Fuzzy Differential Privacy Theory and Its Applications in Subgraph Counting
IEEE Transactions on Fuzzy Systems(2023)
摘要
Transportation networks are essential to the operation of societies and economies. Protecting the privacy of sensitive information is a meaningful conception in sustainable transport when mining the transportation data. In data mining, differential privacy (DP) has provable privacy guarantees for releasing sensitive data by introducing randomness into query results. However, it suffers from significant accuracy loss of outputs when the query has high sensitivity (e.g., triangle counting). The reason is that the range of random perturbation to each query result in DP is too large. It consists of all possible output values for a query that forms a large or even unbounded interval. However, when impose perturbation only in a small neighborhood of the true query result, the similarity measure based on randomness in DP fails. Thereupon, we introduce fuzziness into DP to formulate new models which have smaller disturbance via fuzzy similarity measures. In this article, we establish a novel and general theory of private data analysis, fuzzy differential privacy (FDP). The new theory FDP aims to acquire a more flexible tradeoff between the accuracy of outputs and the privacy-preserving level of data. FDP combines DP with fuzzy set theory by introducing fuzziness into the query results and characterizing similarities between outputs via multiple fuzzy similarity measures. From this perspective, DP can be viewed as a special case of FDP with probabilistic similarity measure. Compared with DP, FDP has three superiorities: 1) most fuzzy similarity measures in FDP support sliding window perturbation strategies we proposed, which refer to perturbation in a small neighborhood of the query results; 2) FDP adds noise to the query results only according to a fraction of all possible neighboring datasets; and 3) the fuzzy similarity with valued in [0,1] quantifies the privacy protection level intuitively. These three points enable more accurate outputs while providing provable and intuitive privacy guarantees. As for subgraph counting, the state-of-the-art method is ladder framework in DP. We illustrate FDP mechanisms by applying them to a common application in subgraph counting–triangle/4-cliques counting. Experiments show that FDP is effective and efficient with smaller output errors than DP.
更多查看译文
关键词
Differential privacy (DP),fuzzy algorithms,fuzzy similarity,privacy protection,subgraph counting
AI 理解论文
溯源树
样例
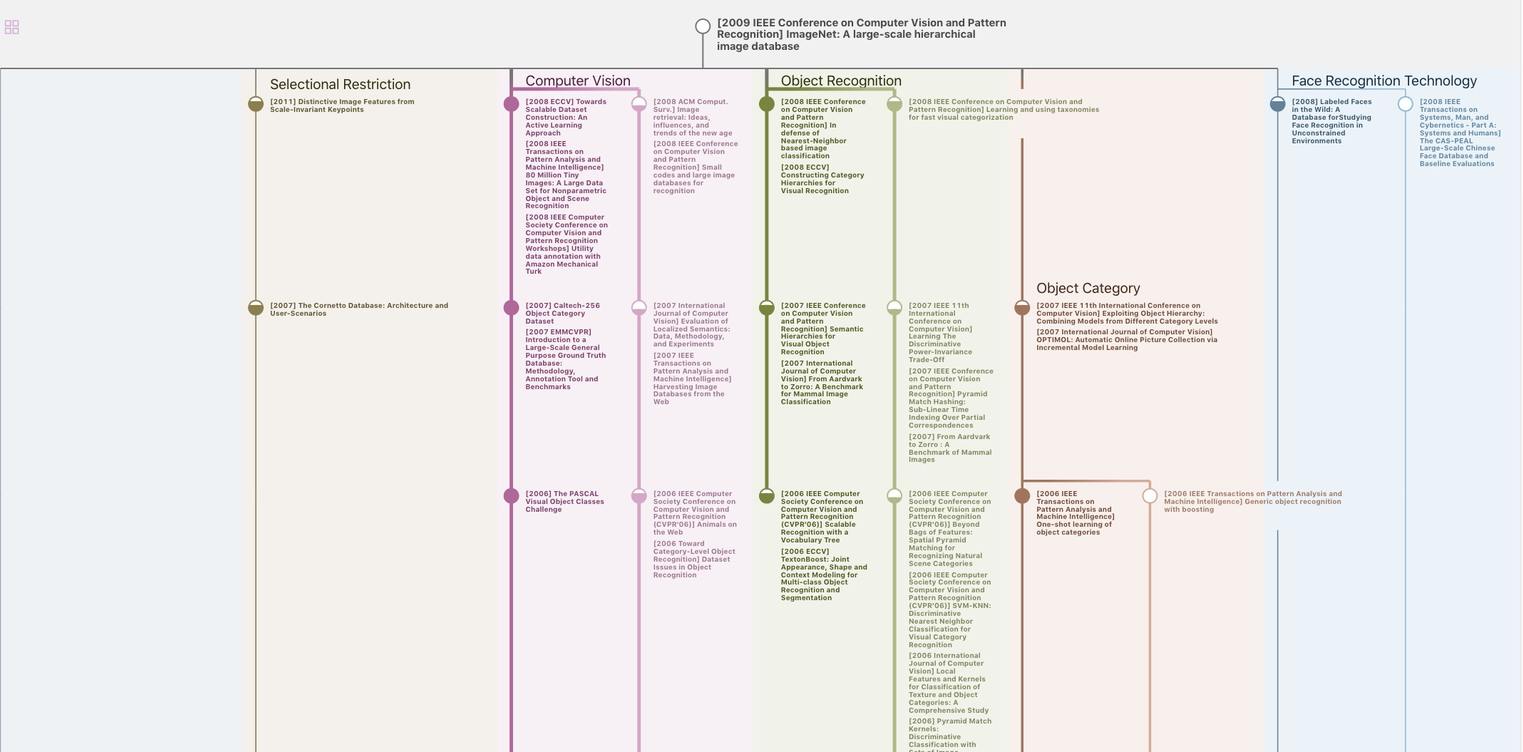
生成溯源树,研究论文发展脉络
Chat Paper
正在生成论文摘要