Low- and Mixed-Precision Inference Accelerators
arxiv(2022)
摘要
With the surging popularity of edge computing, the need to efficiently perform neural network inference on battery-constrained IoT devices has greatly increased. While algorithmic developments enable neural networks to solve increasingly more complex tasks, the deployment of these networks on edge devices can be problematic due to the stringent energy, latency, and memory requirements. One way to alleviate these requirements is by heavily quantizing the neural network, i.e. lowering the precision of the operands. By taking quantization to the extreme, e.g. by using binary values, new opportunities arise to increase the energy efficiency. Several hardware accelerators exploiting the opportunities of low-precision inference have been created, all aiming at enabling neural network inference at the edge. In this chapter, design choices and their implications on the flexibility and energy efficiency of several accelerators supporting extremely quantized networks are reviewed.
更多查看译文
关键词
mixed-precision
AI 理解论文
溯源树
样例
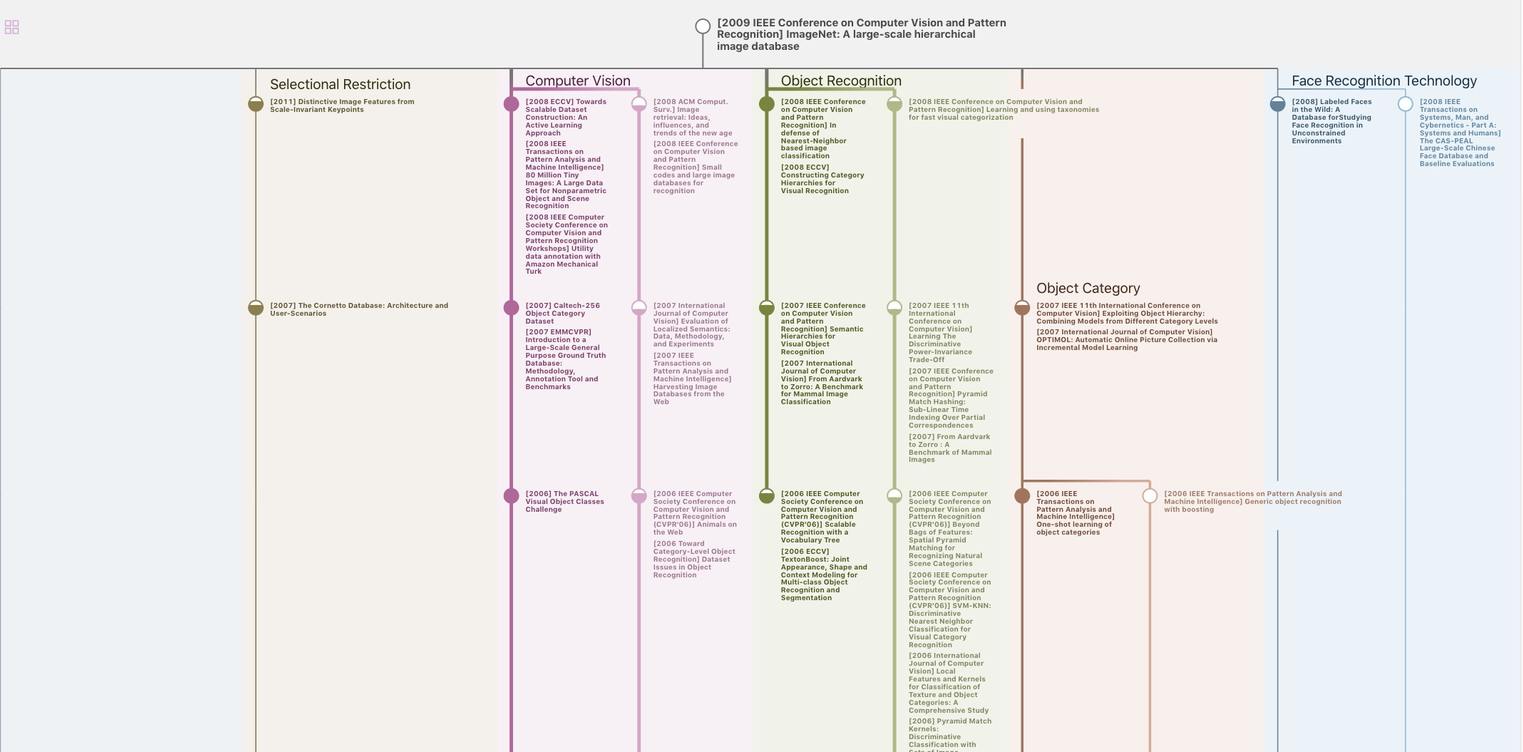
生成溯源树,研究论文发展脉络
Chat Paper
正在生成论文摘要