Active Learning with Safety Constraints
NeurIPS 2022(2022)
摘要
Active learning methods have shown great promise in reducing the number of samples necessary for learning. As automated learning systems are adopted into real-time, real-world decision-making pipelines, it is increasingly important that such algorithms are designed with safety in mind. In this work we investigate the complexity of learning the best safe decision in interactive environments. We reduce this problem to a constrained linear bandits problem, where our goal is to find the best arm satisfying certain (unknown) safety constraints. We propose an adaptive experimental design-based algorithm, which we show efficiently trades off between the difficulty of showing an arm is unsafe vs suboptimal. To our knowledge, our results are the first on best-arm identification in linear bandits with safety constraints. In practice, we demonstrate that this approach performs well on synthetic and real world datasets.
更多查看译文
关键词
bandits,linear bandits,active classification,active learning
AI 理解论文
溯源树
样例
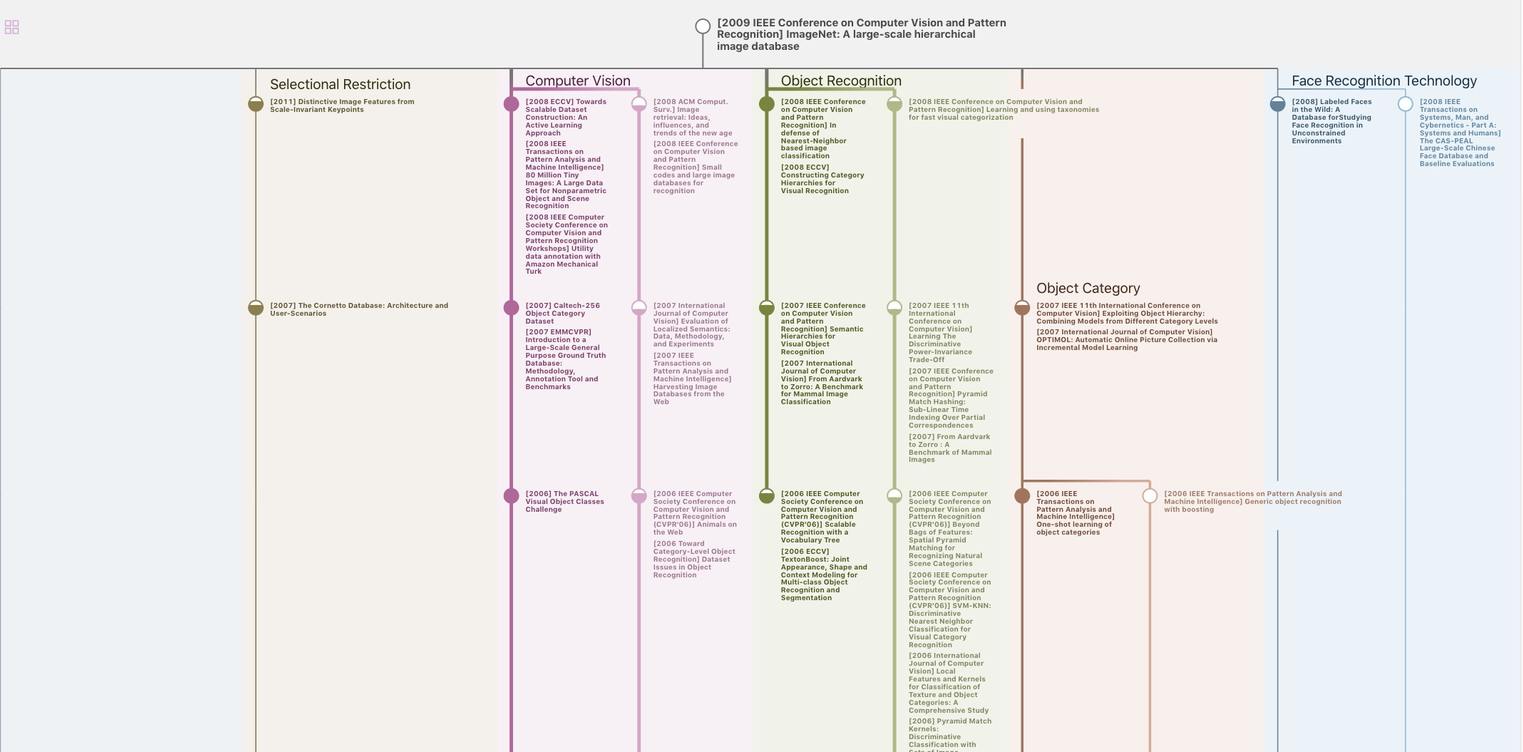
生成溯源树,研究论文发展脉络
Chat Paper
正在生成论文摘要