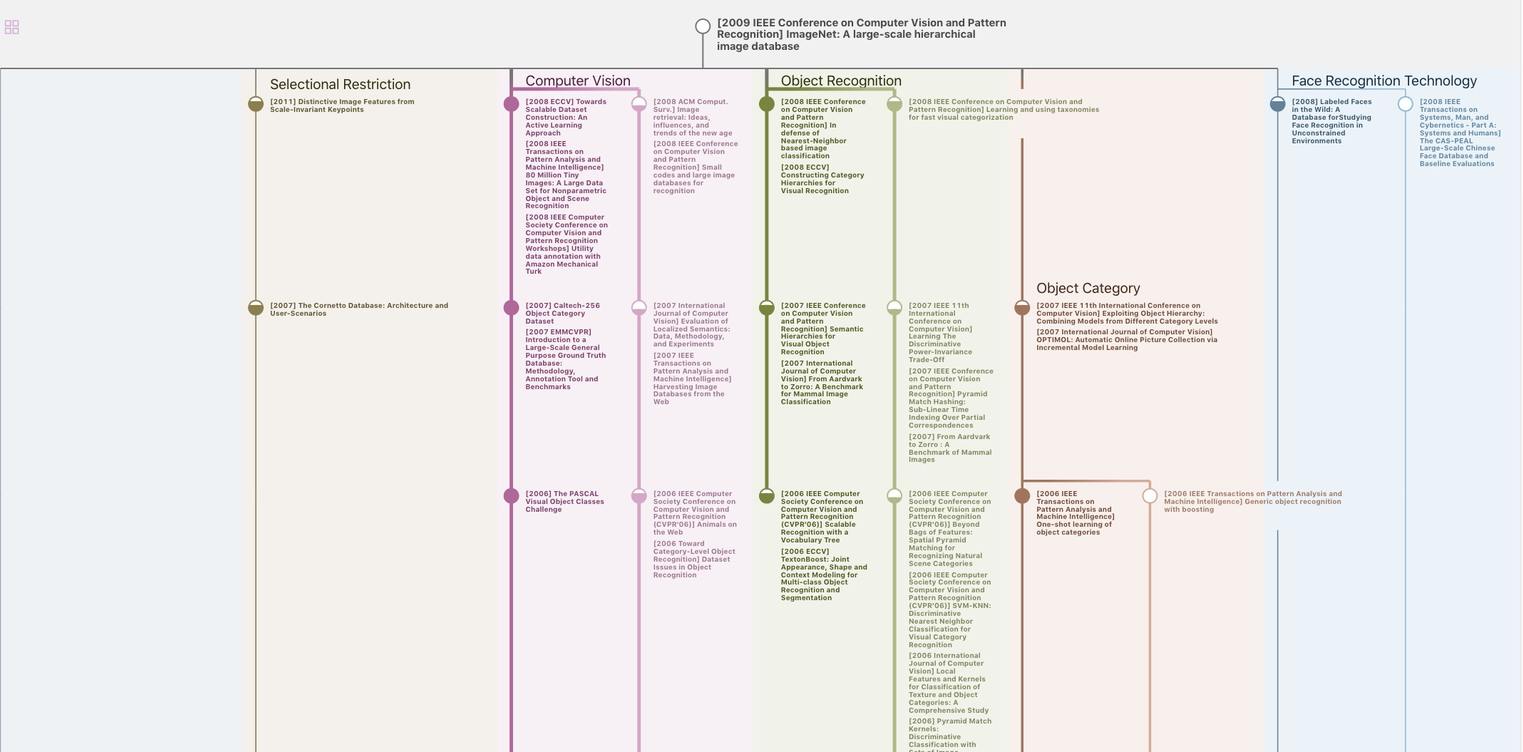
生成溯源树,研究论文发展脉络
Chat Paper
正在生成论文摘要