Modeling Content Creator Incentives on Algorithm-Curated Platforms
ICLR 2023(2022)
摘要
Content creators compete for user attention. Their reach crucially depends on algorithmic choices made by developers on online platforms. To maximize exposure, many creators adapt strategically, as evidenced by examples like the sprawling search engine optimization industry. This begets competition for the finite user attention pool. We formalize these dynamics in what we call an exposure game, a model of incentives induced by algorithms including modern factorization and (deep) two-tower architectures. We prove that seemingly innocuous algorithmic choices -- e.g., non-negative vs. unconstrained factorization -- significantly affect the existence and character of (Nash) equilibria in exposure games. We proffer use of creator behavior models like ours for an (ex-ante) pre-deployment audit. Such an audit can identify misalignment between desirable and incentivized content, and thus complement post-hoc measures like content filtering and moderation. To this end, we propose tools for numerically finding equilibria in exposure games, and illustrate results of an audit on the MovieLens and LastFM datasets. Among else, we find that the strategically produced content exhibits strong dependence between algorithmic exploration and content diversity, and between model expressivity and bias towards gender-based user and creator groups.
更多查看译文
关键词
Nash equilibria,producer incentives,attention monetizing platforms,recommenders,differentiable games,exposure game
AI 理解论文
溯源树
样例
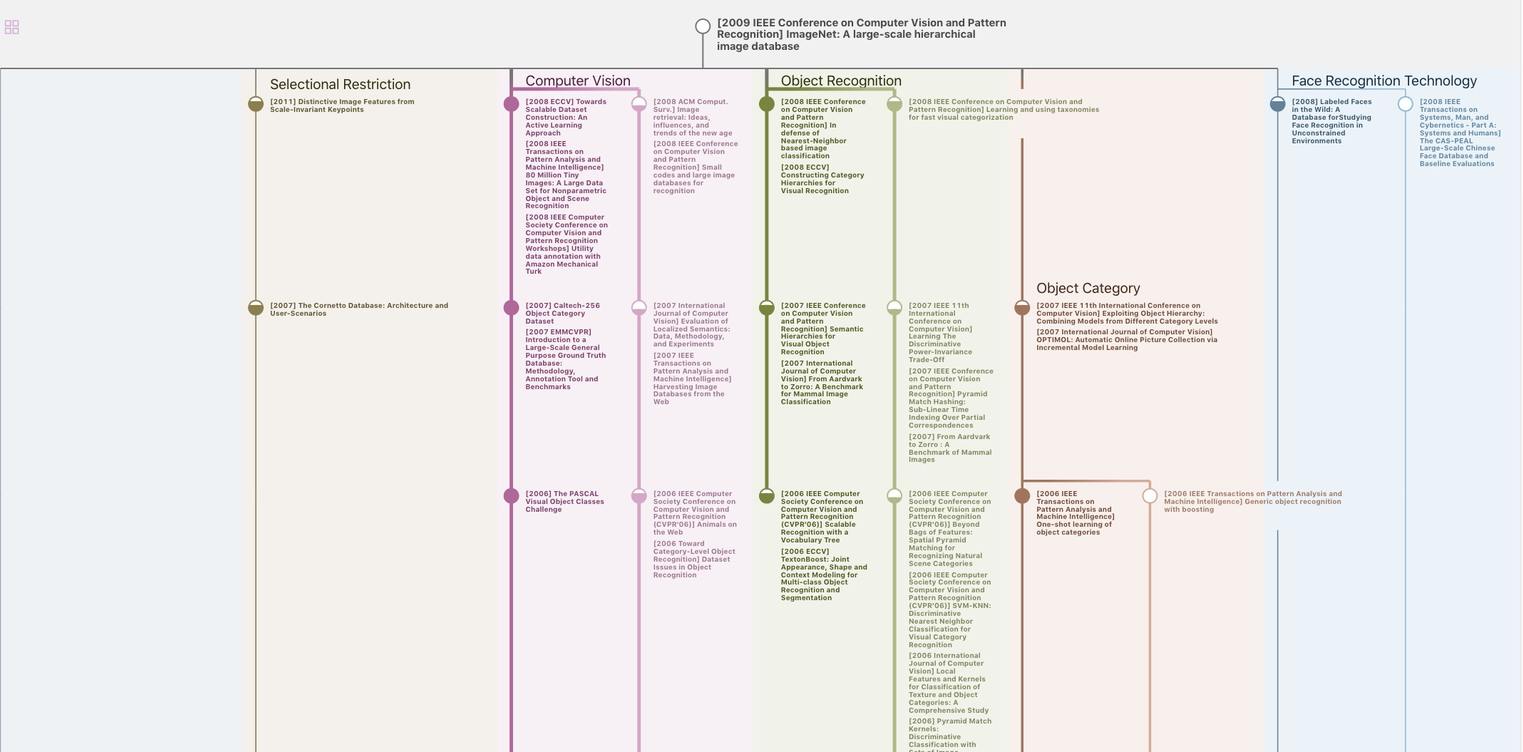
生成溯源树,研究论文发展脉络
Chat Paper
正在生成论文摘要