Auto-weighted sample-level fusion with anchors for incomplete multi-view clustering
Pattern Recognition(2022)
摘要
Aiming at solving the problem of clustering in the multi-view datasets which include samples with information missing in one or more views, incomplete multi-view clustering has received considerable attention. However, most studies can not get satisfying accuracy and efficiency when dealing with datasets in which a considerable number of instances are missing in partial views. To address this problem, a method named Auto-weighted Sample-level Fusion with Anchors for Incomplete Multi-view Clustering (ASA-IC) is proposed in this paper. It designs an auto-weighted sample-level fusion strategy, which realizes the optimized conversion from the individual instance-to-anchor similarity learning to the concensus instance-to-anchor similarity matrix construction. ASA-IC can not only handle incomplete samples and effectively explore the relationship between each instance and anchors, but also deal with various incomplete clustering situations and be applied in large-scale datasets as well. Besides, experiments on 5 complete datasets and 27 incomplete ones illustrate its effectiveness quantitatively and qualitatively. (c) 2022 Elsevier Ltd. All rights reserved.
更多查看译文
关键词
Incomplete data,Multi-view clustering,Anchor,Auto-weighted,Large-scale
AI 理解论文
溯源树
样例
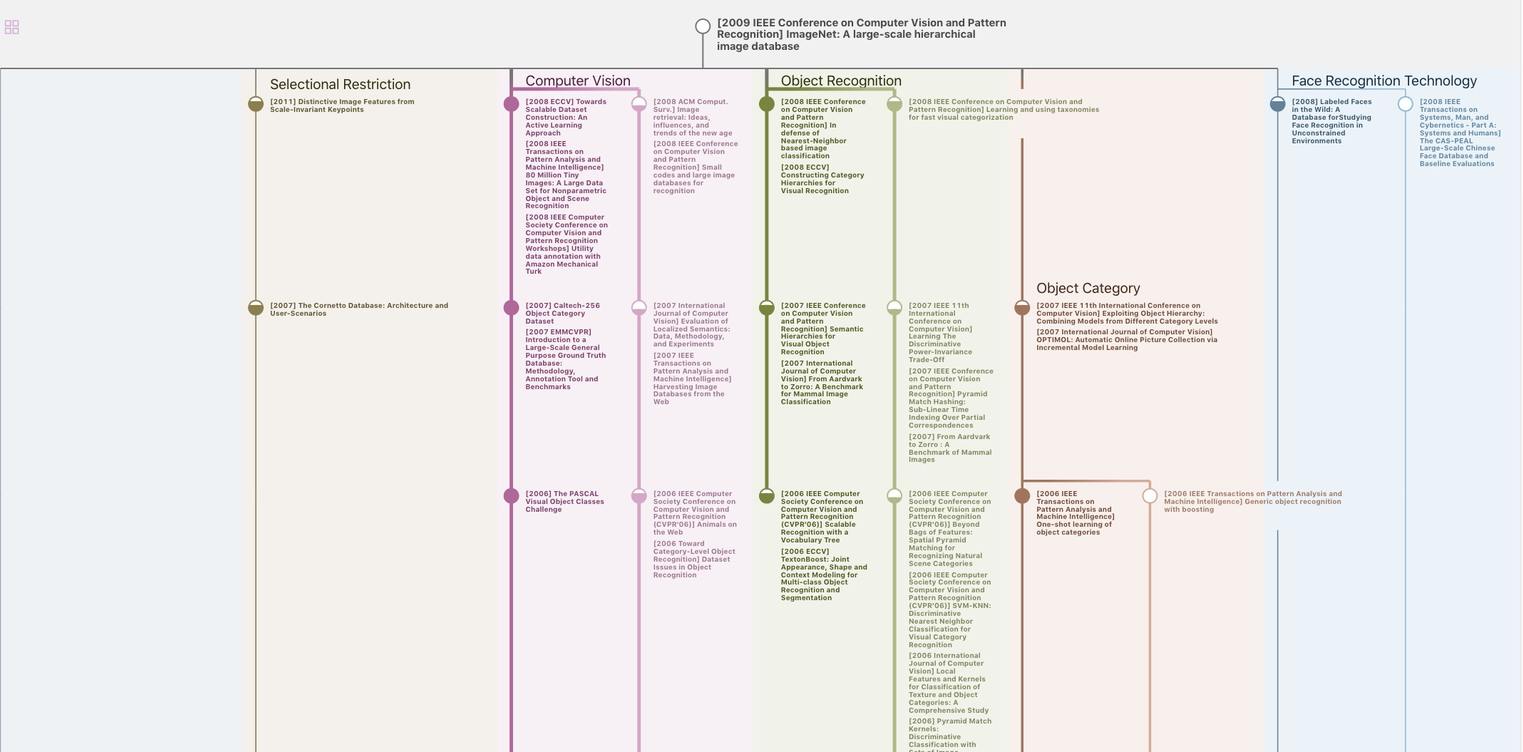
生成溯源树,研究论文发展脉络
Chat Paper
正在生成论文摘要