How to Combine Variational Bayesian Networks in Federated Learning
arxiv(2022)
摘要
Federated Learning enables multiple data centers to train a central model collaboratively without exposing any confidential data. Even though deterministic models are capable of performing high prediction accuracy, their lack of calibration and capability to quantify uncertainty is problematic for safety-critical applications. Different from deterministic models, probabilistic models such as Bayesian neural networks are relatively well-calibrated and able to quantify uncertainty alongside their competitive prediction accuracy. Both of the approaches appear in the federated learning framework; however, the aggregation scheme of deterministic models cannot be directly applied to probabilistic models since weights correspond to distributions instead of point estimates. In this work, we study the effects of various aggregation schemes for variational Bayesian neural networks. With empirical results on three image classification datasets, we observe that the degree of spread for an aggregated distribution is a significant factor in the learning process. Hence, we present an investigation on the question of how to combine variational Bayesian networks in federated learning, while providing benchmarks for different aggregation settings.
更多查看译文
关键词
variational bayesian networks,federated learning
AI 理解论文
溯源树
样例
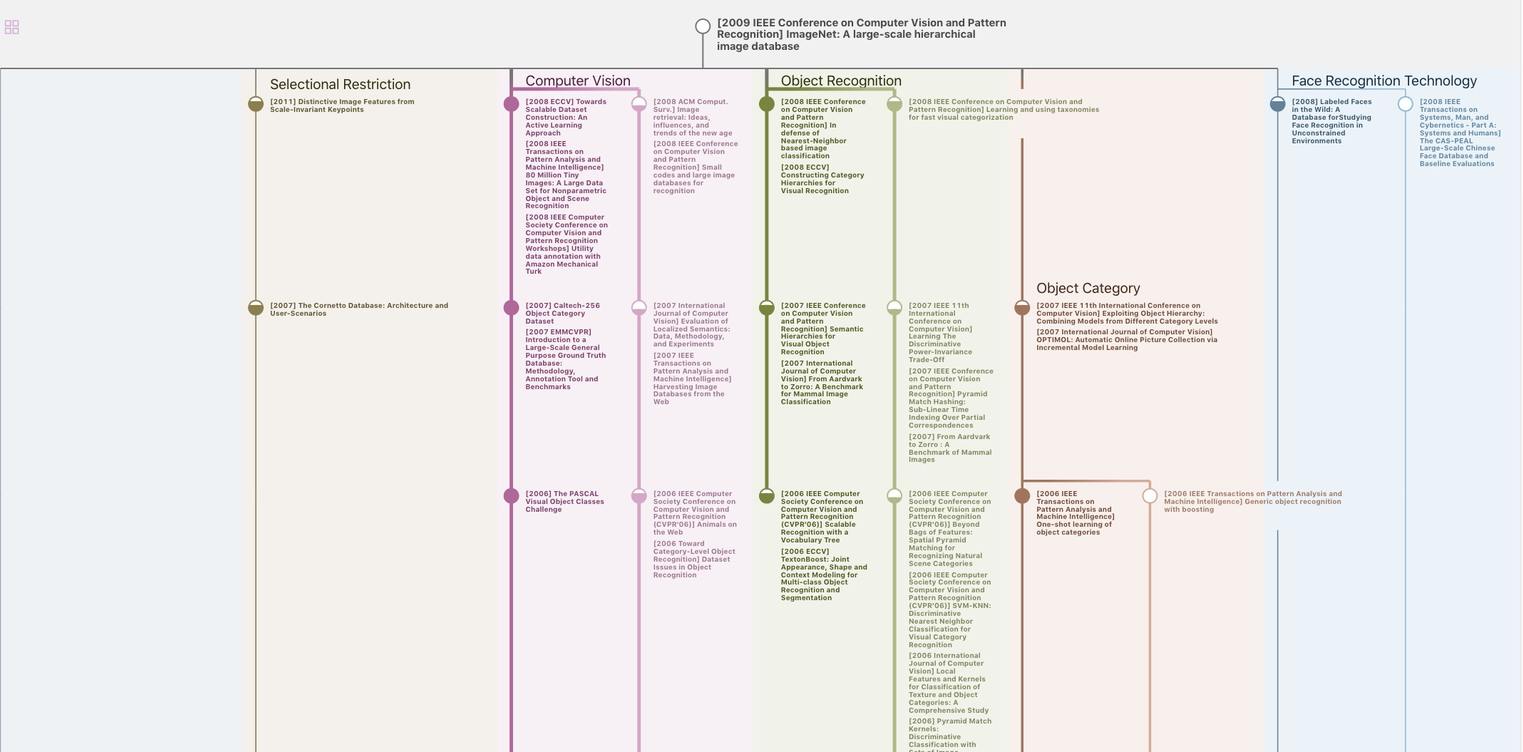
生成溯源树,研究论文发展脉络
Chat Paper
正在生成论文摘要