Supernova Light Curves Approximation based on Neural Network Models
arxiv(2023)
摘要
Photometric data-driven classification of supernovae becomes a challenge due to the appearance of real-time processing of big data in astronomy. Recent studies have demonstrated the superior quality of solutions based on various machine learning models. These models learn to classify supernova types using their light curves as inputs. Preprocessing these curves is a crucial step that significantly affects the final quality. In this talk, we study the application of multilayer perceptron (MLP), bayesian neural network (BNN), and normalizing flows (NF) to approximate observations for a single light curve. We use these approximations as inputs for supernovae classification models and demonstrate that the proposed methods outperform the state-of-the-art based on Gaussian processes applying to the Zwicky Transient Facility Bright Transient Survey light curves. MLP demonstrates similar quality as Gaussian processes and speed increase. Normalizing Flows exceeds Gaussian processes in terms of approximation quality as well.
更多查看译文
关键词
neural network,curves,models
AI 理解论文
溯源树
样例
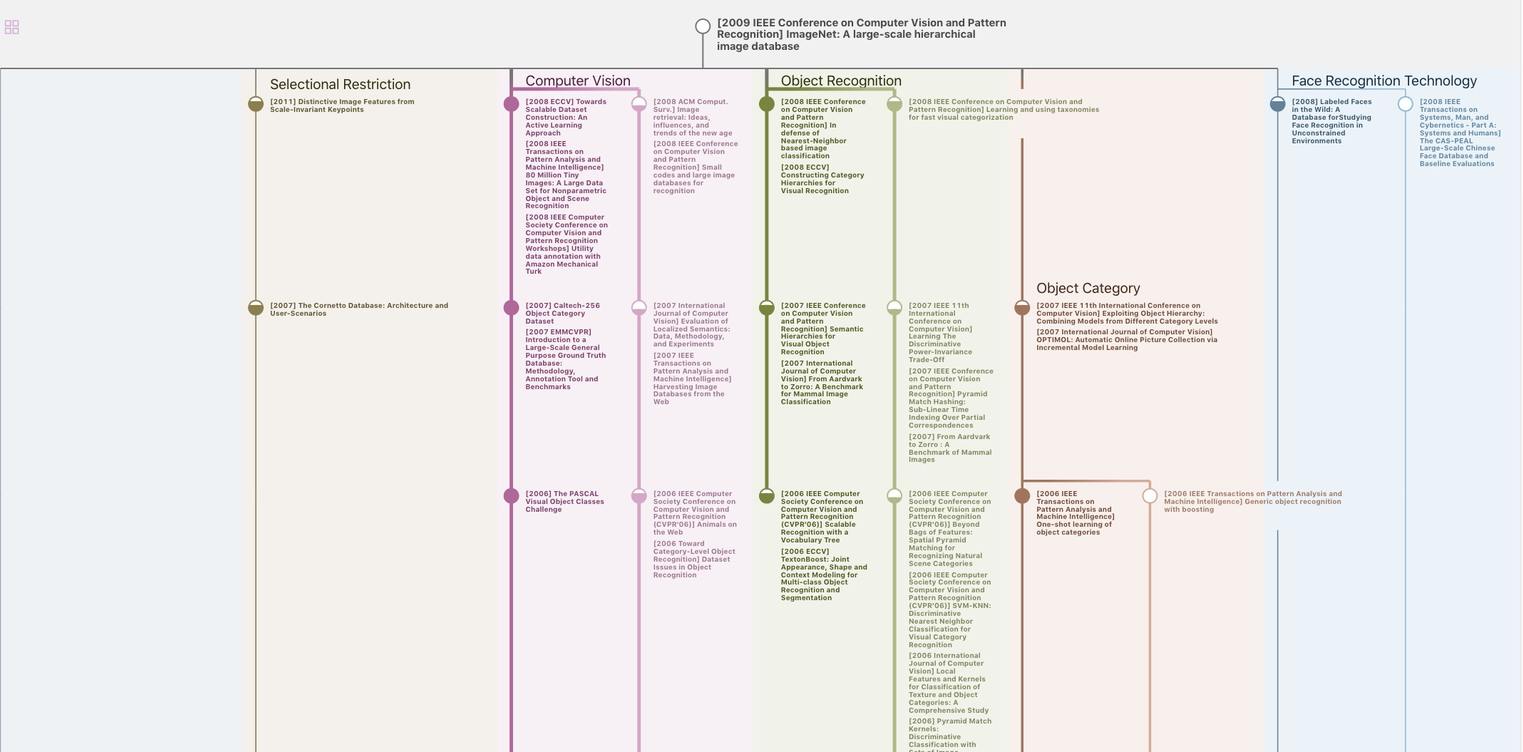
生成溯源树,研究论文发展脉络
Chat Paper
正在生成论文摘要