LPG-PCFG: An Improved Probabilistic Context- Free Grammar to Hit Low-Probability Passwords
SENSORS(2022)
摘要
With the development of the Internet, information security has attracted more attention. Identity authentication based on password authentication is the first line of defense; however, the password-generation model is widely used in offline password attacks and password strength evaluation. In real attack scenarios, high-probability passwords are easy to enumerate; extremely low-probability passwords usually lack semantic structure and, so, are tough to crack by applying statistical laws in machine learning models, but these passwords with lower probability have a large search space and certain semantic information. Improving the low-probability password hit rate in this interval is of great significance for improving the efficiency of offline attacks. However, obtaining a low-probability password is difficult under the current password-generation model. To solve this problem, we propose a low-probability generator-probabilistic context-free grammar (LPG-PCFG) based on PCFG. LPG-PCFG directionally increases the probability of low-probability passwords in the models' distribution, which is designed to obtain a degeneration distribution that is friendly for generating low-probability passwords. By using the control variable method to fine-tune the degeneration of LPG-PCFG, we obtained the optimal combination of degeneration parameters. Compared with the non-degeneration PCFG model, LPG-PCFG generates a larger number of hits. When generating 107 and 108 times, the number of hits to low-probability passwords increases by 50.4% and 42.0%, respectively.
更多查看译文
关键词
information security, password-generation model, PCFG, low-probability password, degeneration distribution
AI 理解论文
溯源树
样例
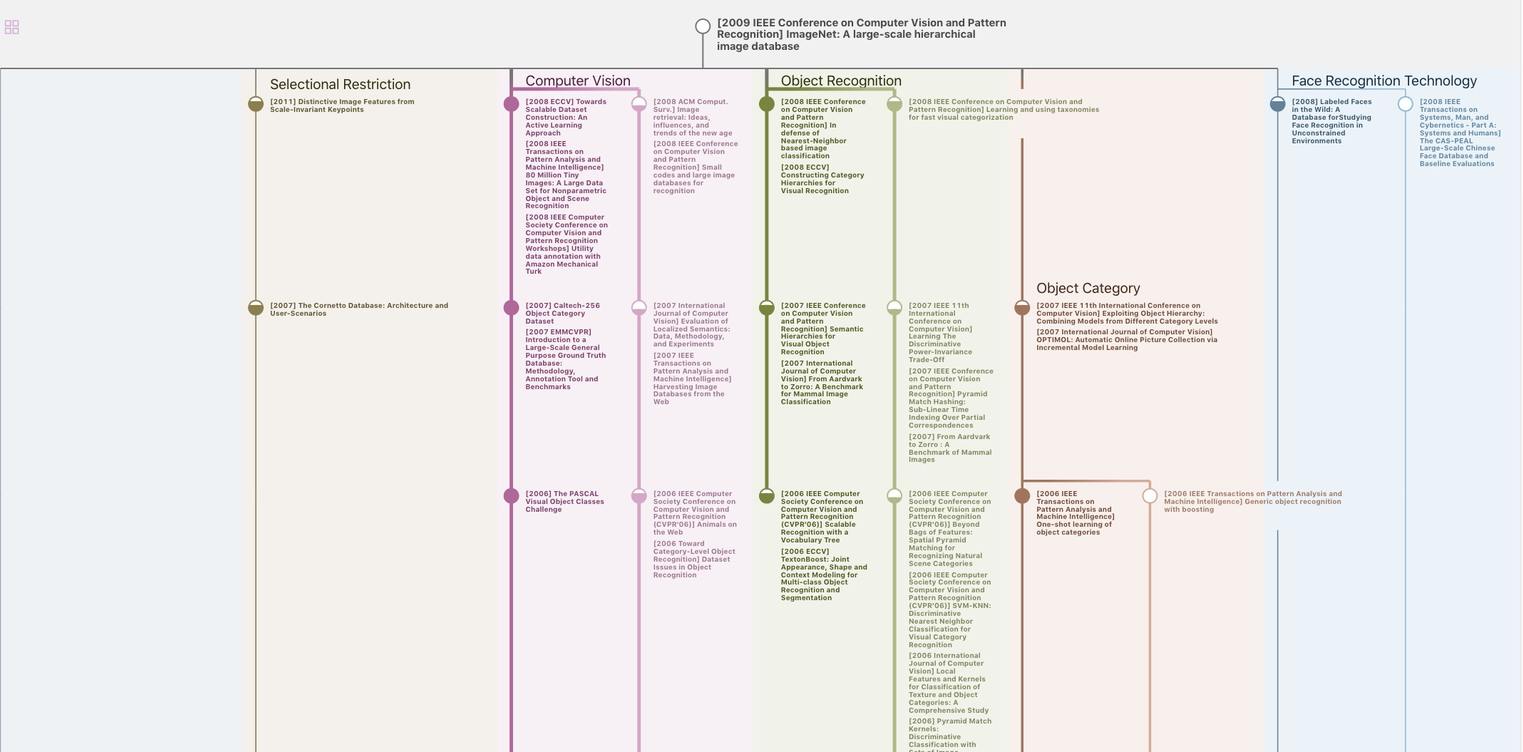
生成溯源树,研究论文发展脉络
Chat Paper
正在生成论文摘要