Robustness to corruption in pre-trained Bayesian neural networks
arxiv(2023)
摘要
We develop ShiftMatch, a new training-data-dependent likelihood for robustness to corruption in Bayesian neural networks (BNNs). ShiftMatch is inspired by the training-data-dependent "EmpCov" priors from Izmailov et al. (2021a), and efficiently matches test-time spatial correlations to those at training time. Critically, ShiftMatch is designed to leave the neural network's training time likelihood unchanged, allowing it to use publicly available samples from pre-trained BNNs. Using pre-trained HMC samples, ShiftMatch gives strong performance improvements on CIFAR-10-C, outperforms EmpCov priors (though ShiftMatch uses extra information from a minibatch of corrupted test points), and is perhaps the first Bayesian method capable of convincingly outperforming plain deep ensembles.
更多查看译文
关键词
bayesian neural networks,robustness,neural networks,corruption,pre-trained
AI 理解论文
溯源树
样例
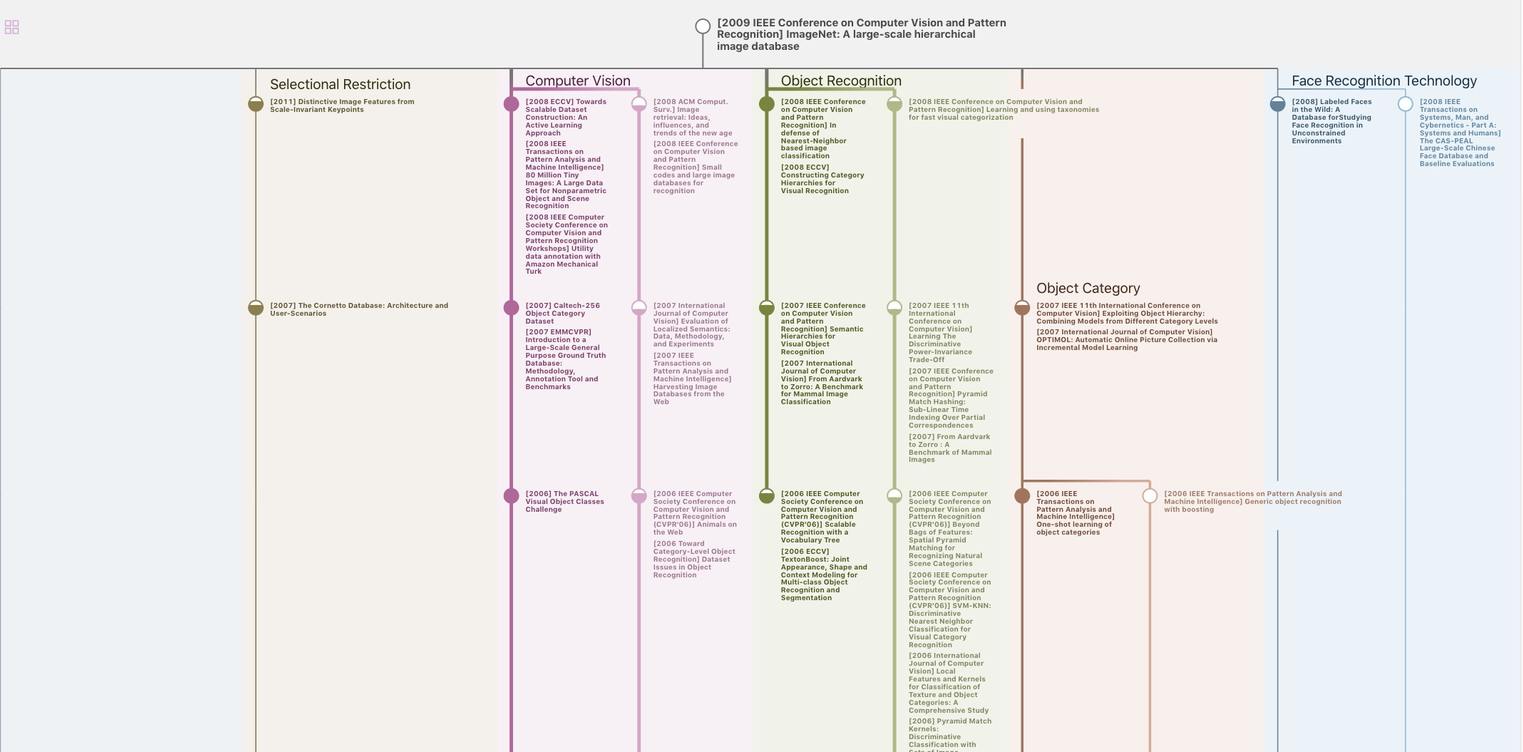
生成溯源树,研究论文发展脉络
Chat Paper
正在生成论文摘要