"Double vaccinated, 5G boosted!": Learning Attitudes towards COVID-19 Vaccination from Social Media
arxiv(2022)
摘要
To address the vaccine hesitancy which impairs the efforts of the COVID-19 vaccination campaign, it is imperative to understand public vaccination attitudes and timely grasp their changes. In spite of reliability and trustworthiness, conventional attitude collection based on surveys is time-consuming and expensive, and cannot follow the fast evolution of vaccination attitudes. We leverage the textual posts on social media to extract and track users' vaccination stances in near real time by proposing a deep learning framework. To address the impact of linguistic features such as sarcasm and irony commonly used in vaccine-related discourses, we integrate into the framework the recent posts of a user's social network neighbours to help detect the user's genuine attitude. Based on our annotated dataset from Twitter, the models instantiated from our framework can increase the performance of attitude extraction by up to 23% compared to state-of-the-art text-only models. Using this framework, we successfully validate the feasibility of using social media to track the evolution of vaccination attitudes in real life. We further show one practical use of our framework by validating the possibility to forecast a user's vaccine hesitancy changes with information perceived from social media.
更多查看译文
关键词
vaccination,social media,attitudes
AI 理解论文
溯源树
样例
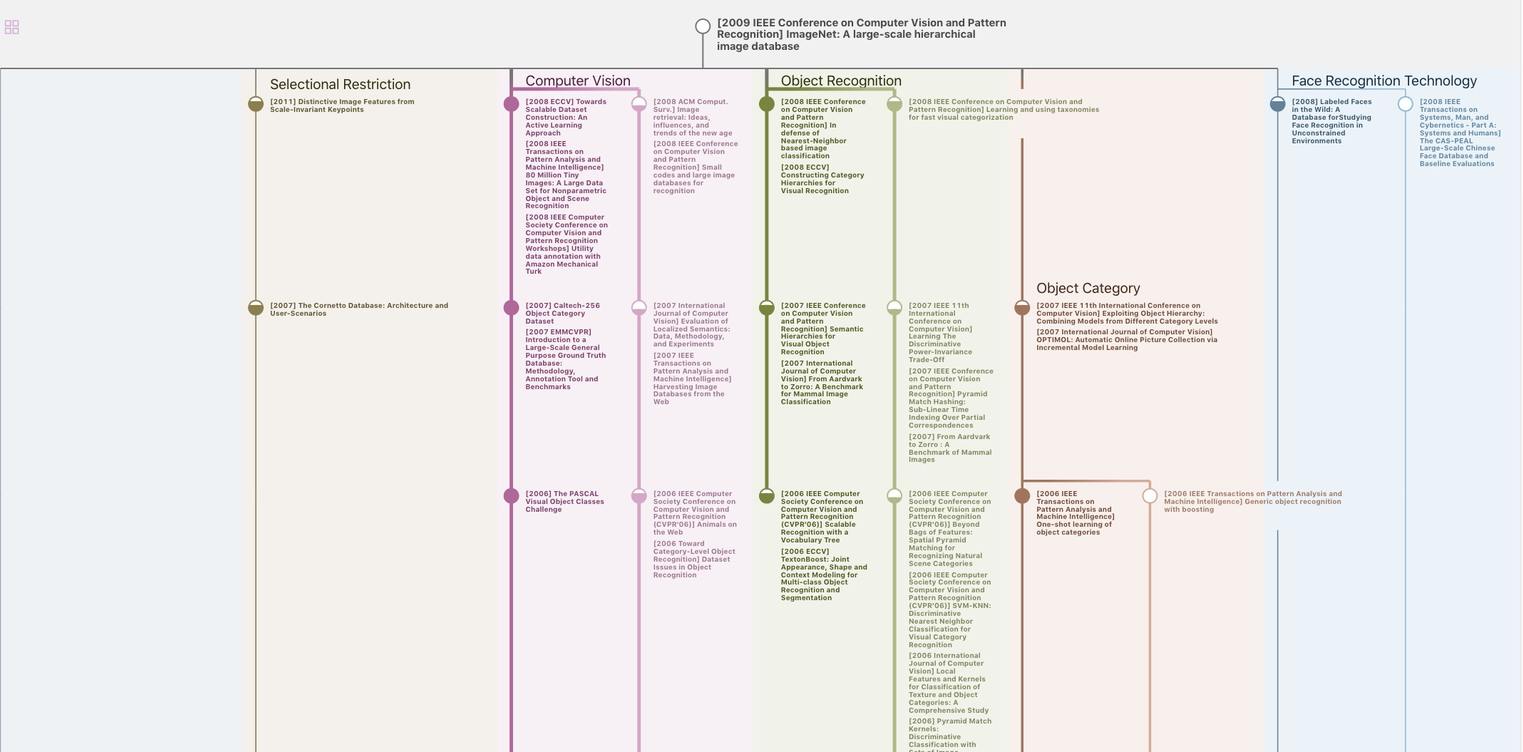
生成溯源树,研究论文发展脉络
Chat Paper
正在生成论文摘要