Superadditivity and Convex Optimization for Globally Optimal Cell Segmentation Using Deformable Shape Models
IEEE Transactions on Pattern Analysis and Machine Intelligence(2023)
摘要
Cell nuclei segmentation is challenging due to shape variation and closely clustered or partially overlapping objects. Most previous methods are not globally optimal, limited to elliptical models, or are computationally expensive. In this work, we introduce a globally optimal approach based on deformable shape models and global energy minimization for cell nuclei segmentation and cluster splitting. We propose an implicit parameterization of deformable shape models and show that it leads to a
convex
energy. Convex energy minimization yields the
global
solution independently of the initialization, is fast, and robust. To
jointly
perform cell nuclei segmentation and cluster splitting, we developed a novel iterative global energy minimization method, which leverages the inherent property of
superadditivity
of the convex energy. This property exploits the lower bound of the energy of the union of the models and improves the computational efficiency. Our method provably determines a solution close to global optimality. In addition, we derive a closed-form solution of the proposed global minimization based on the superadditivity property for non-clustered cell nuclei. We evaluated our method using fluorescence microscopy images of five different cell types comprising various challenges, and performed a quantitative comparison with previous methods. Our method achieved state-of-the-art or improved performance.
更多查看译文
关键词
Cell cluster splitting,global optimization,image models,object representation,segmentation,surface fitting
AI 理解论文
溯源树
样例
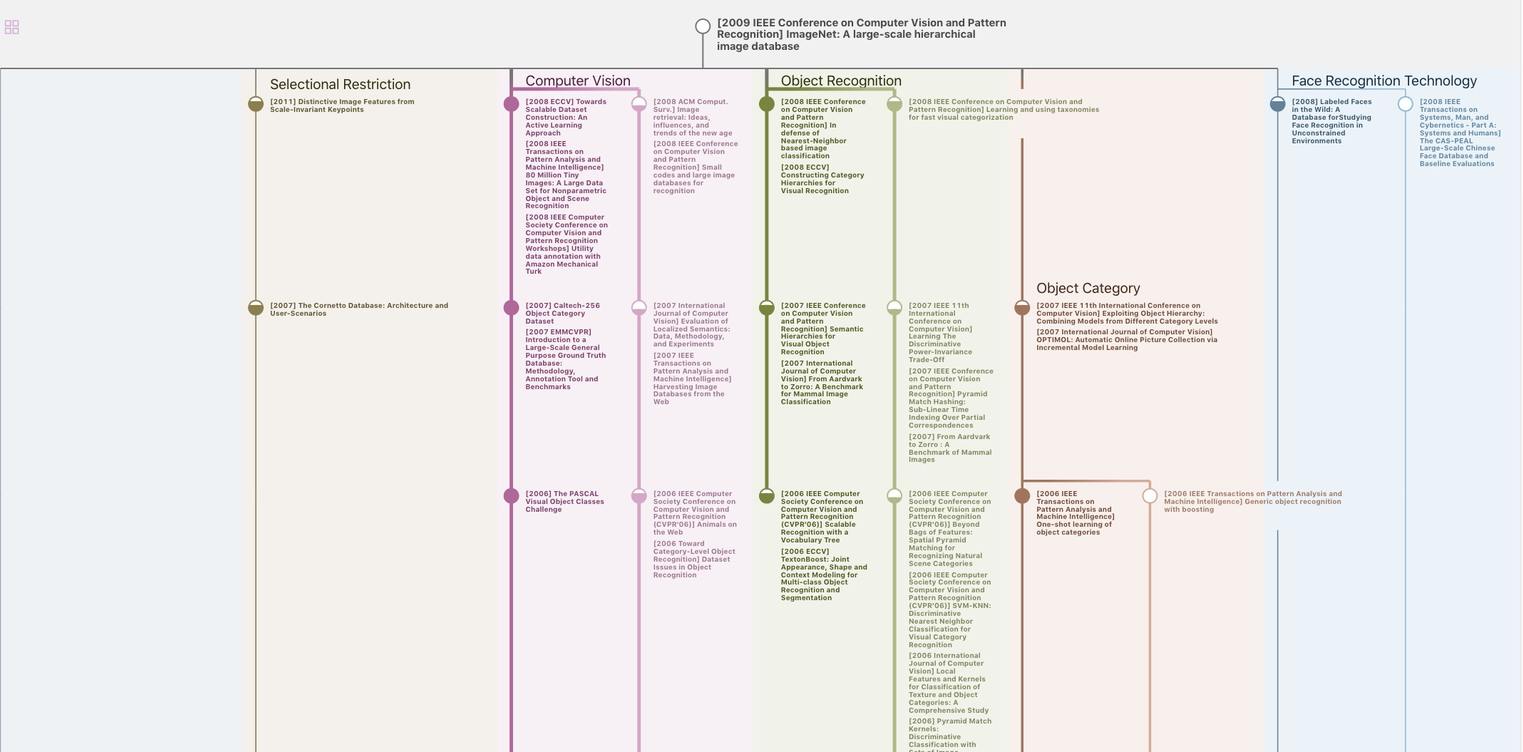
生成溯源树,研究论文发展脉络
Chat Paper
正在生成论文摘要