Bayesian Multi-Task Variable Selection with an Application to Differential DAG Analysis
JOURNAL OF COMPUTATIONAL AND GRAPHICAL STATISTICS(2024)
摘要
We study the Bayesian multi-task variable selection problem, where the goal is to select activated variables for multiple related datasets simultaneously. We propose a new variational Bayes algorithm which generalizes and improves the recently developed "sum of single effects" model of Wang et al. Motivated by differential gene network analysis in biology, we further extend our method to joint structure learning of multiple directed acyclic graphical models, a problem known to be computationally highly challenging. We propose a novel order MCMC sampler where our multi-task variable selection algorithm is used to quickly evaluate the posterior probability of each ordering. Both simulation studies and real gene expression data analysis are conducted to show the efficiency of our method. Finally, we also prove a posterior consistency result for multi-task variable selection, which provides a theoretical guarantee for the proposed algorithms. Supplementary materials for this article are available online.
更多查看译文
关键词
Joint DAG estimation,Spike-and-slab prior,Strong selection consistency,SuSiE model,Variational inference
AI 理解论文
溯源树
样例
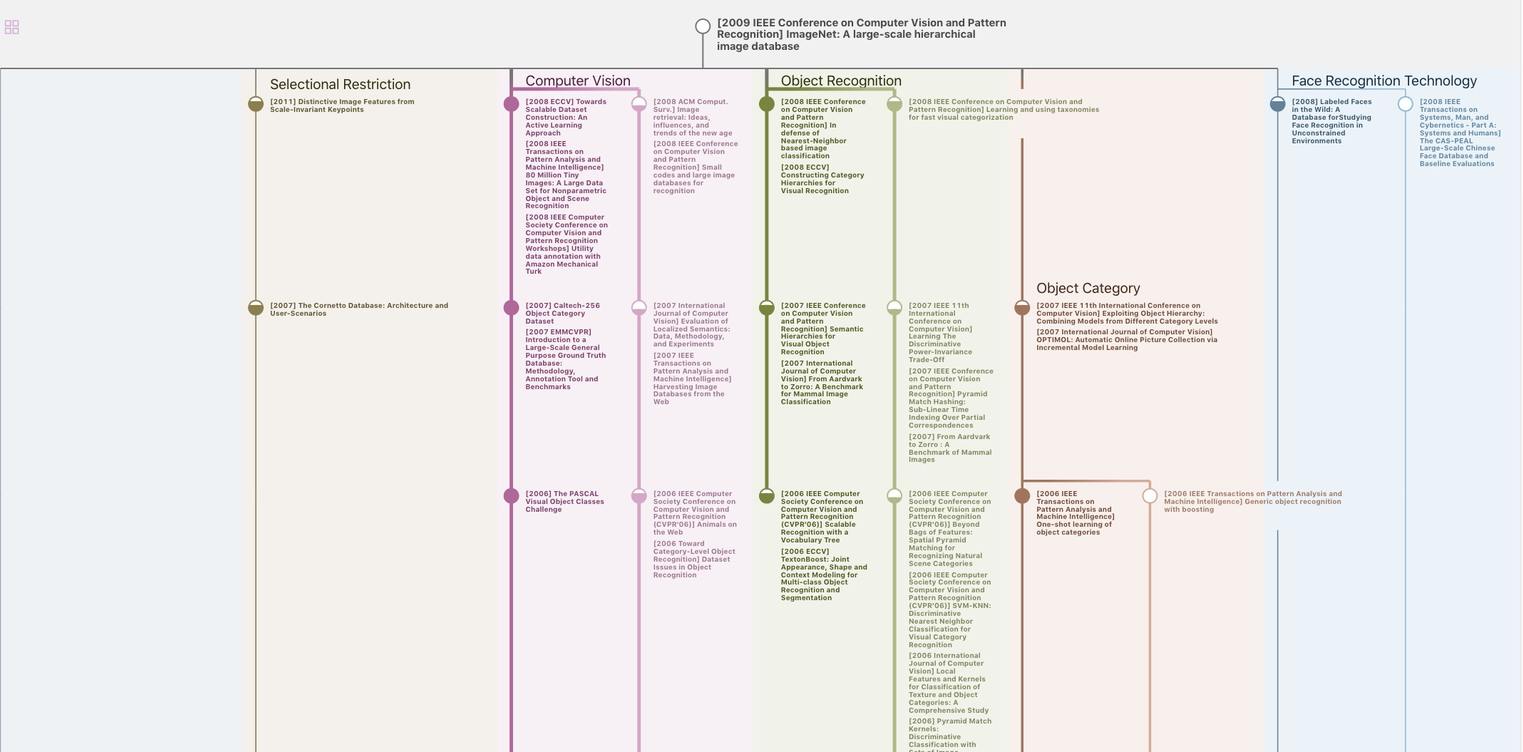
生成溯源树,研究论文发展脉络
Chat Paper
正在生成论文摘要