Standardisation and Data Augmentation in Genetic Programming
IEEE Transactions on Evolutionary Computation(2022)
摘要
Genetic programming (GP) is a common method for performing symbolic regression that relies on the use of ephemeral random constants in order to adequately scale predictions. Suitable values for these constants must be drawn from appropriate, but typically unknown, distributions for the problem being modelled. While rarely used with GP, Z-score standardisation of feature and response spaces often significantly improves the predictive performance of GP by removing scale issues and reducing error due to bias. However, in some cases it is also associated with erratic error due to variance. This paper demonstrates that this variance component increases in the presence of gaps at the boundaries of the training data explanatory variable intervals. An initial solution to this problem is proposed that augments training data with pseudo instances located at the boundaries of the intervals. When applied to benchmark problems, particularly with small training samples, this solution reduces error due to variance and therefore total error. Augmentation is shown to also stabilise error in larger problems, however results suggest that standardised GP works well on such problems with little need for training data augmentation.
更多查看译文
关键词
Bias-variance decomposition,data augmentation,evolutionary machine learning,feature scaling,genetic programming (GP),prediction error,standardization,symbolic regression
AI 理解论文
溯源树
样例
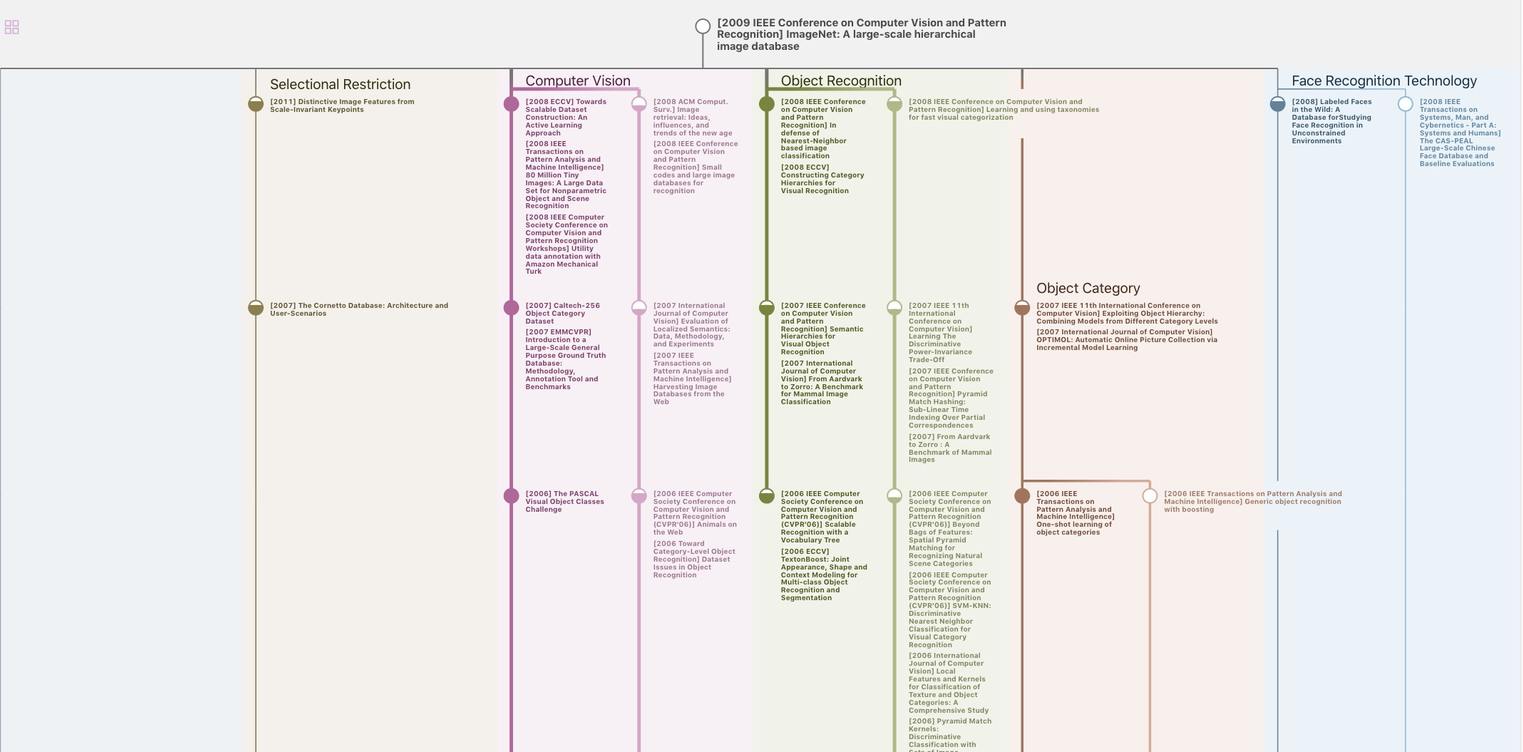
生成溯源树,研究论文发展脉络
Chat Paper
正在生成论文摘要