Synthetic-to-real: instance segmentation of clinical cluster cells with unlabeled synthetic training
BIOINFORMATICS(2022)
摘要
Motivation The presence of tumor cell clusters in pleural effusion may be a signal of cancer metastasis. The instance segmentation of single cell from cell clusters plays a pivotal role in cluster cell analysis. However, current cell segmentation methods perform poorly for cluster cells due to the overlapping/touching characters of clusters, multiple instance properties of cells, and the poor generalization ability of the models. Results In this article, we propose a contour constraint instance segmentation framework (CC framework) for cluster cells based on a cluster cell combination enhancement module. The framework can accurately locate each instance from cluster cells and realize high-precision contour segmentation under a few samples. Specifically, we propose the contour attention constraint module to alleviate over- and under-segmentation among individual cell-instance boundaries. In addition, to evaluate the framework, we construct a pleural effusion cluster cell dataset including 197 high-quality samples. The quantitative results show that the numeric result of AP(mask) is > 90%, a more than 10% increase compared with state-of-the-art semantic segmentation algorithms. From the qualitative results, we can observe that our method rarely has segmentation errors.
更多查看译文
关键词
clinical cluster cells,instance segmentation,synthetic-to-real
AI 理解论文
溯源树
样例
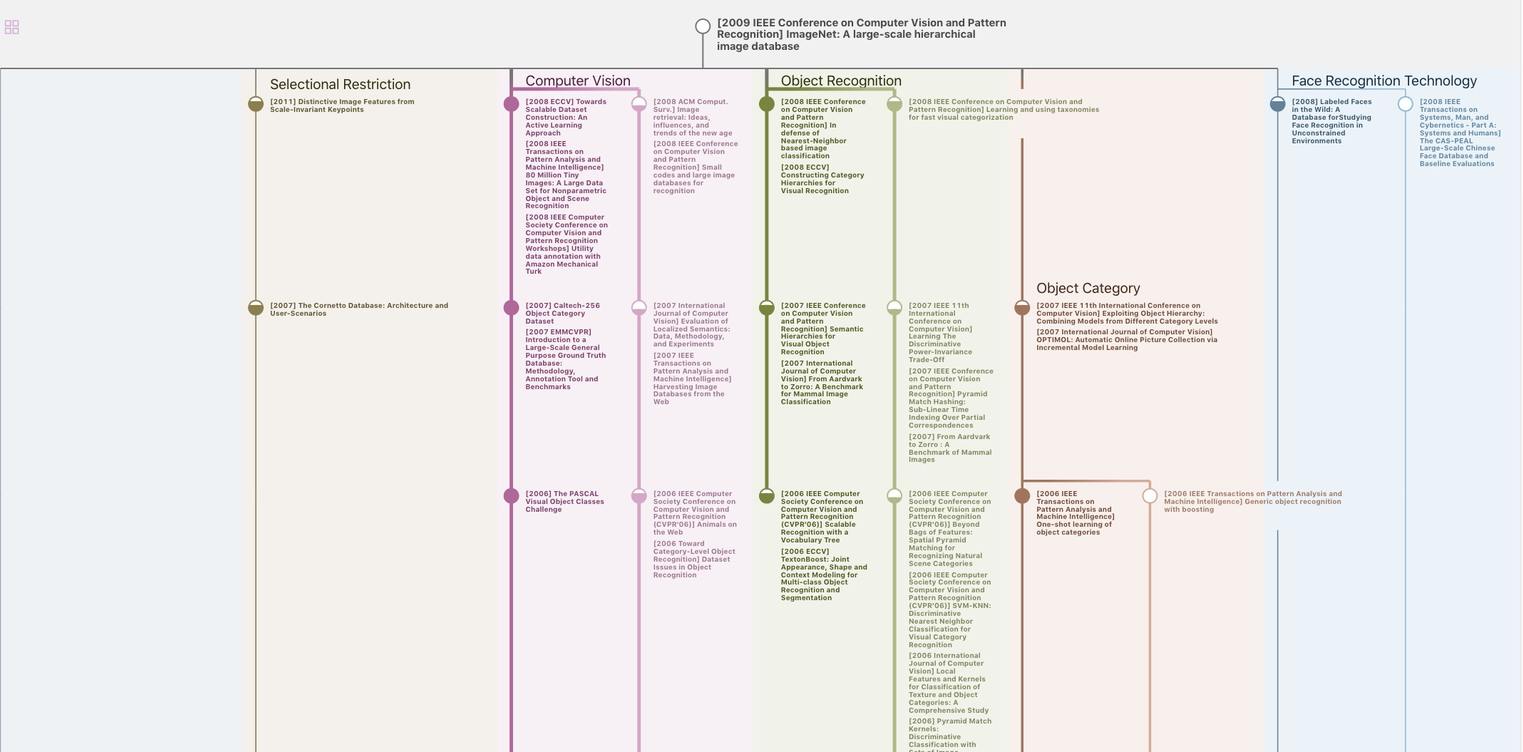
生成溯源树,研究论文发展脉络
Chat Paper
正在生成论文摘要