Deep Learning-Based Classi Fi Cation of Cancer Cell in Leptomeningeal Metastasis on Cytomorphologic Features of CSF
Social Science Research Network(2021)
摘要
Background: Difficulty diagnoses of leptomeningeal metastasis and lack of typical symptoms are considerable challenges in the clinical. As CSF a gold standard in LM, it is necessary to establish a deep learning model to classify cancer cells in CSF to facility doctor early diagnosis.
Method: CNN models based on ResNet-Inception-V2 or ResNet-50 are used to training cells in CSF, which is evaluated by average precision or accuracy. Besides, our research comprised the interpretation of 42 doctors with different experience and CNN in the human-machine test to validate CNN's performance.
Results: The precision of cancer cells was over 95% in the CNN1 model. In the human-machine test, the cell classification model's(CNN1) accuracy was close to experts and higher than other doctors. The cancer cell classification model's accuracy (CNN2) is 10% higher than that of experts, and the time-consuming is only 1/3 of that of an expert. We successfully achieved cell training in the small cell dataset in an 8-way cell classification model(CNN3), which overall accuracy is 90%.
Explanation: Deep learning can be widely used cerebrospinal fluid cytology pictures for classification. For difficult cancer classify task, CNN accuracy is higher than that of specialist doctors, and its performance is better than that of junior doctors and intern.
Funding: The project described was supported by National research and development precision medicine (Program 2016YFC0904500), National Science and Technology Gold Project (81671185), Natural Science Basic Research Program of Shaanxi (Program No. 2019JQ-251), Hospital-level project of Xi'an International Medical Center (Program No. 2020ZD007).
Declaration of Interest: The authors declare that they have no conflicts of interests.
Ethical Approval: The study was conducted in accordance with Declaration of Helsinki and approved by the Institutional Review Boards at Xijing Hospital.
更多查看译文
AI 理解论文
溯源树
样例
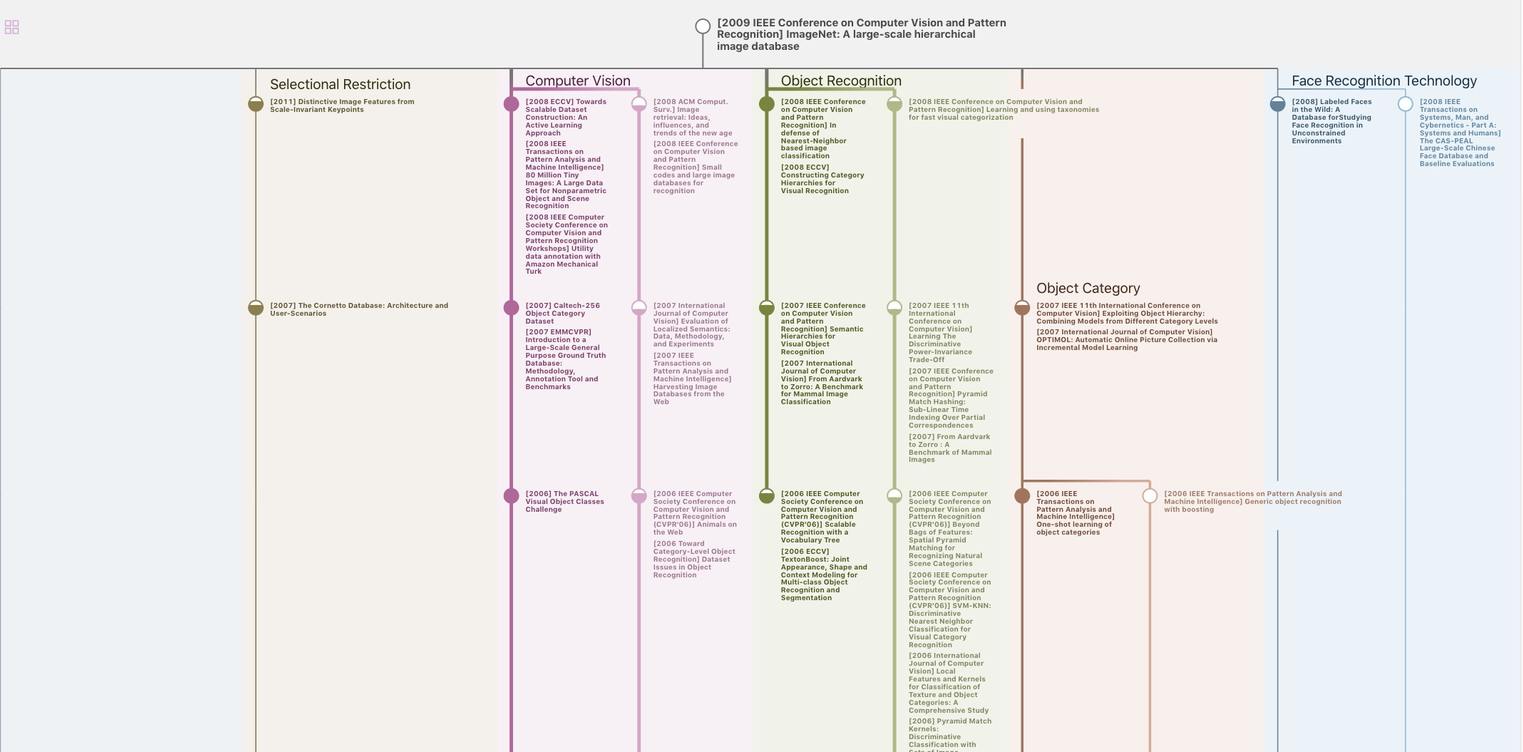
生成溯源树,研究论文发展脉络
Chat Paper
正在生成论文摘要