Benchmarking the Robustness of Deep Neural Networks to Common Corruptions in Digital Pathology
MEDICAL IMAGE COMPUTING AND COMPUTER ASSISTED INTERVENTION, MICCAI 2022, PT II(2022)
摘要
When designing a diagnostic model for a clinical application, it is crucial to guarantee the robustness of the model with respect to a wide range of image corruptions. Herein, an easy-to-use benchmark is established to evaluate how deep neural networks perform on corrupted pathology images. Specifically, corrupted images are generated by injecting nine types of common corruptions into validation images. Besides, two classification and one ranking metrics are designed to evaluate the prediction and confidence performance under corruption. Evaluated on two resulting benchmark datasets, we find that (1) a variety of deep neural network models suffer from a significant accuracy decrease (double the error on clean images) and the unreliable confidence estimation on corrupted images; (2) A low correlation between the validation and test errors while replacing the validation set with our benchmark can increase the correlation. Our codes are available on https://github.com/superjamessyx/robustness_benchmark.
更多查看译文
关键词
Robustness, Digital pathology, Benchmark, Corruption
AI 理解论文
溯源树
样例
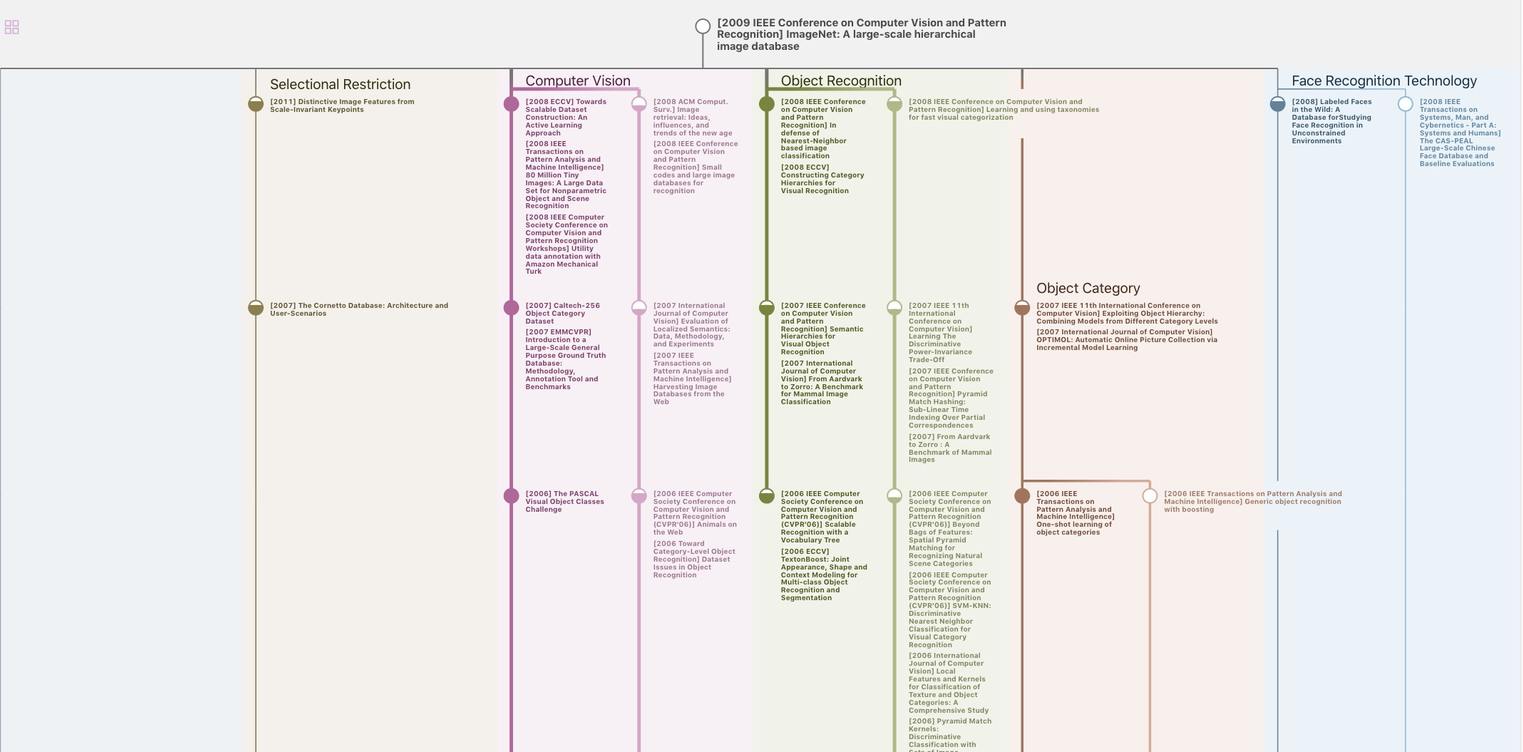
生成溯源树,研究论文发展脉络
Chat Paper
正在生成论文摘要