Fault Diagnosis Based on Deep Residual Shrinkage Network and Maximum Mean Discrepancy
2021 China Automation Congress (CAC)(2021)
摘要
The working environment of rolling bearings is complex, and it is often influenced by noise When the condition changes, a large number of samples with known fault types cannot be provided in the previous condition. These two reasons lead to the decrease of the accuracy of fault diagnosis on testing samples. This paper proposes a transfer learning way based on deep residual shrinkage network and maximum mean discrepancy (DRSN-MMD), which can be used in bearing fault diagnosis under complex and changing conditions. Deep residual shrinkage network (DRSN) has the capacity to eliminate redundant information from vibration signals because soft thresholding is inserted. As a classical criterion to measure the distance between source and target domain, maximum mean discrepancy (MMD) can be used in transfer learning. In our paper, DRSN is used to extract fault feature, and MMD is applied as loss function on model training. In contrast with other methods, the proposed DRSN-MMD approach has higher diagnosis accuracy on the well-known Case Western Reserve University bearing dataset.
更多查看译文
关键词
fault diagnosis,deep residual shrinkage network,feature extraction,transfer learning
AI 理解论文
溯源树
样例
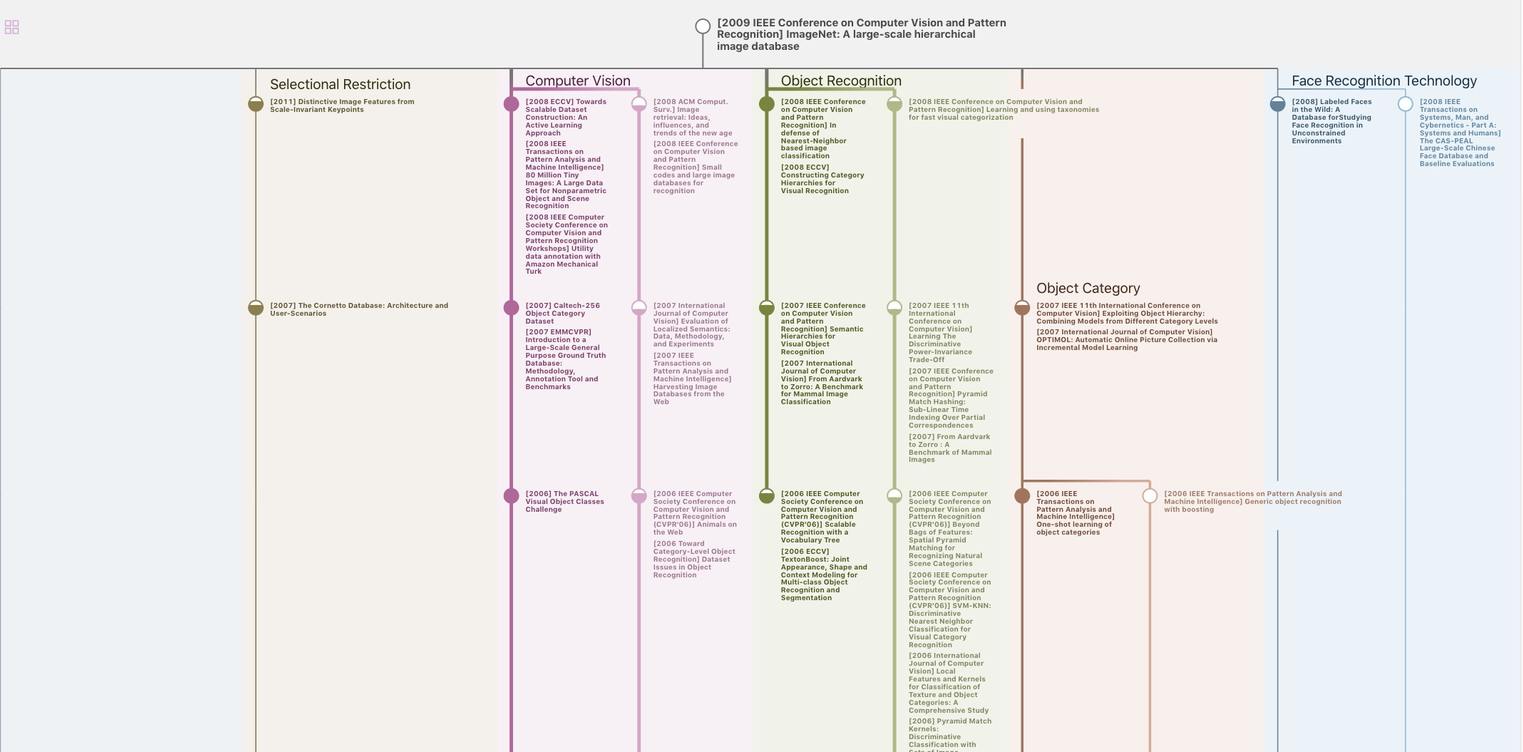
生成溯源树,研究论文发展脉络
Chat Paper
正在生成论文摘要