How to Steer Your Adversary: Targeted and Efficient Model Stealing Defenses with Gradient Redirection.
International Conference on Machine Learning(2022)
摘要
Model stealing attacks present a dilemma for public machine learning APIs. To protect financial investments, companies may be forced to withhold important information about their models that could facilitate theft, including uncertainty estimates and prediction explanations. This compromise is harmful not only to users but also to external transparency. Model stealing defenses seek to resolve this dilemma by making models harder to steal while preserving utility for benign users. However, existing defenses have poor performance in practice, either requiring enormous computational overheads or severe utility trade-offs. To meet these challenges, we present a new approach to model stealing defenses called gradient redirection. At the core of our approach is a provably optimal, efficient algorithm for steering an adversary’s training updates in a targeted manner. Combined with improvements to surrogate networks and a novel coordinated defense strategy, our gradient redirection defense, called GRAD^2, achieves small utility trade-offs and low computational overhead, outperforming the best prior defenses. Moreover, we demonstrate how gradient redirection enables reprogramming the adversary with arbitrary behavior, which we hope will foster work on new avenues of defense.
更多查看译文
关键词
efficient model stealing
AI 理解论文
溯源树
样例
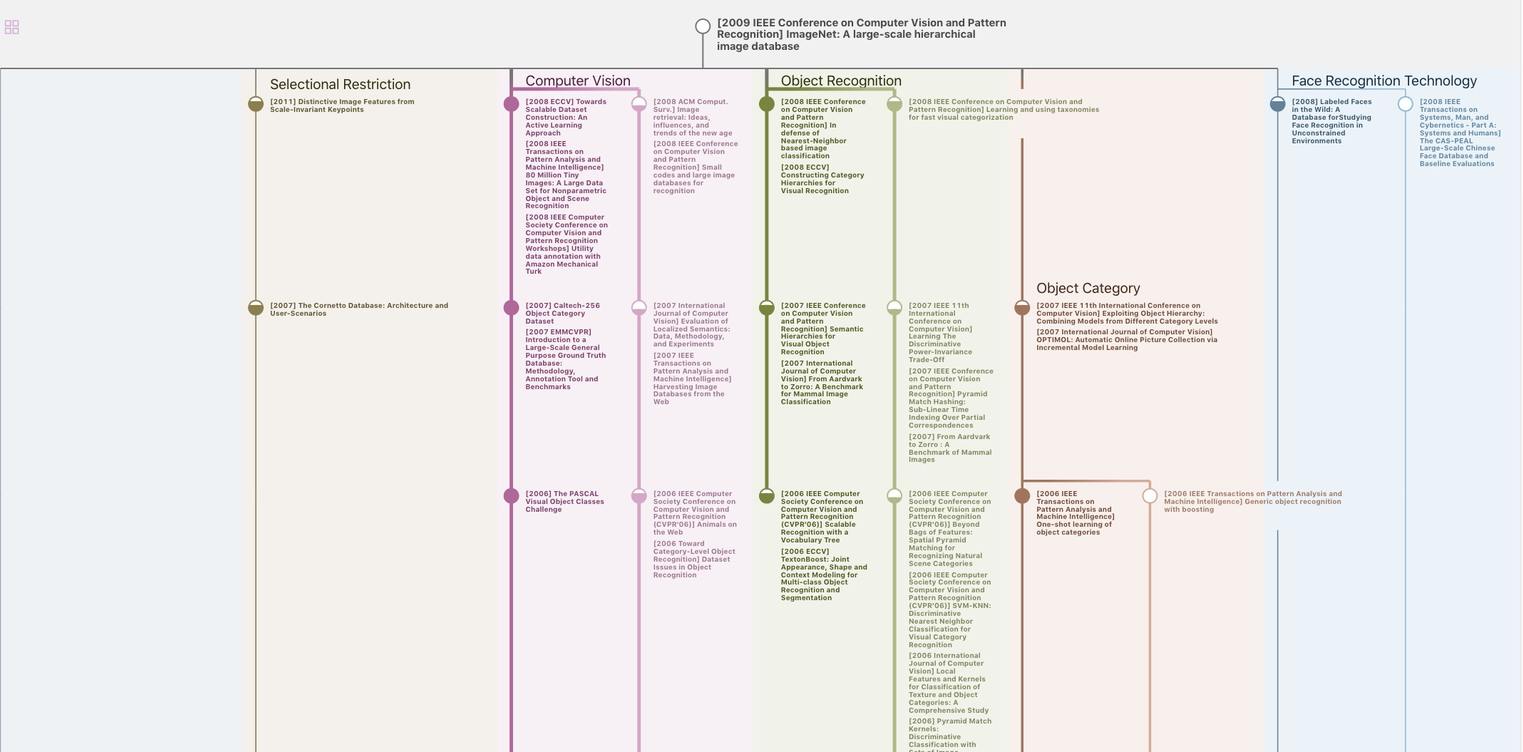
生成溯源树,研究论文发展脉络
Chat Paper
正在生成论文摘要