AFT-VO: Asynchronous Fusion Transformers for Multi-View Visual Odometry Estimation.
IEEE/RJS International Conference on Intelligent RObots and Systems (IROS)(2022)
摘要
Motion estimation approaches typically employ sensor fusion techniques, such as the Kalman Filter, to handle individual sensor failures. More recently, deep learning-based fusion approaches have been proposed, increasing the performance and requiring less model-specific implementations. However, current deep fusion approaches often assume that sensors are synchronised, which is not always practical, especially for low-cost hardware. To address this limitation, in this work, we propose AFT-VO, a novel transformer-based sensor fusion architecture to estimate VO from multiple sensors. Our framework combines predictions from asynchronous multi-view cameras and accounts for the time discrepancies of measurements coming from different sources. Our approach first employs a Mixture Density Network (MDN) to estimate the probability distributions of the 6-DoF poses for every camera in the system. Then a novel transformer-based fusion module, AFT-VO, is introduced, which combines these asynchronous pose estimations, along with their confidences. More specifically, we introduce Discretiser and Source Encoding techniques which enable the fusion of multi-source asynchronous signals. We evaluate our approach on the popular nuScenes and KITTI datasets. Our experiments demonstrate that multi-view fusion for VO estimation provides robust and accurate trajectories, outperforming the state of the art in both challenging weather and lighting conditions.
更多查看译文
关键词
asynchronous fusion transformers,estimation,multi-view
AI 理解论文
溯源树
样例
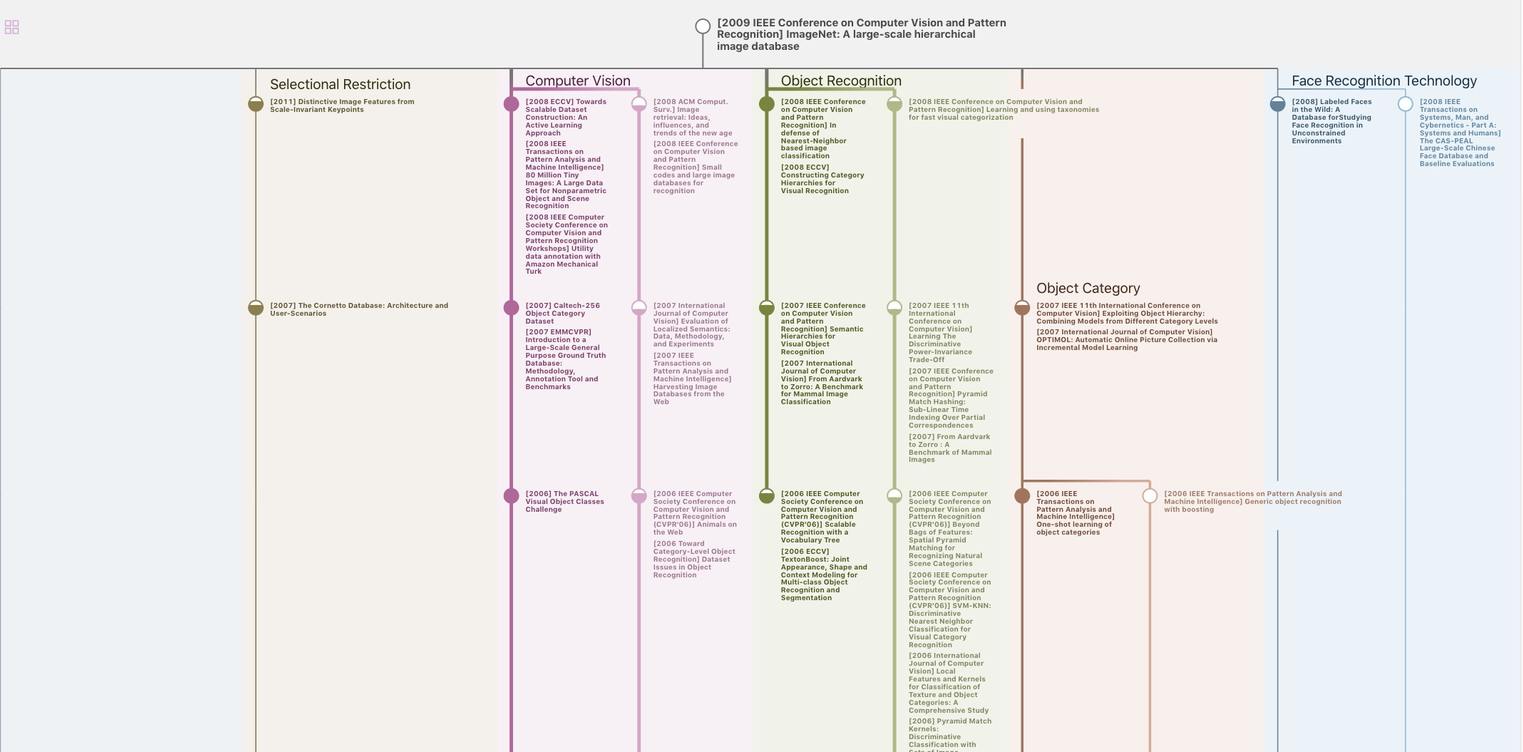
生成溯源树,研究论文发展脉络
Chat Paper
正在生成论文摘要