Images indexing and matched assessment of semantics and visuals similarities applied to a medical learning X-ray image base
JOURNAL OF X-RAY SCIENCE AND TECHNOLOGY(2022)
摘要
BACKGROUND: Medical diagnostic support systems are important tools in the field of radiology. However, the precision obtained, during the exploitation of high homogeneity image datasets, needs to be improved. OBJECTIVE: To develop a new learning system dedicated to public health practitioners. This study presents an upgraded version dedicated to radiology experts for better clinical decision-making when diagnosing and treating the patient (CAD approach). METHODS: Our system is a hybrid approach based on a matching of semantic and visual attributes of images. It is a combination of two complementary subsystems to form the intermodal system. The first one named alpha based on semantic attributes. Indexing and image retrieval based on specific keywords. The second system named beta based on low-level attributes. Vectors characterizing the digital content of the image (color, texture and shape) represent images. Our image database consists of 930 X-ray images including 320 mammograms acquired from the mini-MIAS database of mammograms and 610 X-rays acquired from the Public Hospital Establishment (EPH-Rouiba Algeria). The combination of two subsystems gives rise to the intermodal system: alpha-subsystem offers an overall result (based on visual descriptors), then beta-subsystem (low level descriptors) refines the result and increases relevance. RESULTS: Our system can perform a specific image search (in a database of images with very high homogeneity) with an accuracy of around 90% for a recall of 25%. The average (overall) accuracy of the system exceeds 70%. CONCLUSION: The results obtained are very encouraging, and demonstrate efficiency of our approach, particularly for the intermodal system.
更多查看译文
关键词
Image indexing, medical image, data base indexing, information retrieval, content base retrieval, query by image, cancer diagnosis
AI 理解论文
溯源树
样例
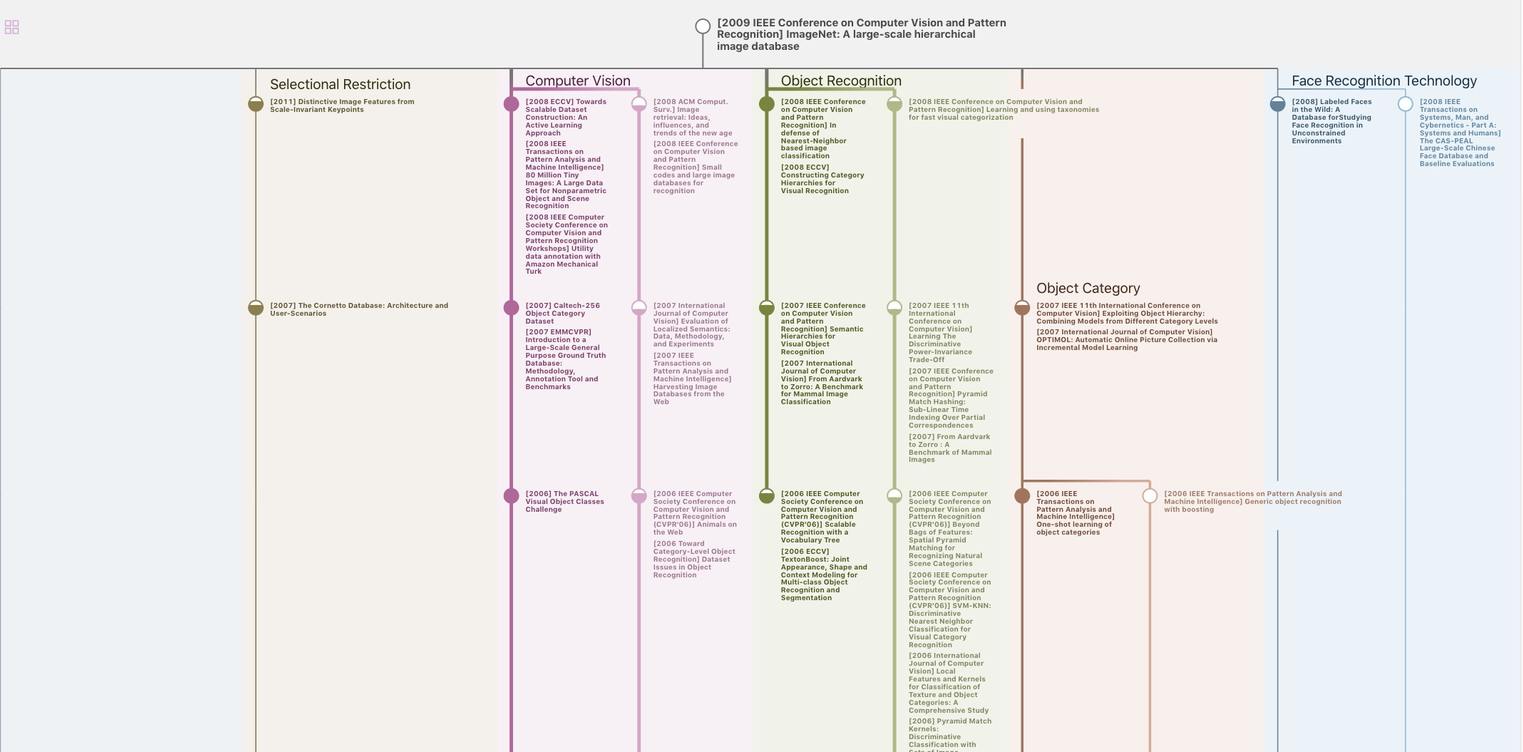
生成溯源树,研究论文发展脉络
Chat Paper
正在生成论文摘要