Profiling Olefins in Gasoline by Bromination Using GCxGC-TOFMS Followed by Discovery-Based Comparative Analysis
Analytical chemistry(2022)
摘要
An analytical workflow for the analysis of olefins in gasoline that combines selective bromination and comprehensive two-dimensional (2D) gas chromatography time-of-flight mass spectrometry (GCxGC-TOFMS) with discovery-based analysis is reported. First, a standard mix containing n-alkanes, 1-alkenes, and aromatic species was brominated and quantified using % reacted as a metric for each compound dass, defined as the difference in the total peak area between the brominated and original samples normalized to the original sample. The average % reacted (1 s.d.) values were -1.45% (2.8%) for the alkanes, 99.5% (0.4%) for the alkenes, and 6.7% (11.6%) for the aromatics, demonstrating excellent selectivity toward the alkenes with only minor aromatic bromination. The bromination chemistry was then applied to gasoline, followed by GCXGC-TOFMS analysis of the original and brominated gasoline. This GCxGC-TOFMS data set was then submitted to the supervised discovery tool tile-based F-ratio analysis (FRA), which reduced the large data set to only the chromatographic regions which distinguish between the original and brominated gasoline samples. FRA discovered 314 hits, 56 of which were determined statistically significant using combinatorial null distribution analysis (CNDA), a permutation-based significance test. Since the brominated olefins elute in an uncrowded region of the 2D chromatogram and have no signal in the original sample, their discoverability was greatly increased relative to the original olefins. By combining the information gained from brominated olefin standards and the structured patterns of the GCxGC separations, the top hits were identified as the dibromoalkane products of mono-olefins, with five C5 mono-olefins identified on a species level. The analytical workflow has broad implications for using selective reaction chemistries to facilitate supervised discovery by targeting desired compound classes.
更多查看译文
AI 理解论文
溯源树
样例
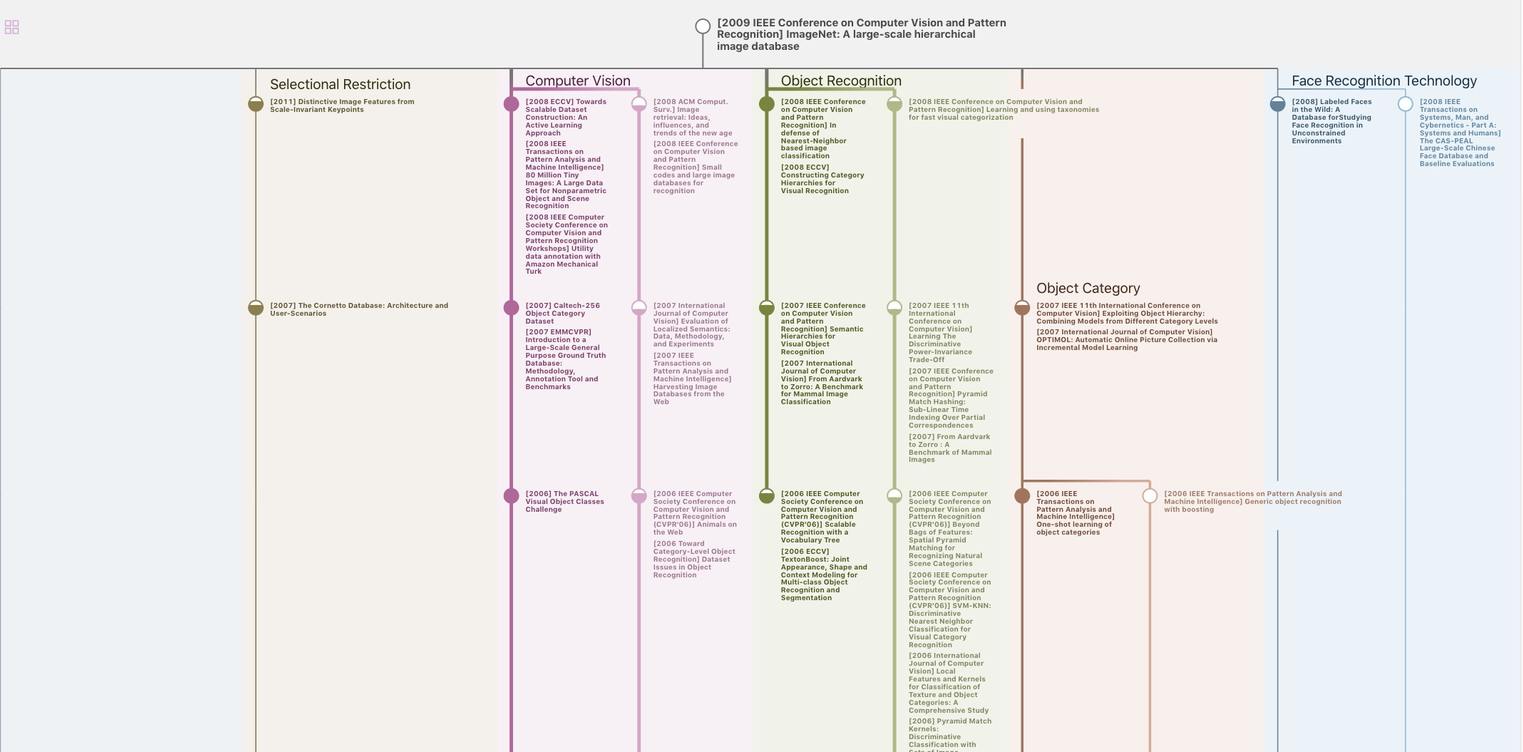
生成溯源树,研究论文发展脉络
Chat Paper
正在生成论文摘要