Stability selection for mixed effect models with large numbers of predictor variables: A simulation study
Social Science Research Network(2022)
摘要
Covariate selection when the number of available variables is large relative to the number of observations is problematic in epidemiology and remains the focus of continued research. Whilst a variety of statistical methods have been developed to attempt to overcome this issue, at present very few methods are available for wide data that include a clustered outcome. The purpose of this research was to make an empirical evaluation of a new method for covariate selection in wide data settings when the dependent variable is clustered. We used 3300 simulated datasets with a variety of defined structures and known sets of true predictor variables to conduct an empirical evaluation of a mixed model stability selection procedure. Comparison was made with an alternative method based on regularisation using the least absolute shrinkage and selection operator (Lasso) penalty. Model performance was assessed using several metrics including the true positive rate (proportion of true covariates selected in a final model) and false discovery rate (proportion of variables selected in a final model that were non-true (false) variables). For stability selection, the false discovery rate was consistently low, generally remaining ≤ 0.02 indicating that on average fewer than 1 in 50 of the variables selected in a final model were false variables. This was in contrast to the Lasso-based method in which the false discovery rate was between 0.59 and 0.72, indicating that generally more than 60% of variables selected in a final model were false variables. In contrast however, the Lasso method attained higher true positive rates than stability selection, although both methods achieved good results. For the Lasso method, true positive rates remained ≥ 0.93 whereas for stability selection the true positive rate was 0.73–0.97. Our results suggest both methods may be of value for covariate selection with high dimensional data with a clustered outcome. When high specificity is needed for identification of true covariates, stability selection appeared to offer the better solution, although with a slight loss of sensitivity. Conversely when high sensitivity is needed, the Lasso approach may be useful, even if accompanied by a substantial loss of specificity. Overall, the results indicated the loss of sensitivity when employing stability selection is relatively small compared to the loss of specificity when using the Lasso and therefore stability selection may provide the better option for the analyst when evaluating data of this type.
更多查看译文
关键词
Covariate selection,Mixed models,Selection stability,High dimensional clustered data,Permutation methods,False discovery rate
AI 理解论文
溯源树
样例
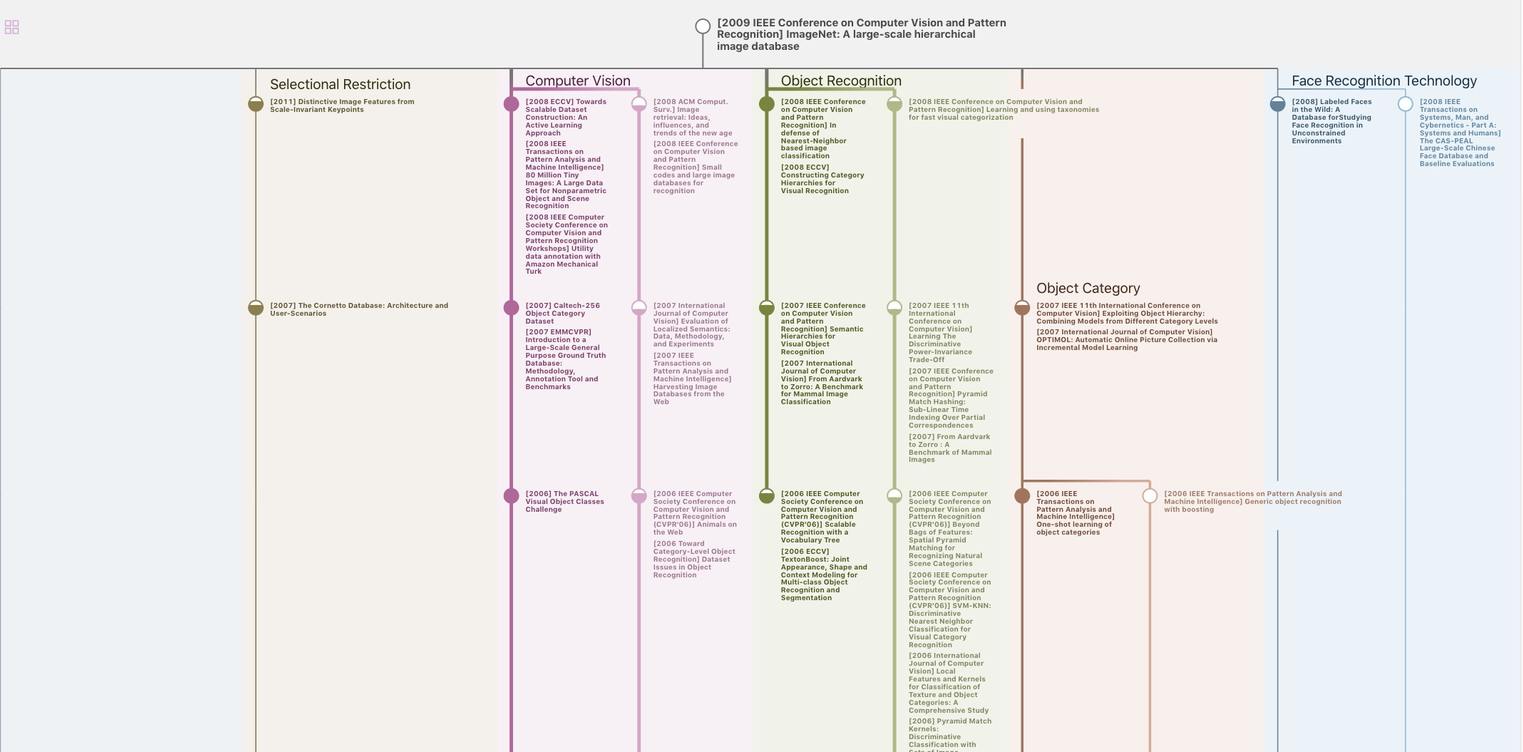
生成溯源树,研究论文发展脉络
Chat Paper
正在生成论文摘要