An infinite hidden Markov model with stochastic volatility
JOURNAL OF FORECASTING(2024)
摘要
This paper extends the Bayesian semiparametric stochastic volatility (SV-DPM) model. Instead of using a Dirichlet process mixture (DPM) to model return innovations, we use an infinite hidden Markov model (IHMM). This allows for time variation in the return density beyond that attributed to parametric latent volatility. The new model nests several special cases as well as the SV-DPM. We also discuss posterior and predictive density simulation methods for the model. Applied to equity returns, foreign exchange rates, oil price growth and industrial production growth, the new model improves density forecasts, compared with the SV-DPM, a stochastic volatility with Student's t$$ t $$ innovations and other fat-tailed volatility models.
更多查看译文
关键词
Bayesian,Markov-switching,MCMC,nonparametric,semiparametric,stochastic volatility
AI 理解论文
溯源树
样例
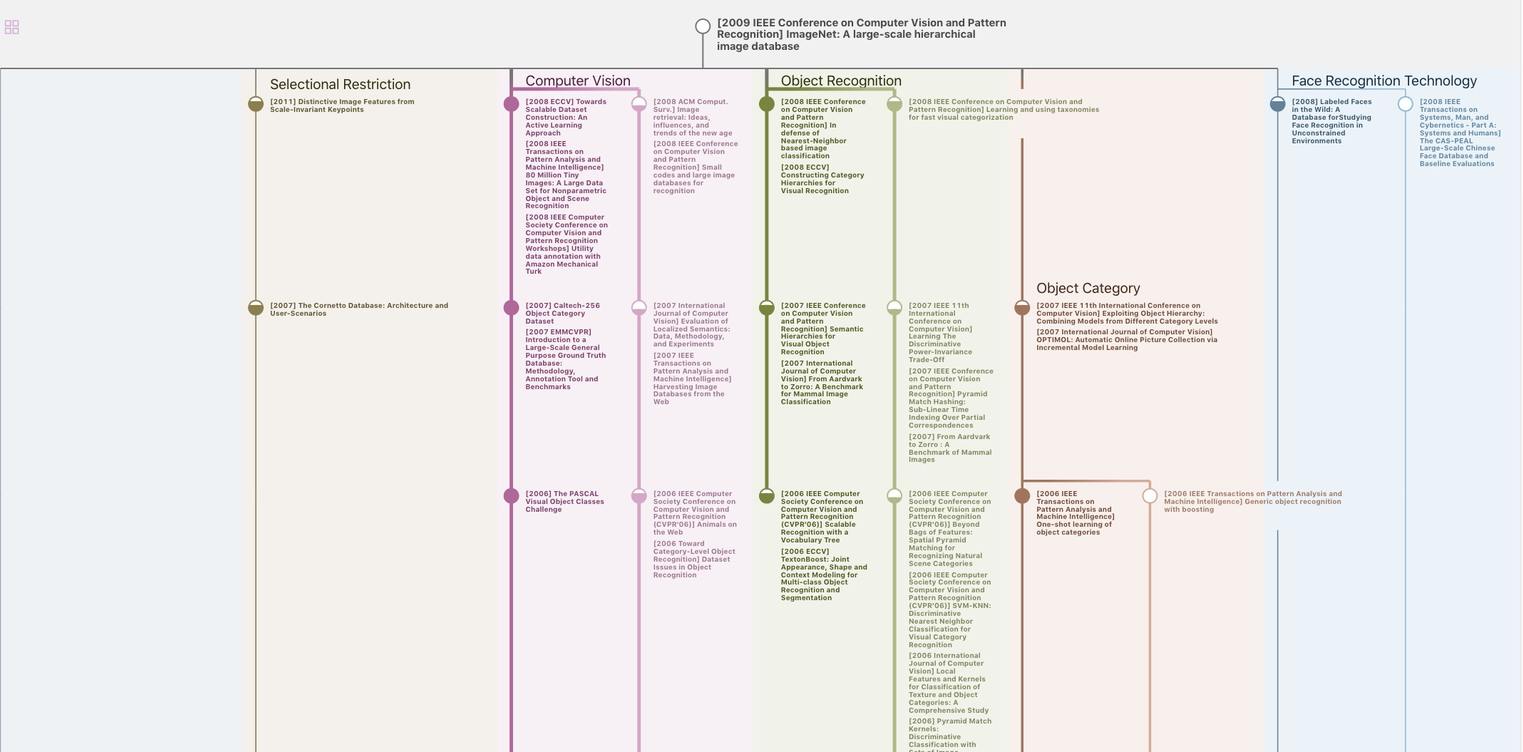
生成溯源树,研究论文发展脉络
Chat Paper
正在生成论文摘要