Cut Inner Layers: A Structured Pruning Strategy for Efficient U-Net GANs
arxiv(2022)
摘要
Pruning effectively compresses overparameterized models. Despite the success of pruning methods for discriminative models, applying them for generative models has been relatively rarely approached. This study conducts structured pruning on U-Net generators of conditional GANs. A per-layer sensitivity analysis confirms that many unnecessary filters exist in the innermost layers near the bottleneck and can be substantially pruned. Based on this observation, we prune these filters from multiple inner layers or suggest alternative architectures by completely eliminating the layers. We evaluate our approach with Pix2Pix for image-to-image translation and Wav2Lip for speech-driven talking face generation. Our method outperforms global pruning baselines, demonstrating the importance of properly considering where to prune for U-Net generators.
更多查看译文
关键词
structured pruning strategy,inner layers,cut,u-net
AI 理解论文
溯源树
样例
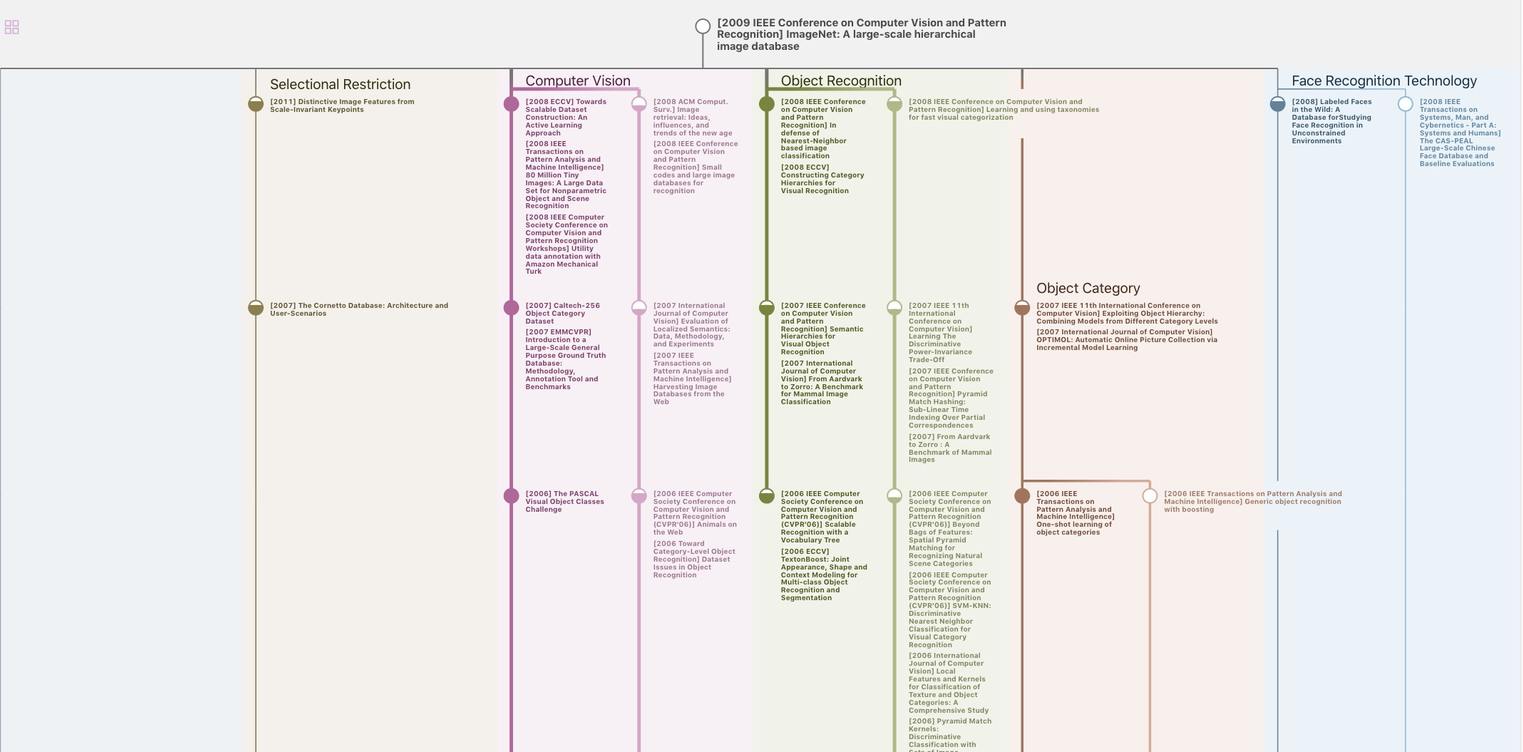
生成溯源树,研究论文发展脉络
Chat Paper
正在生成论文摘要