A competitive Markov decision process model and a recursive reinforcement-learning algorithm for fairness scheduling of agile satellites.
Comput. Ind. Eng.(2022)
摘要
• We proposed an image quality representation method for fairness measurement. • A finite competitive Markov decision process model is constructed. • An algorithm based on reinforcement learning with block coding is proposed. With the development of space science and technology, the requirements of stereo imaging tasks are becoming ever more urgent in many space applications, such as 3-D building changes, natural disaster assessment, and target positioning. As the new generation of three degrees freedom(roll, pitch, and yaw)satellites, agile earth observation satellites have a wider variable-roll visible scope and longer variable-pitch visible time for ground targets, thus they are suitable for stereo tasks. However, current task scheduling models and algorithms ignore the imaging quality and fairness of resource sharing by pitch and roll. This paper investigated a new scheduling model and algorithm to realize the satellite fairness scheduling for stereo tasks. First, a novel image quality representation method is proposed to measure the fairness of task observation. Second, a finite Markov decision process model that considers flexible pitch and roll capabilities is designed. Finally, an algorithm based on the reinforcement learning technique with block coding is proposed to solve fairness scheduling problems. We apply the proposed scheduling technique to several different problem instances, the computation results verify that our approach is effective for obtaining optimum solutions and relative fairness solutions.
更多查看译文
关键词
Fair schedule,Stereo image,Multi-agile satellite scheduling,Reinforcement learning
AI 理解论文
溯源树
样例
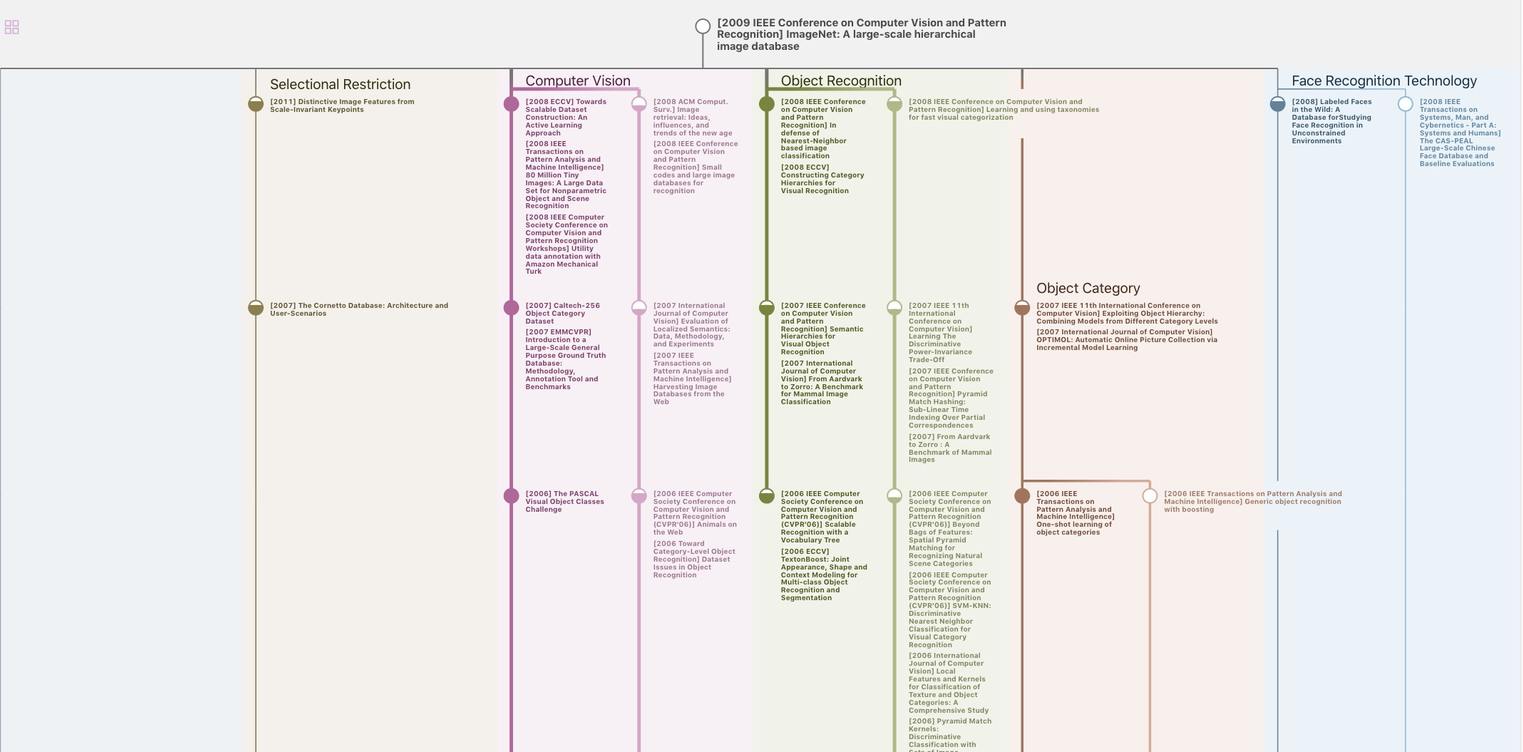
生成溯源树,研究论文发展脉络
Chat Paper
正在生成论文摘要