Generating and Adapting to Diverse Ad-Hoc Partners in Hanabi
IEEE transactions on games(2022)
摘要
Hanabi is a cooperative game that brings the problem of modeling other players to the forefront. In this game, coordinated groups of players can leverage pre-established conventions to great effect. In this paper, we focus on ad-hoc settings with no previous coordination between partners. We introduce a ‘`Bayesian Meta-Agent’' that maintains a belief distribution over hypotheses of partner policies. The policies that serve as initial hypotheses are generated using MAP-Elites, to ensure behavioral diversity. We evaluate an ‘`Adaptive’' version of the agent, which selects a response policy based on the updated belief distribution and a ‘`Generalist’' version, which selects a response based on the uniform prior. In short episodes of 10 games with a consistent partner, the ‘`Adaptive’' version outperforms the ‘`Generalist’' when the training and evaluation populations are the same. This presents a first step towards an agent that can model its partner and adapt within a time frame that is compatible with human interaction.
更多查看译文
关键词
Learning (artificial intelligence), Naive Bayes methods, Computational and artificial intelligence, Evolutionary computation
AI 理解论文
溯源树
样例
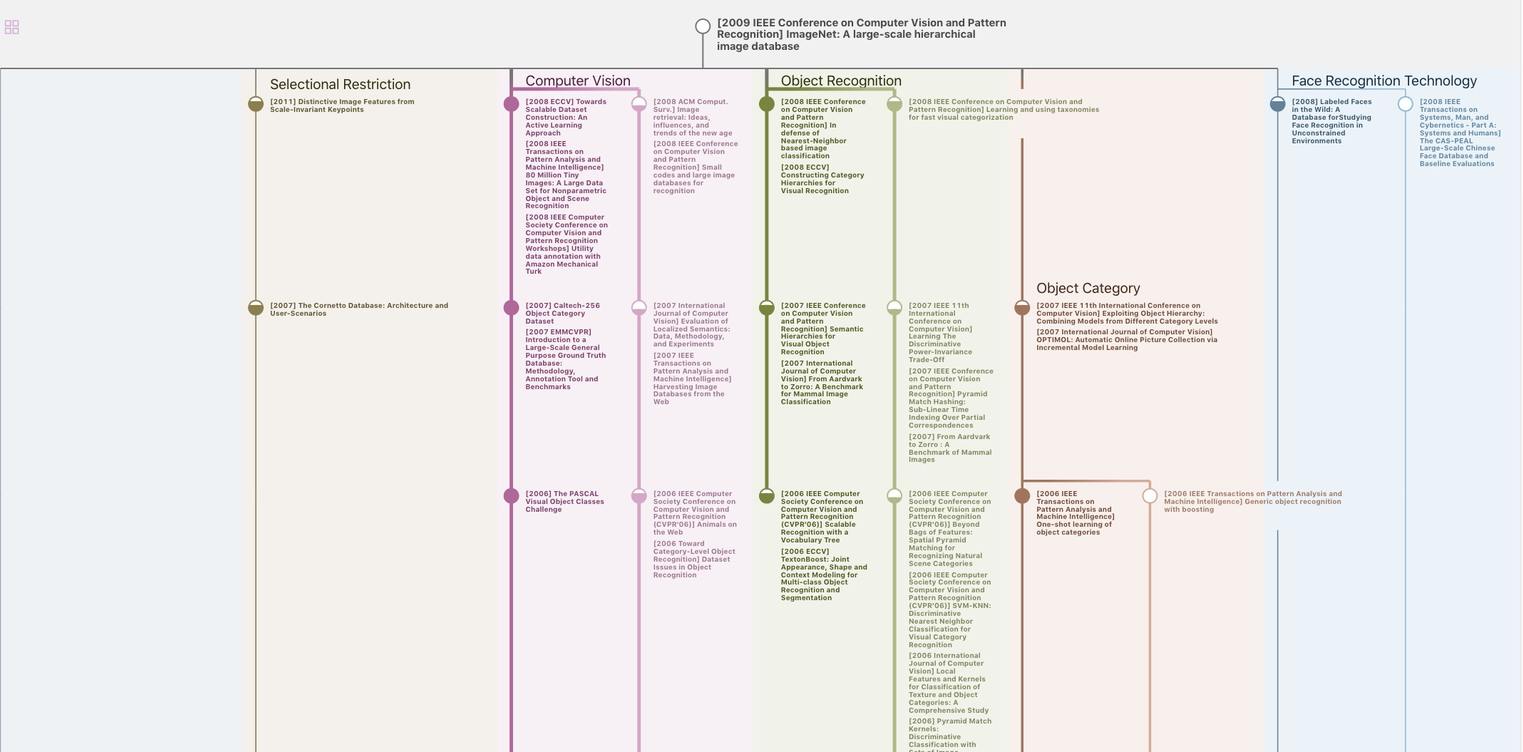
生成溯源树,研究论文发展脉络
Chat Paper
正在生成论文摘要