zPROBE: Zero Peek Robustness Checks for Federated Learning
arxiv(2022)
摘要
Privacy-preserving federated learning allows multiple users to jointly train a model with coordination of a central server. The server only learns the final aggregation result, thus the users' (private) training data is not leaked from the individual model updates. However, keeping the individual updates private allows malicious users to perform Byzantine attacks and degrade the accuracy without being detected. Best existing defenses against Byzantine workers rely on robust rank-based statistics, e.g., median, to find malicious updates. However, implementing privacy-preserving rank-based statistics is nontrivial and not scalable in the secure domain, as it requires sorting all individual updates. We establish the first private robustness check that uses high break point rank-based statistics on aggregated model updates. By exploiting randomized clustering, we significantly improve the scalability of our defense without compromising privacy. We leverage our statistical bounds in zero-knowledge proofs to detect and remove malicious updates without revealing the private user updates. Our novel framework, zPROBE, enables Byzantine resilient and secure federated learning. Empirical evaluations demonstrate that zPROBE provides a low overhead solution to defend against state-of-the-art Byzantine attacks while preserving privacy.
更多查看译文
关键词
peek robustness checks
AI 理解论文
溯源树
样例
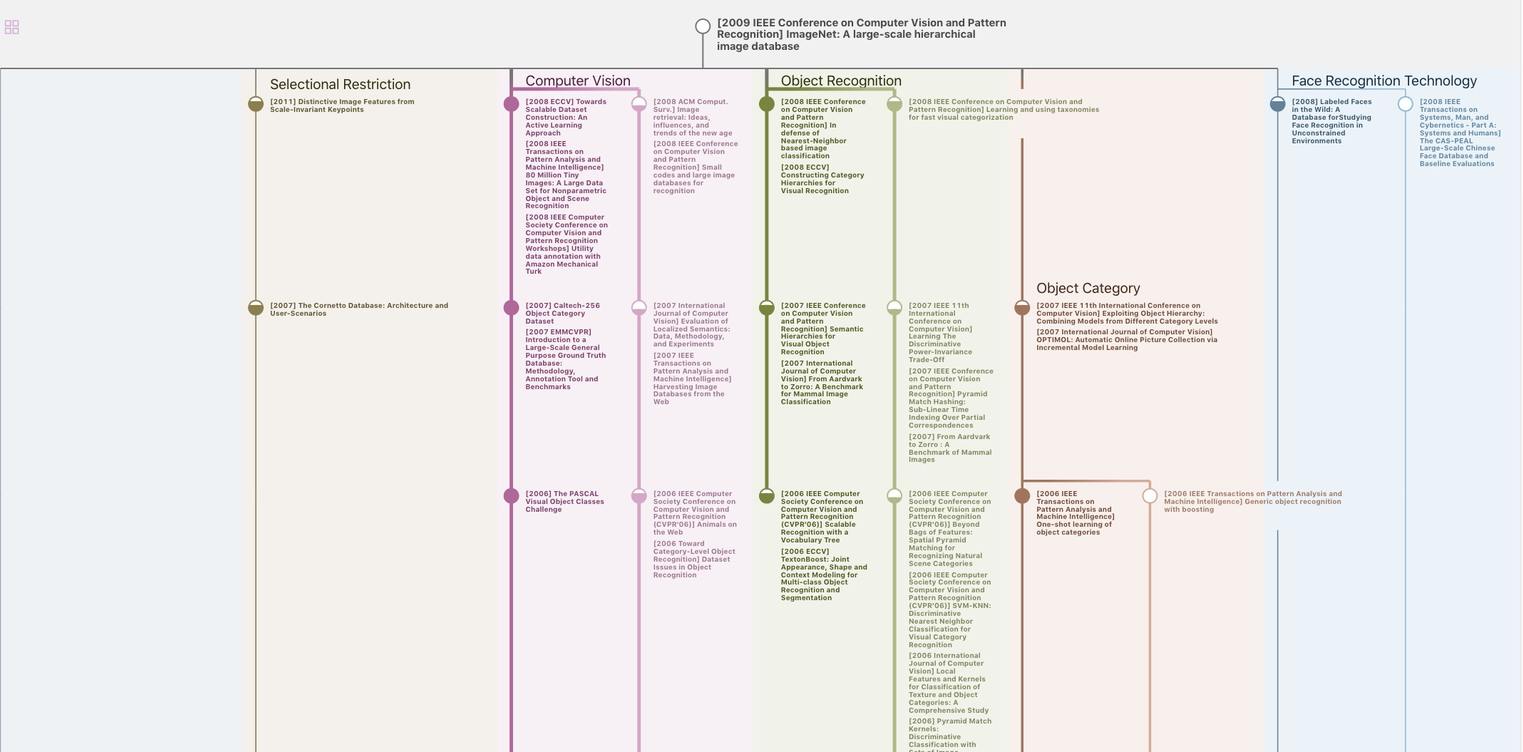
生成溯源树,研究论文发展脉络
Chat Paper
正在生成论文摘要