Efficient Range and kNN Twin Subsequence Search in Time Series
IEEE Transactions on Knowledge and Data Engineering(2023)
摘要
Analyzing time series data is crucial for many applications. In particular, subsequence search refers to finding subsequences within an input time series $T$ T that are similar to a query sequence $Q$ Q . Existing subsequence search approaches typically employ Euclidean distance or Dynamic Time Warping as similarity measures and address range queries. In this paper, we focus on Chebyshev distance, which is the largest difference between each individual pair of points across the entire length of two compared subsequences. We call such similar pairs twins . We first show how existing time series indices can be extended to perform twin subsequence search. Then, we introduce TS-Index, a novel index tailored to the computation of twin subsequence search queries. Moreover, given that specifying a distance threshold is often not straightforward, we show how TS-Index can also be used to evaluate $k$ k NN queries. Our extensive experimental evaluation compares these approaches using real time series datasets. The results demonstrate that TS-Index can retrieve twin subsequences faster than all other methods under various conditions.
更多查看译文
AI 理解论文
溯源树
样例
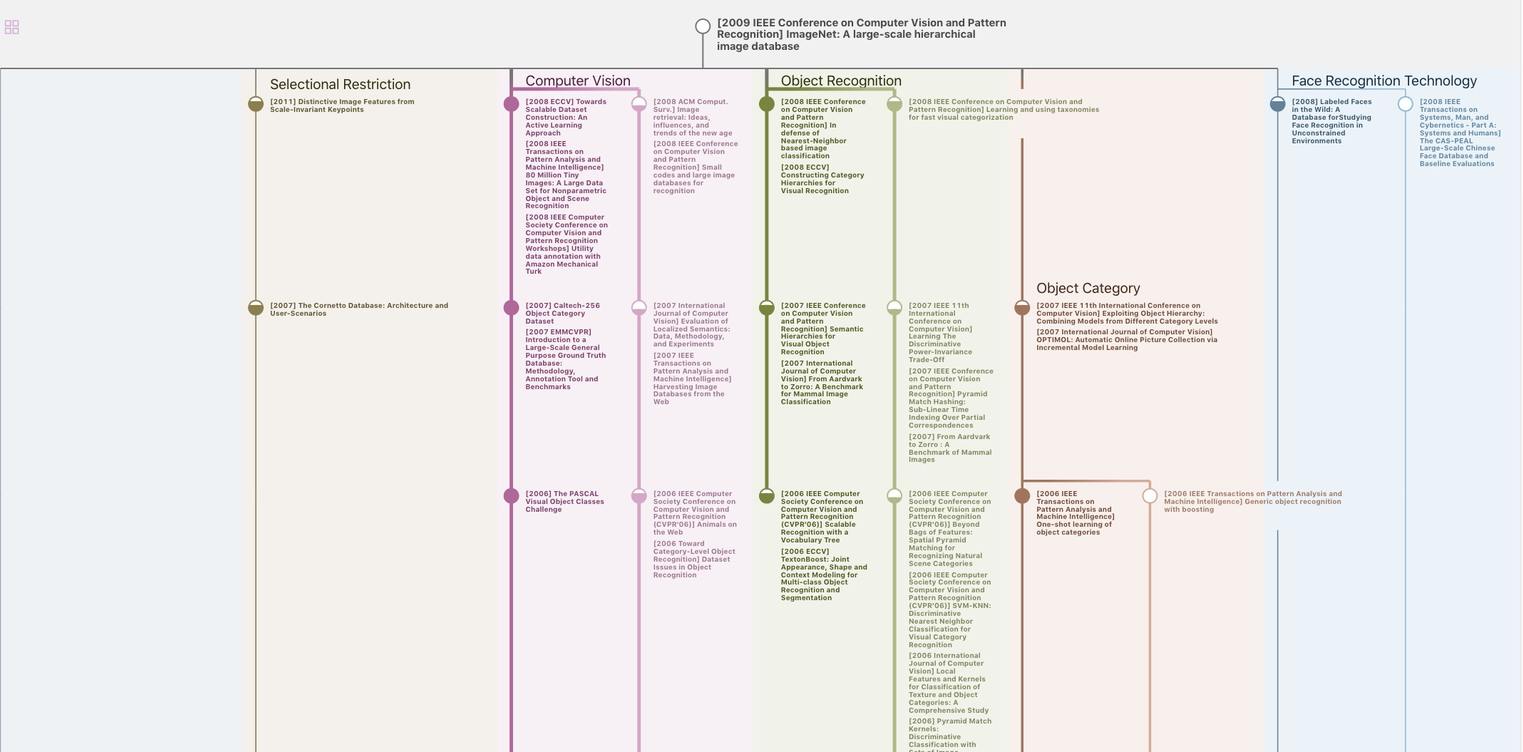
生成溯源树,研究论文发展脉络
Chat Paper
正在生成论文摘要