EAD-Conformer: a Conformer-Based Encoder-Attention-Decoder-Network for Multi-Task Audio Source Separation
ICASSP 2022 - 2022 IEEE International Conference on Acoustics, Speech and Signal Processing (ICASSP)(2022)
摘要
In this paper, we propose a Conformer-based network to improve the performance of multi-task audio source separation. This network, named EAD-Conformer, employs Conformer blocks to capture both local and global information, and an encoder-attention-decoder manner encourages the network to perform attentive modeling based on different sources. Specifically, EAD-Conformer first parses out the feature representations from the mixture by a Conformer-based encoder. Then, an attention module extracts selective information for each track and bridges encoder and decoders. Finally, three decoders respectively process attentive features and generate output masks for different sources. In addition, the proposed discriminate loss further enlarges the distance between different sources. Experiments demonstrate the effectiveness of EAD-Conformer, which achieves 13.37 dB, 11.41 dB, 10.56 dB signal-to-distortion ratio improvement on speech, music, noise track, respectively, and shows advantages over several well-known models.
更多查看译文
关键词
Multi-task Audio Source Separation,EAD-Conformer,Discriminate Loss
AI 理解论文
溯源树
样例
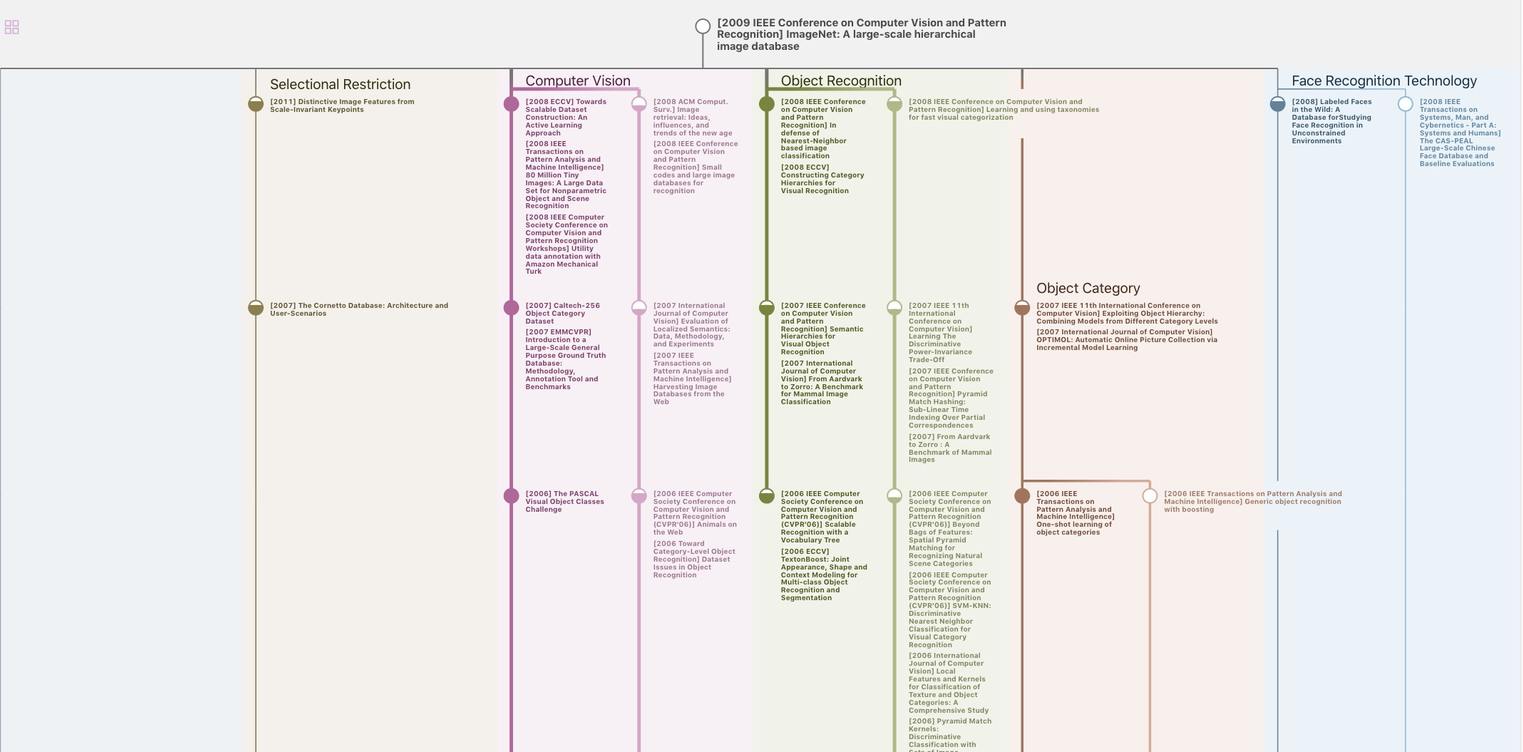
生成溯源树,研究论文发展脉络
Chat Paper
正在生成论文摘要