Hyperparameter Optimization of Bayesian Neural Network Using Bayesian Optimization and Intelligent Feature Engineering for Load Forecasting
SENSORS(2022)
摘要
This paper proposes a new hybrid framework for short-term load forecasting (STLF) by combining the Feature Engineering (FE) and Bayesian Optimization (BO) algorithms with a Bayesian Neural Network (BNN). The FE module comprises feature selection and extraction phases. Firstly, by merging the Random Forest (RaF) and Relief-F (ReF) algorithms, we developed a hybrid feature selector based on grey correlation analysis (GCA) to eliminate feature redundancy. Secondly, a radial basis Kernel function and principal component analysis (KPCA) are integrated into the feature-extraction module for dimensional reduction. Thirdly, the Bayesian Optimization (BO) algorithm is used to fine-tune the control parameters of a BNN and provides more accurate results by avoiding the optimal local trapping. The proposed FE-BNN-BO framework works in such a way to ensure stability, convergence, and accuracy. The proposed FE-BNN-BO model is tested on the hourly load data obtained from the PJM, USA, electricity market. In addition, the simulation results are also compared with other benchmark models such as Bi-Level, long short-term memory (LSTM), an accurate and fast convergence-based ANN (ANN-AFC), and a mutual-information-based ANN (ANN-MI). The results show that the proposed model has significantly improved the accuracy with a fast convergence rate and reduced the mean absolute percent error (MAPE).
更多查看译文
关键词
Bayesian Neural Networks,Bayesian Optimization,convergence rate,Hamilton dynamic,electric load forecasting
AI 理解论文
溯源树
样例
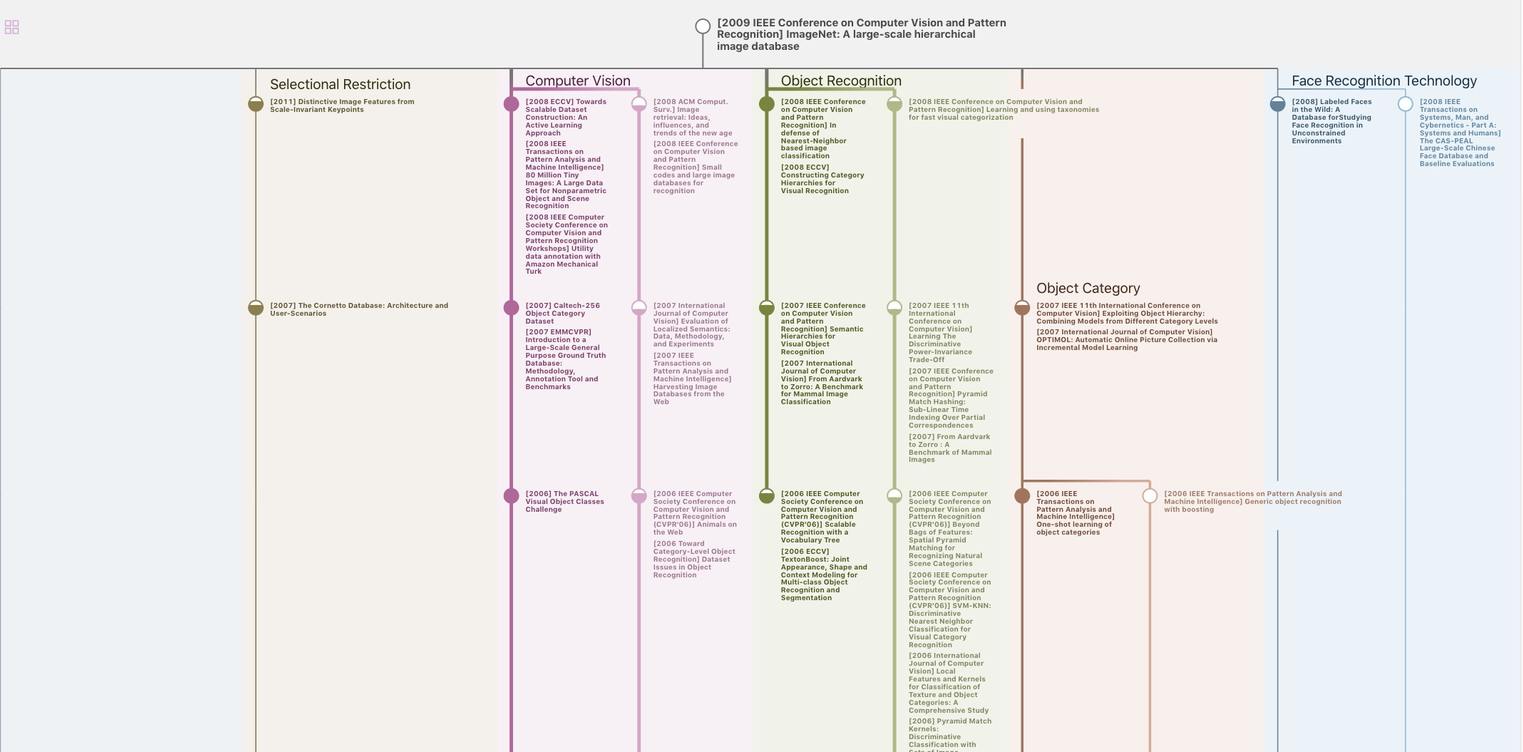
生成溯源树,研究论文发展脉络
Chat Paper
正在生成论文摘要