Local Evaluation of Time Series Anomaly Detection Algorithms
KDD '22: Proceedings of the 28th ACM SIGKDD Conference on Knowledge Discovery and Data Mining(2022)
摘要
In recent years, specific evaluation metrics for time series anomaly detection algorithms have been developed to handle the limitations of the classical precision and recall. However, such metrics are heuristically built as an aggregate of multiple desirable aspects, introduce parameters and wipe out the interpretability of the output. In this article, we first highlight the limitations of the classical precision/recall, as well as the main issues of the recent event-based metrics -- for instance, we show that an adversary algorithm can reach high precision and recall on almost any dataset under weak assumption. To cope with the above problems, we propose a theoretically grounded, robust, parameter-free and interpretable extension to precision/recall metrics, based on the concept of "affiliation'' between the ground truth and the prediction sets. Our metrics leverage measures of duration between ground truth and predictions, and have thus an intuitive interpretation. By further comparison against random sampling, we obtain a normalized precision/recall, quantifying how much a given set of results is better than a random baseline prediction. By construction, our approach keeps the evaluation local regarding ground truth events, enabling fine-grained visualization and interpretation of algorithmic results. We compare our proposal against various public time series anomaly detection datasets, algorithms and metrics. We further derive theoretical properties of the affiliation metrics that give explicit expectations about their behavior and ensure robustness against adversary strategies.
更多查看译文
关键词
anomaly detection,algorithms
AI 理解论文
溯源树
样例
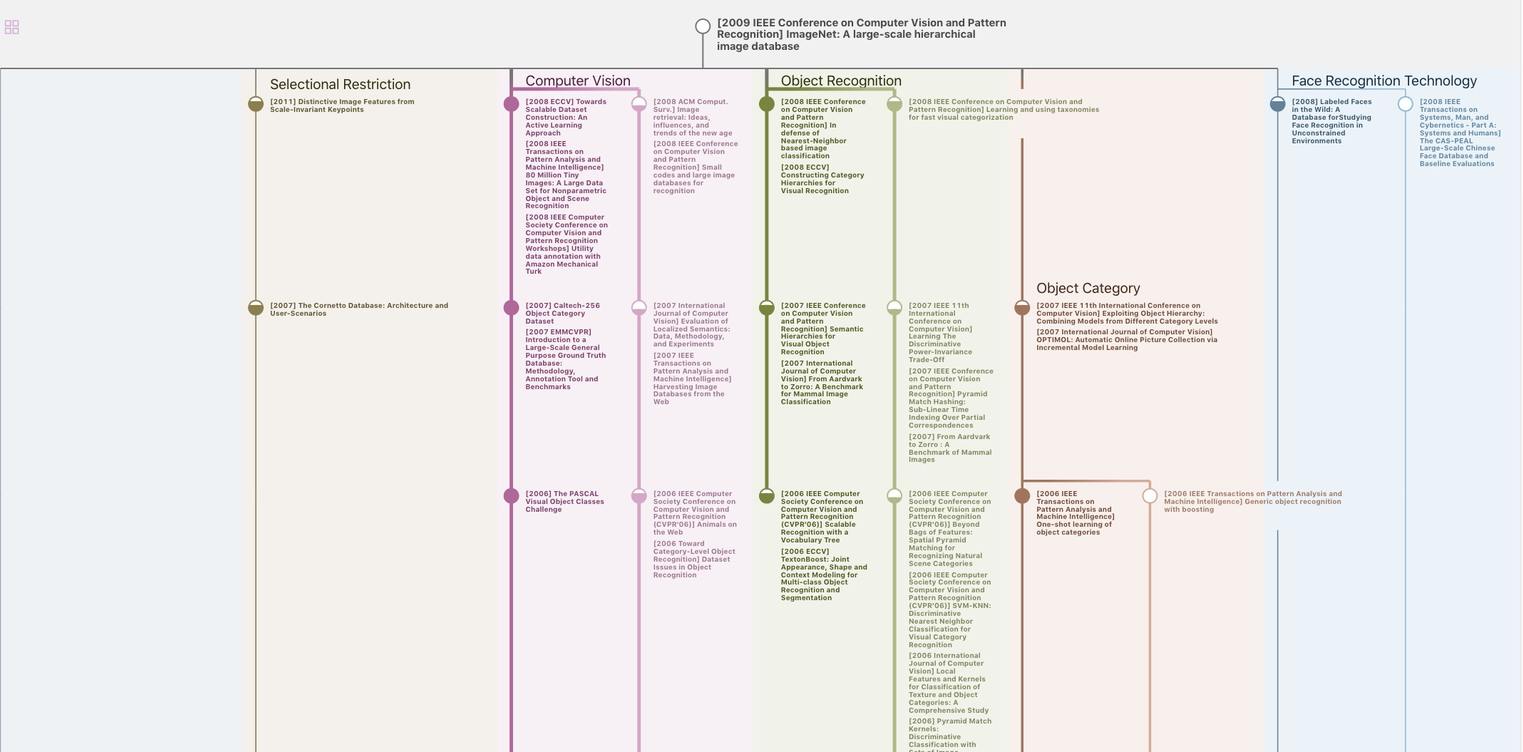
生成溯源树,研究论文发展脉络
Chat Paper
正在生成论文摘要