Hyperspectral image reconstruction for spectral camera based on ghost imaging via sparsity constraints using V-DUnet
arxiv(2022)
摘要
Spectral camera based on ghost imaging via sparsity constraints (GISC spectral camera) obtains three-dimensional (3D) hyperspectral information with two-dimensional (2D) compressive measurements in a single shot, which has attracted much attention in recent years. However, its imaging quality and real-time performance of reconstruction still need to be further improved. Recently, deep learning has shown great potential in improving the reconstruction quality and reconstruction speed for computational imaging. When applying deep learning into GISC spectral camera, there are several challenges need to be solved: 1) how to deal with the large amount of 3D hyperspectral data, 2) how to reduce the influence caused by the uncertainty of the random reference measurements, 3) how to improve the reconstructed image quality as far as possible. In this paper, we present an end-to-end V-DUnet for the reconstruction of 3D hyperspectral data in GISC spectral camera. To reduce the influence caused by the uncertainty of the measurement matrix and enhance the reconstructed image quality, both differential ghost imaging results and the detected measurements are sent into the network's inputs. Compared with compressive sensing algorithm, such as PICHCS and TwIST, it not only significantly improves the imaging quality with high noise immunity, but also speeds up the reconstruction time by more than two orders of magnitude.
更多查看译文
关键词
hyperspectral image reconstruction,hyperspectral camera,sparsity constraints,imaging,v-dunet
AI 理解论文
溯源树
样例
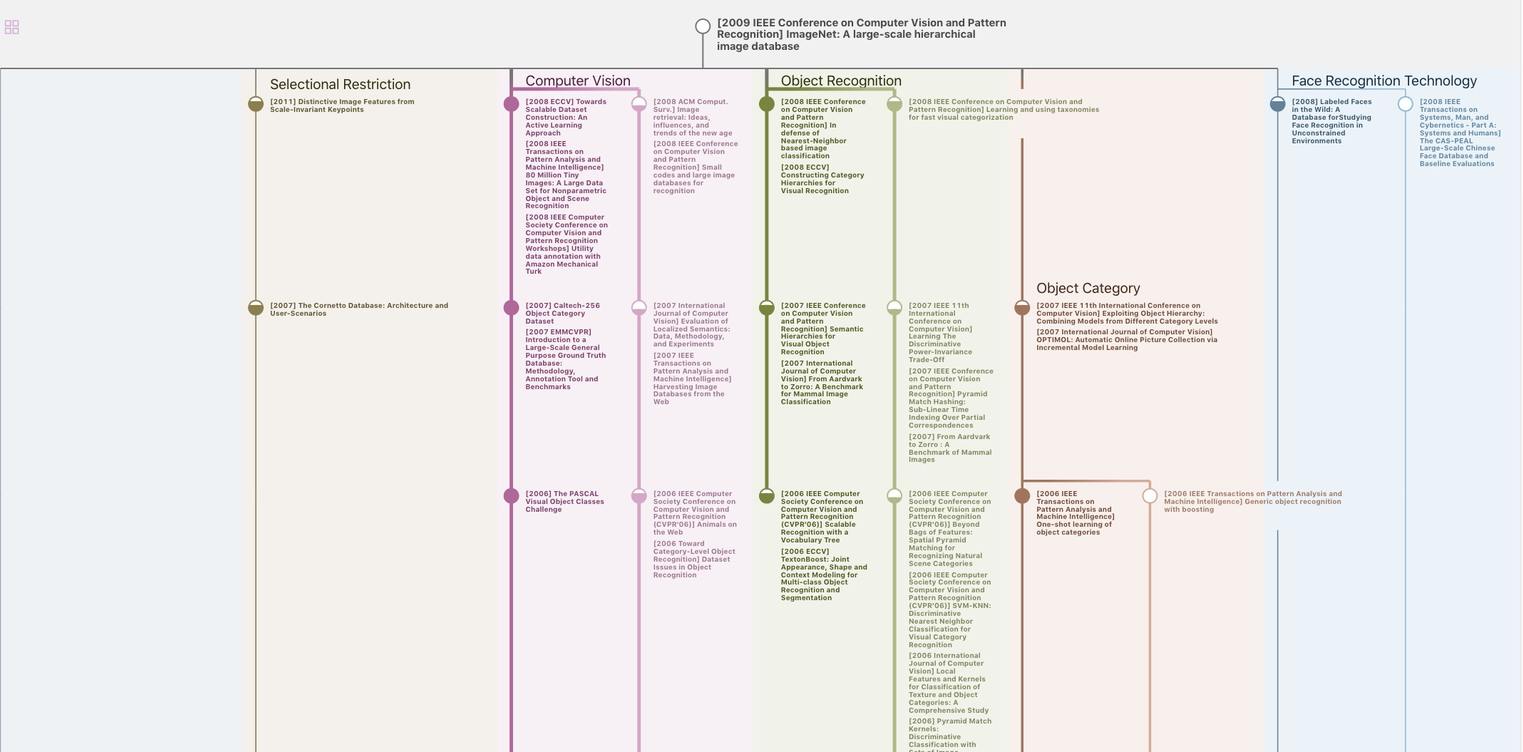
生成溯源树,研究论文发展脉络
Chat Paper
正在生成论文摘要