Hardware Neural Network using Hybrid Synapses via Transfer Learning: WOx Nano-Resistors and TiOx RRAM Synapse for Energy-Efficient Edge-AI Sensor
2021 IEEE International Electron Devices Meeting (IEDM)(2021)
摘要
We present a neural network (NN) based intelligent sensor for biomedical applications on edge using nano-electronic synapses. The proposed NN for the on-device AI comprises newly developed WOx resistors and TiO x based resistive switching memories (RRAMs). A highly resistive WOx linear resistor is engineered to construct a low power, high density pre-trained NN. Also, TiOx RRAM shows multi-level programmable states with a large memory window (> x1000), which customizes the Edge device to a new subject with only partial learning of NN. We experimentally evaluate 1k-bit WOx resistor arrays and 8-inch wafer-scale TiOx RRAM chips to verify the high accuracy of the ECG sensor. By using experimental ECG data as well as the Physiobank dataset, we have confirmed high classification accuracy (∼ 98%) at the edge even with a limited training data (only 20 beats) and parameters (5.4%) of entire system.
更多查看译文
关键词
nanoelectronic synapses,WOx resistors,multilevel programmable states,memory window,edge device,partial learning,wafer-scale TiOx RRAM chips,ECG sensor,experimental ECG data,hardware neural network,hybrid synapses,transfer learning,WOx nanoresistors,TiOx RRAM synapse,energy-efficient Edge-AI sensor,intelligent sensor,biomedical applications,WOx resistor arrays,resistive WOx linear resistor,TiOx-based resistive switching memories,energy-efficient edge-AI S ensor
AI 理解论文
溯源树
样例
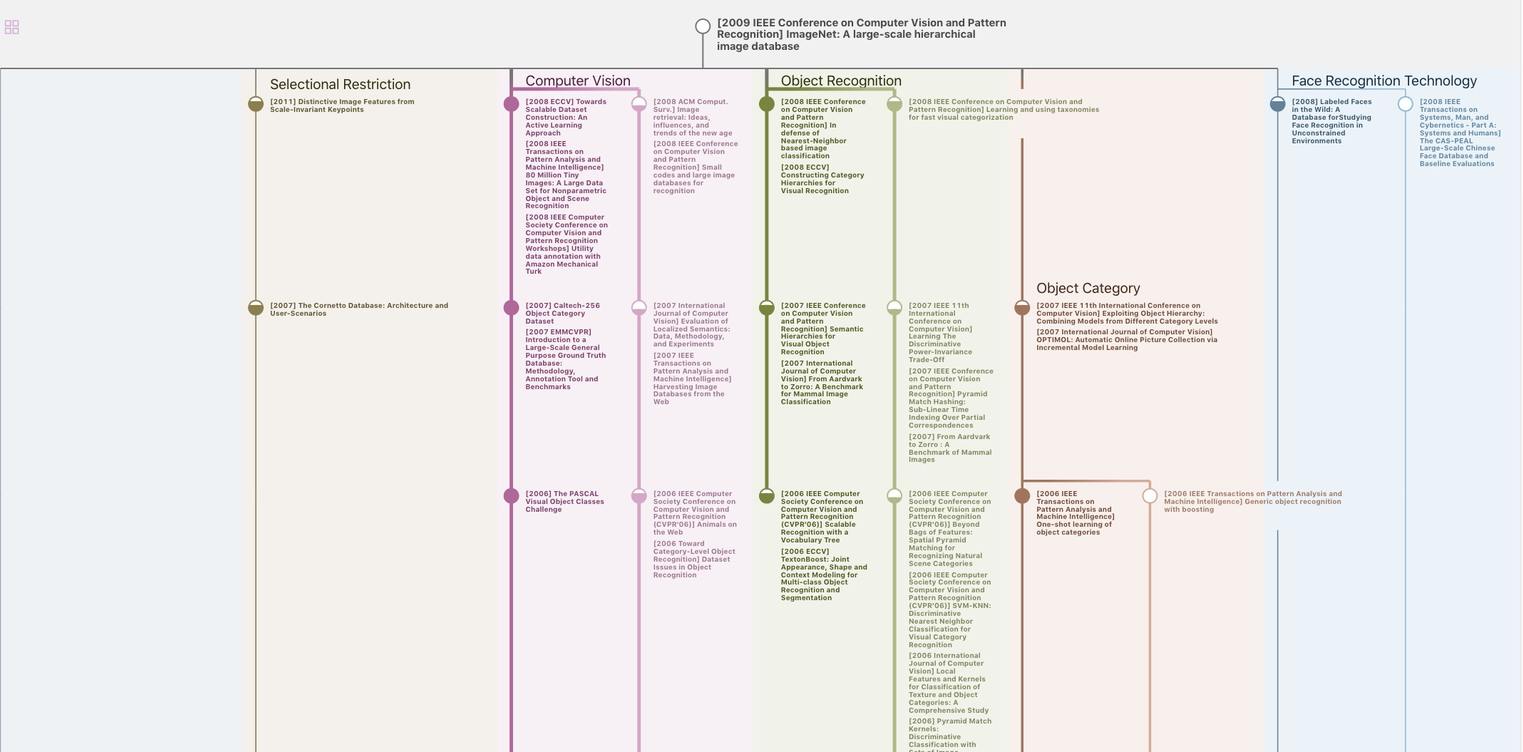
生成溯源树,研究论文发展脉络
Chat Paper
正在生成论文摘要