Assessing severity of road cracks using deep learning-based segmentation and detection
JOURNAL OF SUPERCOMPUTING(2022)
摘要
For the successful operation of pavement management system, it is necessary to automate the detection, classification, and severity assessment of road cracks, which are bottlenecks in the entire process. Although good results for the detection and classification of road cracks have been published in many related studies, the number of crack types detected is still insufficient for actual field use, and studies on crack severity assessment are difficult to find. In this study, the number of crack types are expanded to five types (alligator crack, longitudinal crack, transverse crack, pothole, and patching) to meet the needs of fieldwork, and the assessment of crack severity is also included in the proposed model. In this system constructed using SqueezeNet, U-Net, and Mobilenet-SSD models together, an accuracy of 91.2% has been achieved for both crack type and severity assessment. The authors have performed segmentation of the input images using separately trained U-Nets for linear cracking and area cracking to improve object detection performance and automate crack severity assessment. With reference to the system presented in this study, it is expected that an automated pavement management system that better reflects each country’s requirements for various crack types and severity standards is possible.
更多查看译文
关键词
Crack segmentation,Crack severity,Object detection,Pavement management,Road crack detection
AI 理解论文
溯源树
样例
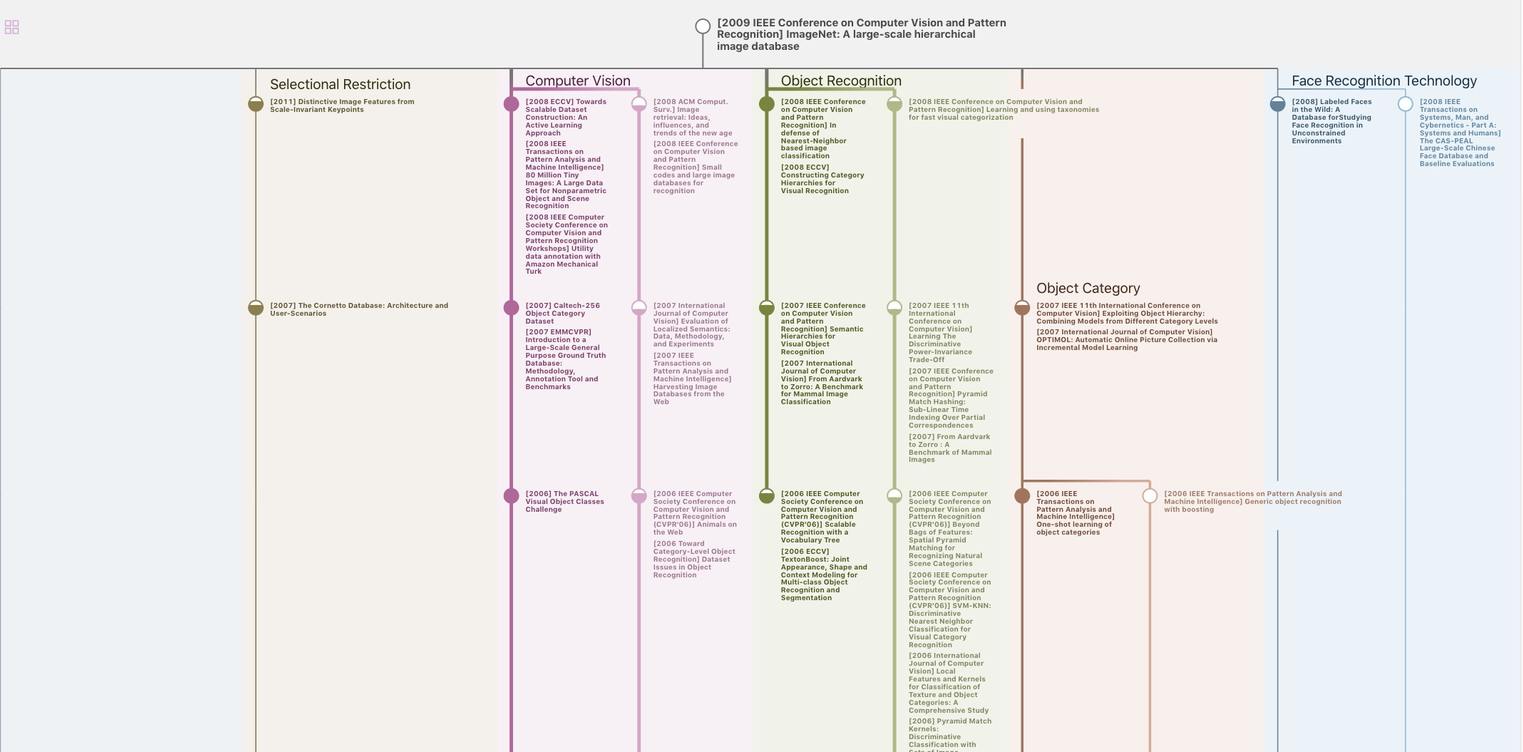
生成溯源树,研究论文发展脉络
Chat Paper
正在生成论文摘要