Generative Datalog with Stable Negation
PROCEEDINGS OF THE 42ND ACM SIGMOD-SIGACT-SIGAI SYMPOSIUM ON PRINCIPLES OF DATABASE SYSTEMS, PODS 2023(2023)
摘要
Extending programming languages with stochastic behaviour such as probabilistic choices or random sampling has a long tradition in computer science. A recent development in this direction is a declarative probabilistic programming language, proposed by Barany et al. in 2017, which operates on standard relational databases. In particular, Barany et al. proposed generative Datalog, a probabilistic extension of Datalog that allows sampling from discrete probability distributions. Intuitively, the output of a generative Datalog program Pi on an input database D is a probability space over the minimal models of D and Pi, the so-called possible outcomes. This is a natural generalization of the (deterministic) semantics of Datalog, where the output of a program on a database is their unique minimal model. A natural question to ask is how generative Datalog can be enriched with the useful feature of negation, which in turn leads to a strictly more expressive declarative probabilistic programming language. In particular, the challenging question is how the probabilistic semantics of generative Datalog with negation can be robustly defined. Our goal is to provide an answer to this question by interpreting negation according to the stable model semantics.
更多查看译文
关键词
probabilistic programming,generative Datalog,negation,stable model semantics
AI 理解论文
溯源树
样例
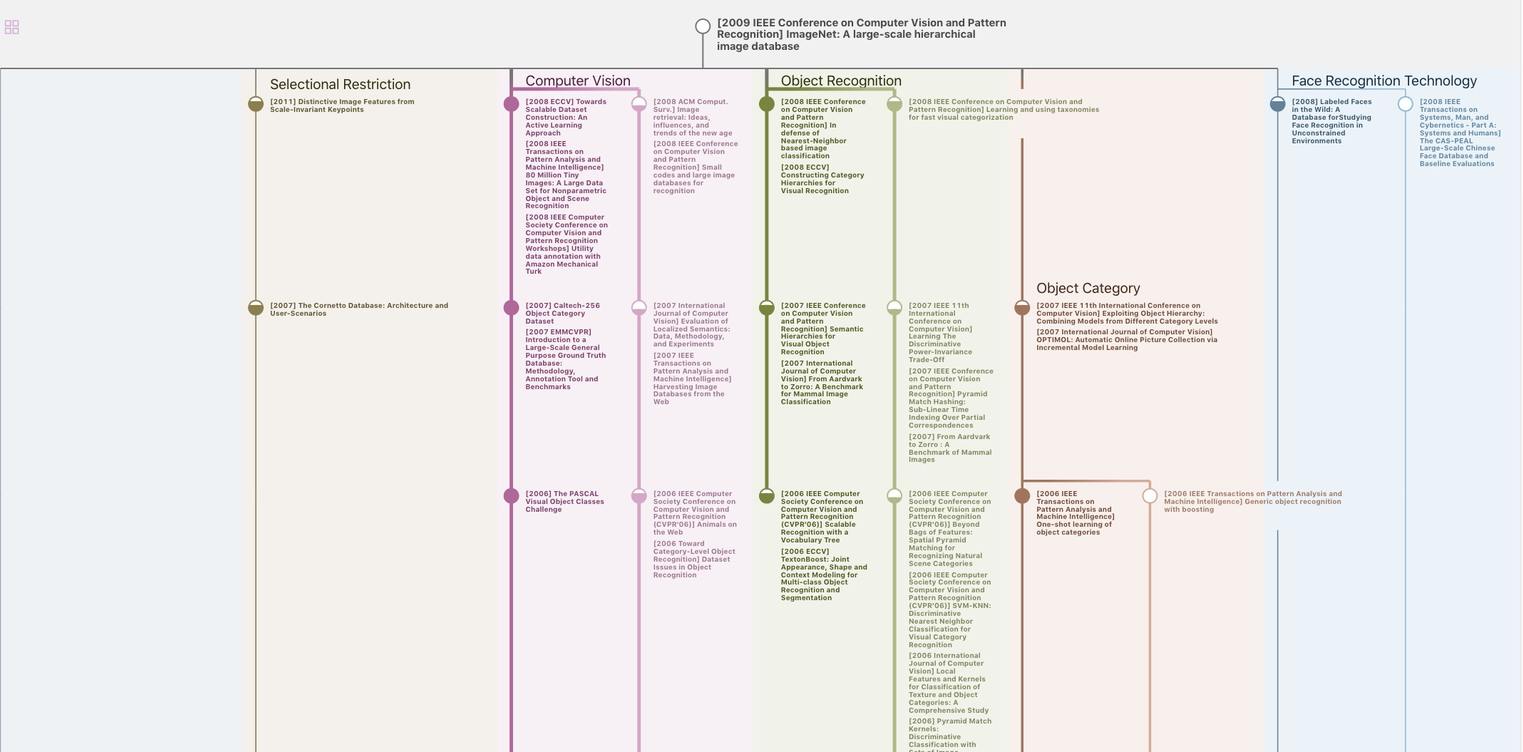
生成溯源树,研究论文发展脉络
Chat Paper
正在生成论文摘要