S2RL: Do We Really Need to Perceive All States in Deep Multi-Agent Reinforcement Learning?
KDD '22: Proceedings of the 28th ACM SIGKDD Conference on Knowledge Discovery and Data Mining(2022)
摘要
Collaborative multi-agent reinforcement learning (MARL) has been widely used in many practical applications, where each agent makes a decision based on its own observation. Most mainstream methods treat each local observation as an entirety when modeling the decentralized local utility functions. However, they ignore the fact that local observation information can be further divided into several entities, and only part of the entities is helpful to model inference. Moreover, the importance of different entities may change over time. To improve the performance of decentralized policies, the attention mechanism is used to capture features of local information. Nevertheless, existing attention models rely on dense fully connected graphs and cannot better perceive important states. To this end, we propose a sparse state based MARL (S2RL) framework, which utilizes a sparse attention mechanism to discard irrelevant information in local observations. The local utility functions are estimated through the self-attention and sparse attention mechanisms separately, then are combined into a standard joint value function and auxiliary joint value function in the central critic. We design the S2RL framework as a plug-and-play module, making it general enough to be applied to various methods. Extensive experiments on StarCraft II show that S2RL can significantly improve the performance of many state-of-the-art methods.
更多查看译文
关键词
reinforcement learning,deep,states,multi-agent
AI 理解论文
溯源树
样例
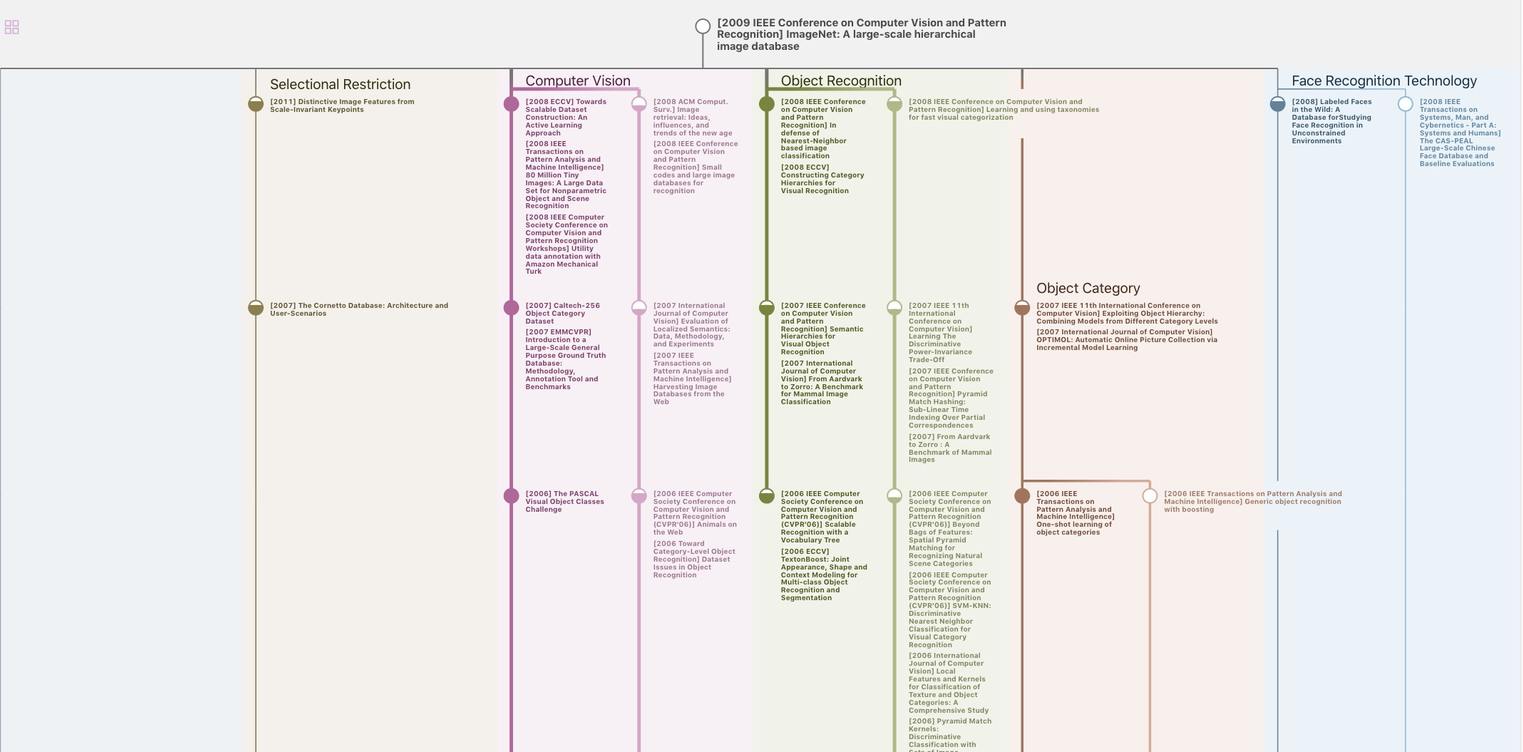
生成溯源树,研究论文发展脉络
Chat Paper
正在生成论文摘要