Efficiently Predicting Vancomycin Resistance of Enterococcus Faecium From MALDI-TOF MS Spectra Using a Deep Learning-Based Approach
FRONTIERS IN MICROBIOLOGY(2022)
摘要
Matrix-assisted laser desorption ionization time-of-flight (MALDI-TOF) mass spectrometry (MS) has recently become a useful analytical approach for microbial identification. The presence and absence of specific peaks on MS spectra are commonly used to identify the bacterial species and predict antibiotic-resistant strains. However, the conventional approach using few single peaks would result in insufficient prediction power without using complete information of whole MS spectra. In the past few years, machine learning algorithms have been successfully applied to analyze the MALDI-TOF MS peaks pattern for rapid strain typing. In this study, we developed a convolutional neural network (CNN) method to deal with the complete information of MALDI-TOF MS spectra for detecting Enterococcus faecium, which is one of the leading pathogens in the world. We developed a CNN model to rapidly and accurately predict vancomycin-resistant Enterococcus faecium (VREfm) samples from the whole mass spectra profiles of clinical samples. The CNN models demonstrated good classification performances with the average area under the receiver operating characteristic curve (AUROC) of 0.887 when using external validation data independently. Additionally, we employed the score-class activation mapping (CAM) method to identify the important features of our CNN models and found some discriminative signals that can substantially contribute to detecting the ion of resistance. This study not only utilized the complete information of MALTI-TOF MS data directly but also provided a practical means for rapid detection of VREfm using a deep learning algorithm.
更多查看译文
关键词
vancomycin-resistant Enterococcus faecium (VREfm), antibacterial drug resistance, MALDI-TOF MS, convolutional neural network (CNN), rapid detection
AI 理解论文
溯源树
样例
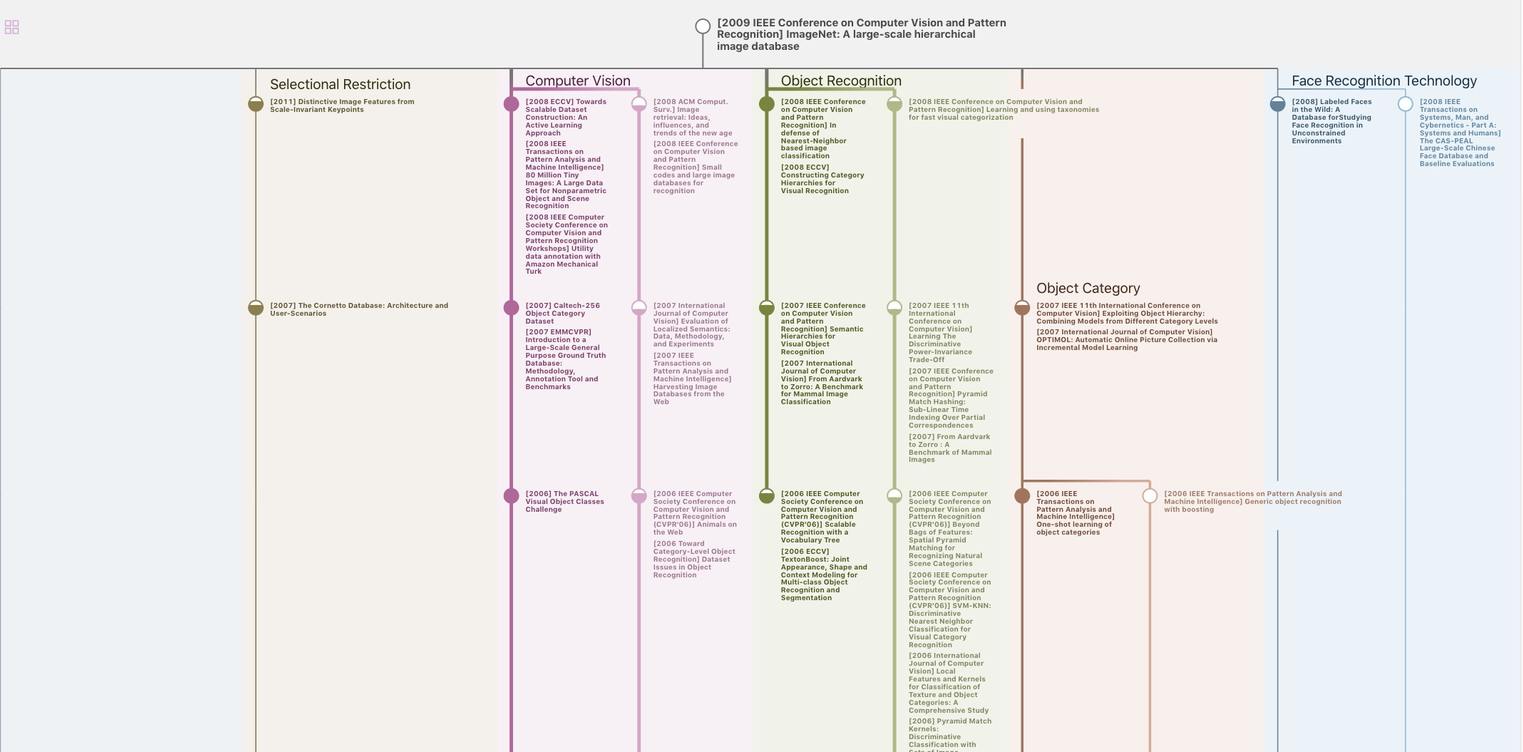
生成溯源树,研究论文发展脉络
Chat Paper
正在生成论文摘要