FairGrad: Fairness Aware Gradient Descent
ICLR 2023(2022)
摘要
We tackle the problem of group fairness in classification, where the objective is to learn models that do not unjustly discriminate against subgroups of the population. Most existing approaches are limited to simple binary tasks or involve difficult to implement training mechanisms. This reduces their practical applicability. In this paper, we propose FairGrad, a method to enforce fairness based on a reweighting scheme that iteratively learns group specific weights based on whether they are advantaged or not. FairGrad is easy to implement and can accommodate various standard fairness definitions. Furthermore, we show that it is comparable to standard baselines over various datasets including ones used in natural language processing and computer vision.
更多查看译文
关键词
Group Fairness,Optimization
AI 理解论文
溯源树
样例
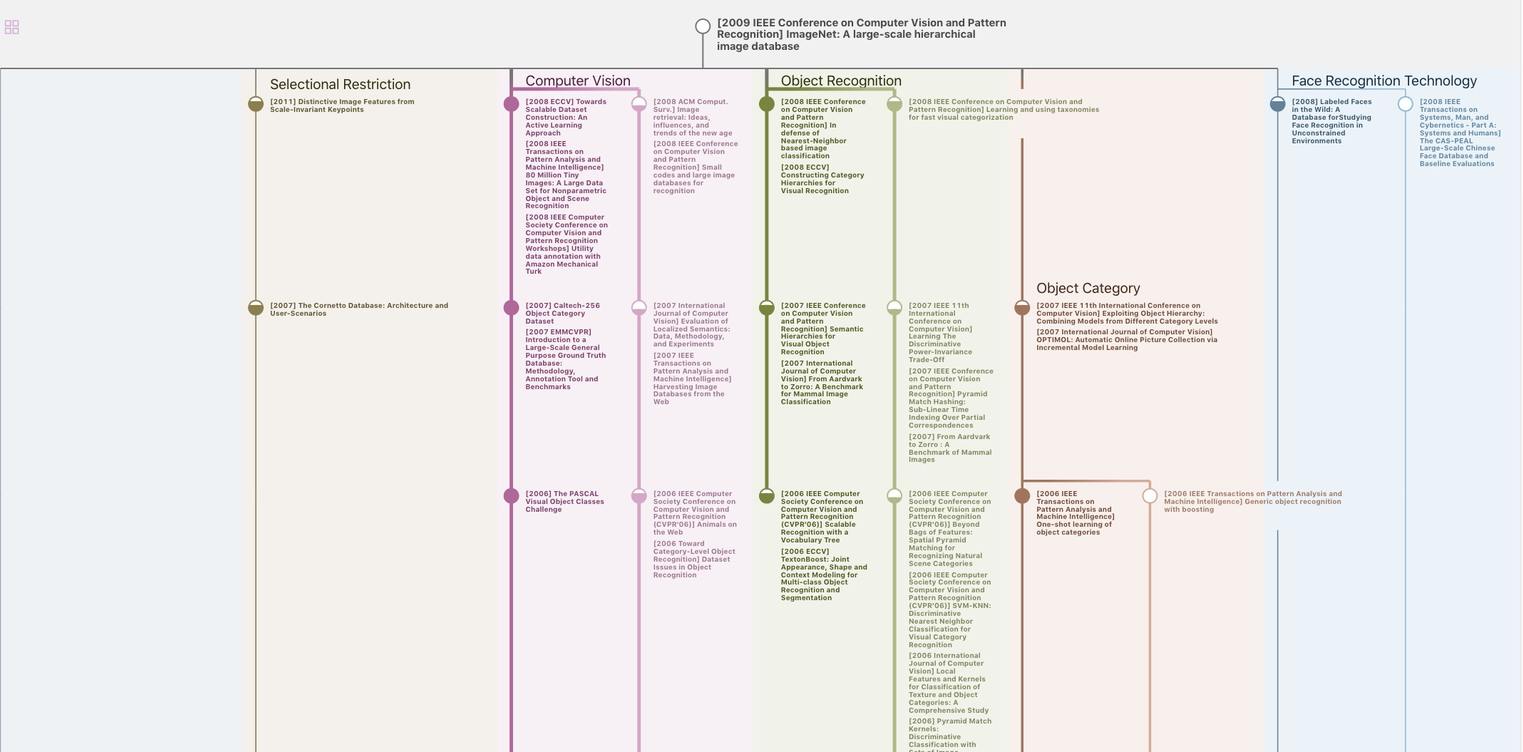
生成溯源树,研究论文发展脉络
Chat Paper
正在生成论文摘要