MACSA: A multimodal aspect-category sentiment analysis dataset with multimodal fine-grained aligned annotations
Multimedia Tools and Applications(2024)
摘要
As a fundamental task of fine-grained sentiment analysis, Aspect-Category Sentiment Analysis (ACSA) aims to predict the sentiment polarities of sentences with respect to given aspect categories. Previous works on ACSA are text-based, but with the increase of multimodal user-generated content (e.g. text and image), multimodal fine-grained sentiment analysis has attracted more attention in recent years. However, most of the existing multimodal fine-grained sentiment analysis work focuses on analyzing the sentiment of aspects that explicitly exist in the textual content. And there has been rare work on multimodal sentiment analysis of implicit categories in multimodal data, due to the lack of a sufficient dataset. In this paper, we introduce a new task, named Multimodal Aspect-Category Sentiment Analysis (MACSA), with the goal of predicting sentiment polarities of image-text pairs with respect to given aspect categories. And we propose a novel Multimodal Graph-based Aligned Network (MGAM) model for this task. Our model constructs heterogeneous graphs through multimodal fine-grained information and uses a convolutional graph neural network to learn cross-modal fine-grained interaction. We provide a new multimodal aspect category sentiment dataset, named the Hotel-MACSA dataset to evaluate our model, which contains multimodal fine-grained aligned annotations. The experimental results demonstrate the effectiveness of our proposed MGAM model for this new task. The MGAM model achieves an accuracy of 86.06
更多查看译文
关键词
Multimodal learning,Sentiment analysis,Dataset,Fine-grained learning
AI 理解论文
溯源树
样例
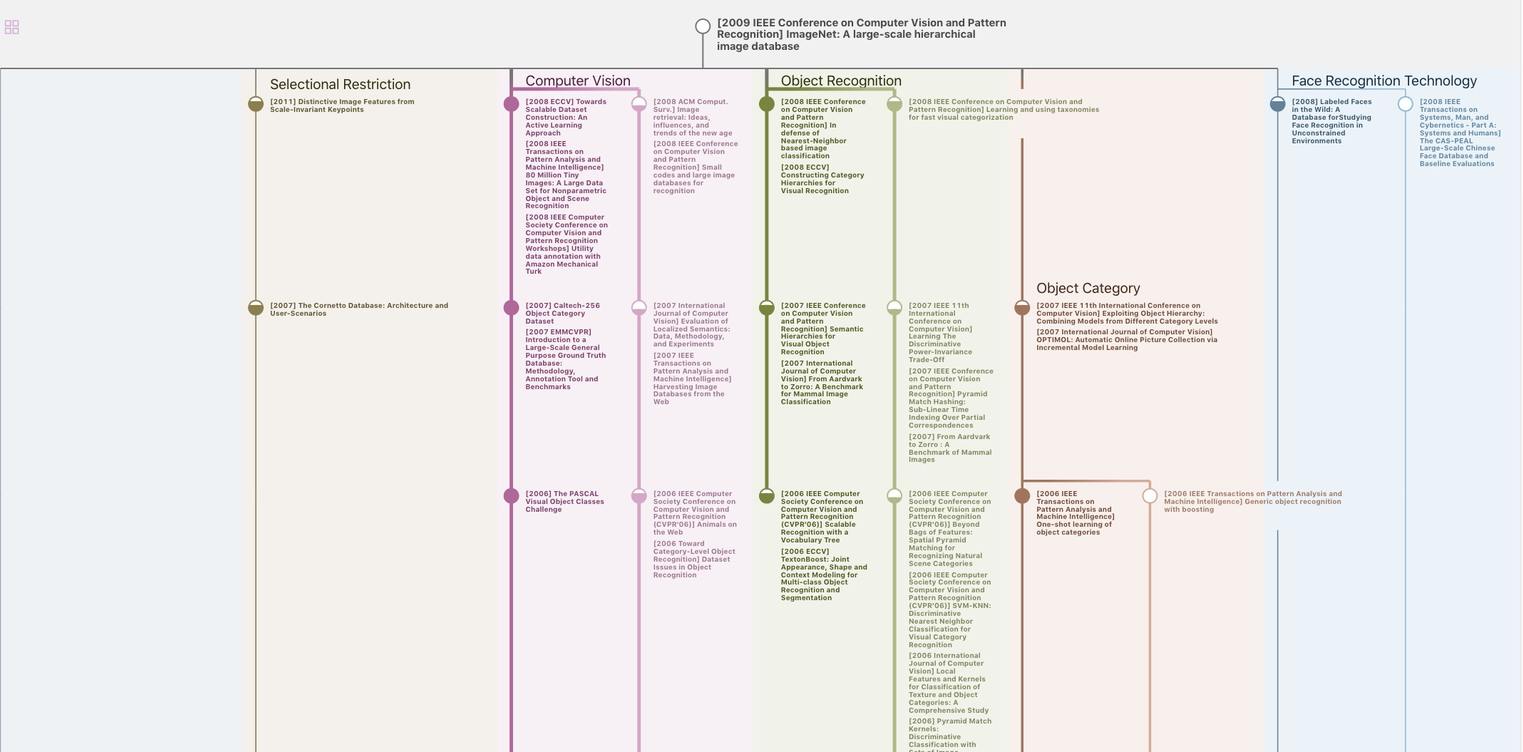
生成溯源树,研究论文发展脉络
Chat Paper
正在生成论文摘要