Effect of conductance linearity of Ag-chalcogenide CBRAM synaptic devices on the pattern recognition accuracy of an analog neural training accelerator
Neuromorphic computing and engineering(2022)
摘要
Abstract Pattern recognition using Deep Neural Networks (DNN) has been implemented using Resistive RAM (RRAM) devices. To achieve high classification accuracy in pattern recognition with DNN systems, a linear, symmetric weight update, as well as multi-level conductance (MLC) behavior of the analog synapse is required. Ag-Chalcogenide-based Conductive Bridge RAM (CBRAM) devices have demonstrated multiple resistive states making them potential candidates for use as analog synapses in neuromorphic hardware. In this work, we analyze the conductance linearity response of these devices to different pulsing schemes. We have demonstrated an improved linear response of the devices from a non-linearity factor of 6.65 to 1 for potentiation and -2.25 to -0.95 for depression with non-identical pulse application. The effect of improved linearity was quantified by simulating the devices in an artificial neural network. The classification accuracy of the 2-layer neural network was seen to be improved from 85% to 92% for the small digit MNIST dataset.
更多查看译文
AI 理解论文
溯源树
样例
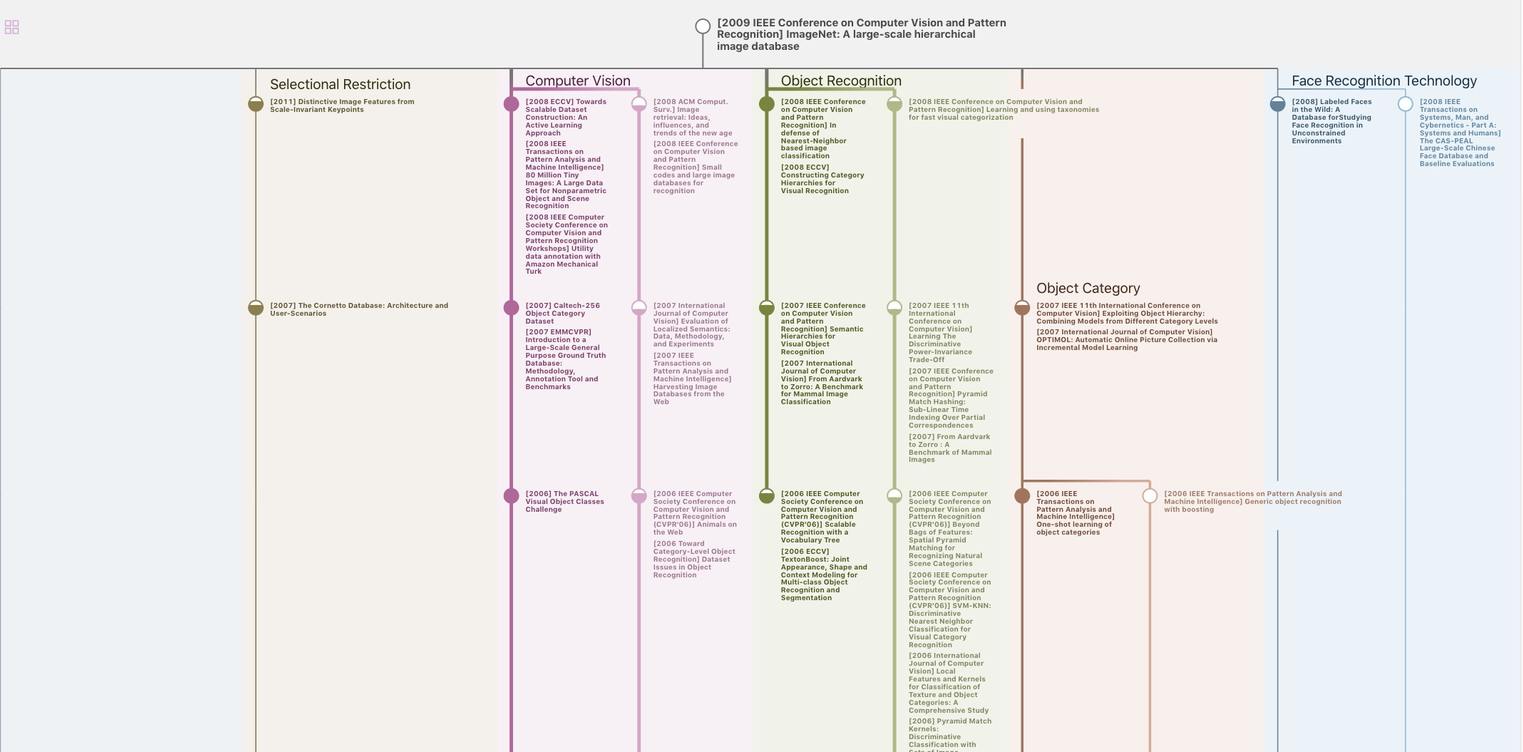
生成溯源树,研究论文发展脉络
Chat Paper
正在生成论文摘要