Learning Invariant Representation Using Synthetic Imagery for Object Detection
Social Science Research Network(2022)
摘要
Recent years have witnessed a rapid advance in training and testing synthetic data through deep learning networks for the annotation of synthetic data can be automatically marked. However, domain discrepancy still exists between synthetic data and real data. In this paper, we address the domain discrepancy issue from three aspects: 1) We design a synthetic image generator with automatically labeled based on 3d scenes. 2) A novel adversarial domain adaptation model is proposed to learn robust intermediate representation free of distractors to improve the transfer performance. 3) We construct a distractor-invariant network and adopt the sample transferability strategy on global-local levels respectively to mitigate the cross-domain gap. Additional exploratory experiments demonstrate that the proposed model achieves large performance margins, which show significant advance over the other state-of-the-art models, performing a promotion of 10%-15% mAP on various domain adaptation scenarios.
更多查看译文
关键词
Invariant representation,distractor-invariant,synthetic data,feature alignment,domain discriminator
AI 理解论文
溯源树
样例
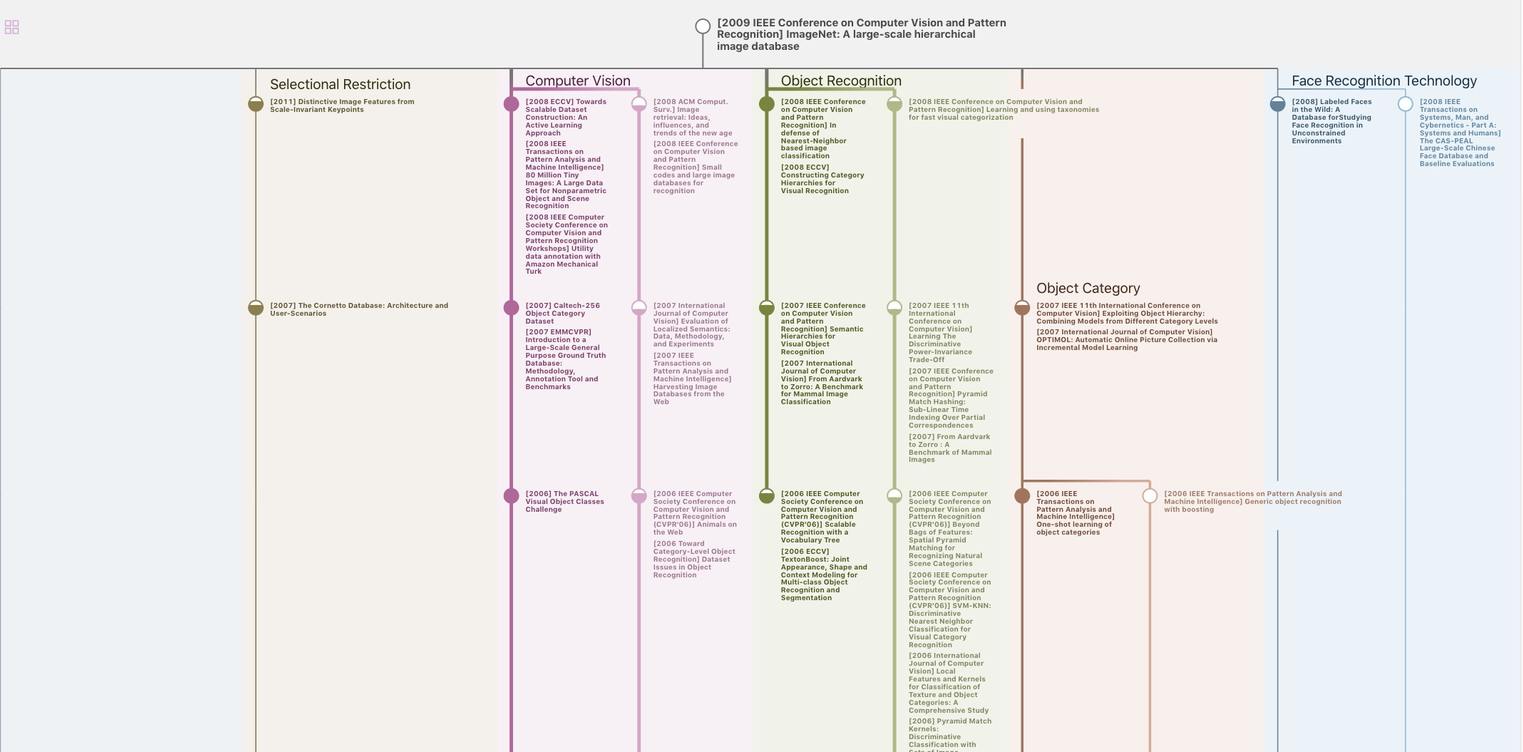
生成溯源树,研究论文发展脉络
Chat Paper
正在生成论文摘要