Retrospective quality by design r(QbD) for lactose production using historical process data and design of experiments
COMPUTERS IN INDUSTRY(2022)
摘要
Quality by Design (QbD) is a popular formal approach for designing, upscaling and optimizing industrial production facilities towards guaranteed quality. To avoid the many costly experiments required for QbD, historical production data may be exploited for optimization instead in what is known as a retrospective QbD (rQbD) study. Current rQbD literature does limitedly discuss data-driven identification of Critical Process Parameters (CPPs) to optimize limited process knowledge availability, and does not cover situations where technical operation limits have not yet been fully explored and/or where parallel equipment (lines) are used. This work presents a new rQbD strategy that addresses these challenges by balancing knowledge that can be obtained from statistical analysis of historical data, together with process experts with a carefully designed set of plant-scale experiments within current operational limits. This novel strategy is demonstrated on a long-running industrial lactose production facility. By digitally and experimentally exploring historical operation variability, we found new operational regimes for this production that may lead to up to 7% product quality improvement, reduced energy consumption and increased process under-standing. Although optimizing a specific process by necessity requires a process-specific approach, the way in which we systematically optimize the current process with Hybrid AI (combining available knowledge with new insights from historical data) shows that approaches that are currently used in prospective process upscaling may be modified to be invaluable for optimization of full-scale processes with a long operational history. (C) 2022 The Authors. Published by Elsevier B.V. CC_BY_4.0
更多查看译文
AI 理解论文
溯源树
样例
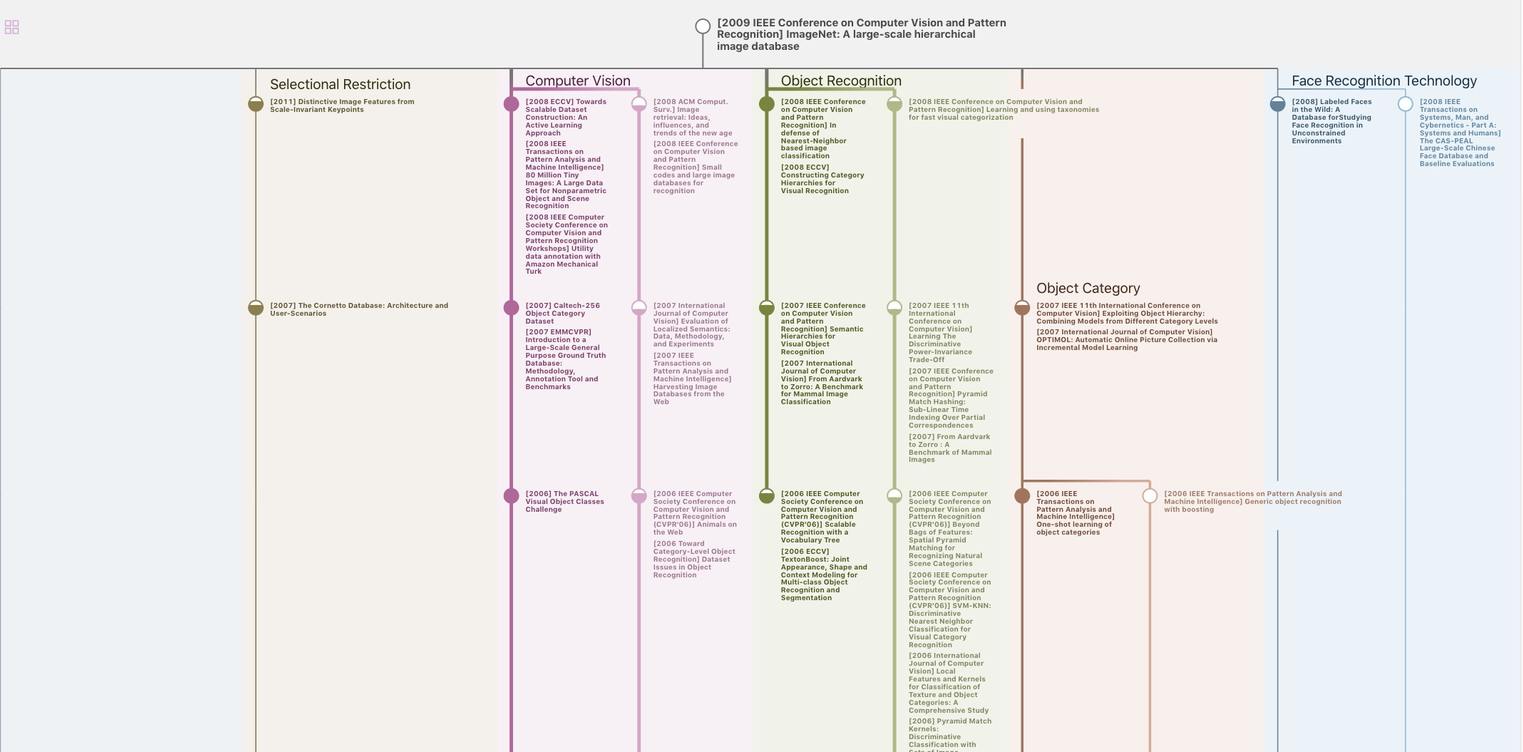
生成溯源树,研究论文发展脉络
Chat Paper
正在生成论文摘要